Figures 4 and 5 show data dispersion in form of a plot. Moreover, there are approximately 1200 total posts where 200 are depressed and others are not depressed. Here, 0 shows negative and 1 shows positive.
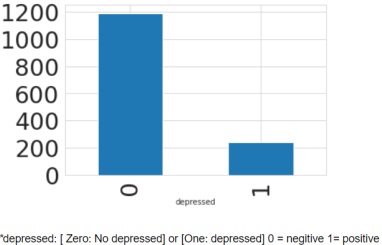
Figure 6: Positive and Negative depression
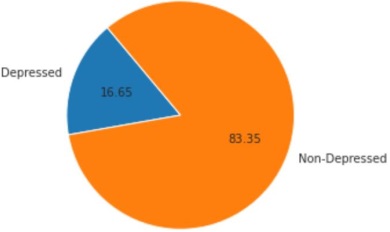
Figure 7: Circle diagram how much depression or non-depress
As per the circular diagram, 83.35% were non-depress and 16.65% were depressed. In next various other attributes were also considered including Gender, Age, No of Children, educational level, category, and income of each participant.
Gender plays a significant role, as studies have indicated differing prevalence rates between men and women, with societal expectations and biological factors influencing susceptibility. Age is another crucial factor, as the manifestation and severity of depression can vary across different life stages. The number of children in a participant's life can also impact their mental well-being, with parenting responsibilities and family dynamics influencing stress levels. Educational level is linked to mental health, with higher education often associated with better-coping mechanisms. Additionally, socioeconomic factors such as category and income contribute to depression, as individuals facing economic challenges may experience heightened stressors. Therefore, a holistic understanding of depression necessitates an exploration of these multifaceted parameters to develop targeted interventions and support systems for individuals experiencing depressive symptoms.
The choice between Naive Bayes and Support Vector Machines (SVM) for estimating depression based on comments from social media platforms depends on several factors, including the characteristics of your data, the size of your dataset, and the specific requirements of your task. Both algorithms have their strengths and weaknesses.
Naive Bayes is a simple and computationally efficient algorithm, making it well-suited for text classification tasks. It assumes independence between features, which may not hold true in the case of natural language, but it often performs surprisingly well in practice. Naive Bayes is particularly useful when dealing with large datasets and can handle high-dimensional feature spaces efficiently.
On the other hand, Support Vector Machines are powerful classifiers that excel in finding complex patterns and nonlinear relationships in data. SVM can capture intricate relationships between words and expressions in text, making it suitable for tasks where feature interactions are crucial. However, SVMs can be computationally intensive, especially with large datasets.
For estimating depression from social media comments, where the language used can be complex and nuanced, and the dataset might be large, SVM might be a good choice due to its ability to capture intricate patterns. However, it's recommended to experiment with both algorithms on your specific dataset and evaluate their performance using metrics like accuracy, precision, recall, and F1-score to determine which one works better for your particular application. Additionally, other advanced techniques like deep learning models, such as recurrent neural networks (RNNs) or transformers, could also be explored for their effectiveness in capturing context and nuances in textual data.
In succeeding parts of Figure 8, light colors show the maximum level and dark colors show the minimum level.
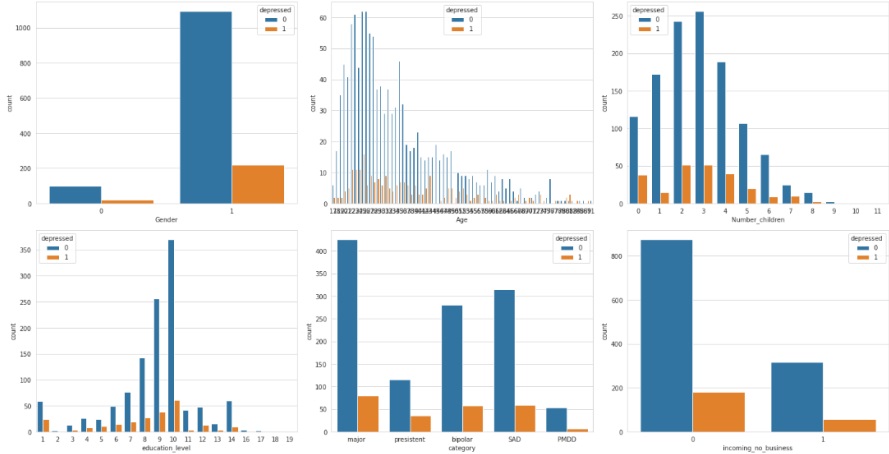
Figure 8: Graphical results of depression on the base of gender, age and different personals attributes
Discussion:
The investigation of depression by social media data mining is an example of how technology, mental health, and ethical issues are dynamically intersecting. The process of gathering data and applying machine learning models has revealed new information and shown the possibility of classifying and comprehending depression in the digital sphere.
Interpretation of Results:
The sentiment and keyword analyzed to train the machine learning models provide promising results in differentiating between social media posts that are symptomatic of depression and those that are not. The precision of the categorization process and dependability highlight data mining potential as a useful tool for mental health research. But it's important to recognize the inherent difficulties in interpreting findings when dealing with subjective, complex diseases like depression.
Ethical Considerations and User Privacy:
When analyzing mental health discussions on social media, ethical considerations remain paramount. Striking the correct balance between acquiring valuable insights and safeguarding user privacy demands meticulous attention. Future studies should explore more reliable anonymization techniques, and ethical standards for responsible data utilization in digitally conducted mental health research need to be considered.
Potential for Intervention and Support:
While this research has primarily focused on classification and understanding, it also aims to offer responsible interventions. Identifying individuals at risk of depression provides an opportunity for timely and targeted treatments, ranging from the allocation of mental health resources to the establishment of virtual support groups. However, the ethical implications of these interventions must be carefully considered, underscoring the importance of user consent and respect for individual preferences.
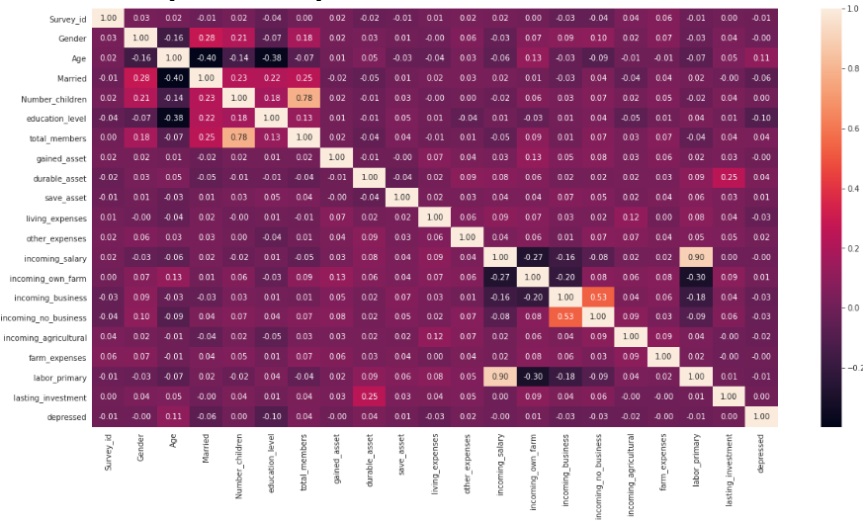
Figure 9: Results in the form of a Heat Map diagram
Conclusion
In conclusion, the synergistic integration of Digital Twin technology, alongside sophisticated data mining and analysis techniques, holds immense promise for advancing the categorization of depression based on social media interactions. By creating a virtual representation of individuals and their online behaviors, Digital Twin technology offers a dynamic and real-time framework for capturing the evolving nature of mental health expressions. Coupled with powerful data mining algorithms, such as Support Vector Machines and Naive Bayes, this approach enables a nuanced understanding of complex linguistic patterns and contextual nuances within social media content. The fusion of these technologies not only facilitates more accurate and timely depression categorization but also opens avenues for personalized interventions and targeted mental health support. As we continue to navigate the evolving landscape of digital interactions, the synergistic application of Digital Twin technology and data mining analysis emerges as a transformative strategy with the potential to enhance our comprehension and response to mental health challenges in the digital era.
Limitations and Future Directions:
The models generated may not entirely capture the spectrum of depressive experiences, given their reliance on readily available public data. Future research should explore methods to integrate diverse data sources and consider the nuanced differences in language and expression across various cultures. Additionally, due to the dynamic nature of social media platforms, regular updates to the models are essential to ensure their continued effectiveness in identifying emerging language patterns.
Acknowledgment:
I would like to express my sincere gratitude to Mr. Muhammad Dawood Khan for his technical assistance in conducting this research. Their expertise and insights significantly enriched the quality of this work.
Reference
[1] M.-Y. Fan et al., “Acupoints compatibility rules of acupuncture for depression disease based on data mining technology,” Zhongguo Zhen Jiu, vol. 43, no. 3, pp. 269–76, 2023, doi: 10.13703/J.0255-2930.20221103-K0001.
[2] D. Xue, Y. Zhang, Z. Song, X. Jie, R. Jia, and A. Zhu, “Integrated meta-analysis, data mining, and animal experiments to investigate the efficacy and potential pharmacological mechanism of a TCM tonic prescription, Jianpi Tongmai formula, in depression,” Phytomedicine, vol. 105, p. 154344, Oct. 2022, doi: 10.1016/J.PHYMED.2022.154344.
[3] “Meme Detection of Journalists from Social Media by Using Data Mining Techniques | International Journal of Innovations in Science & Technology.” Accessed: Nov. 26, 2023. [Online]. Available: https://journal.50sea.com/index.php/IJIST/article/view/404
[4] F. Alhussain, A. Bin Onayq, D. Ismail, M. Alduayj, T. Alawbathani, and M. Aljaffer, “Adjustment disorder among first year medical students at King Saud University, Riyadh, Saudi Arabia, in 2020,” J. Fam. Community Med., vol. 30, no. 1, p. 59, 2023, doi: 10.4103/JFCM.JFCM_227_22.
[5] Ö. Baltacı, “The Predictive Relationships between the Social Media Addiction and Social Anxiety, Loneliness, and Happiness,” Int. J. Progress. Educ., vol. 15, no. 4, pp. 73–82, Aug. 2019, doi: 10.29329/IJPE.2019.203.6.
[6] W. Lu et al., “Differences in cognitive functions of atypical and non-atypical depression based on propensity score matching,” J. Affect. Disord., vol. 325, pp. 732–738, Mar. 2023, doi: 10.1016/J.JAD.2023.01.071.
[7] M. P. Valerio, J. Lomastro, A. Igoa, and D. J. Martino, “Clinical Characteristics of Melancholic and Nonmelancholic Depressions,” J. Nerv. Ment. Dis., vol. 211, no. 3, pp. 248–252, Mar. 2023, doi: 10.1097/NMD.0000000000001616.
[8] F. Tasnim, S. U. Habiba, N. Nafisa, and A. Ahmed, “Depressive Bangla Text Detection from Social Media Post Using Different Data Mining Techniques,” Lect. Notes Electr. Eng., vol. 834, pp. 237–247, 2022, doi: 10.1007/978-981-16-8484-5_21/COVER.
[9] M. Maes and A. F. Almulla, “Research and Diagnostic Algorithmic Rules (RADAR) and RADAR Plots for the First Episode of Major Depressive Disorder: Effects of Childhood and Recent Adverse Experiences on Suicidal Behaviors, Neurocognition and Phenome Features,” Brain Sci. 2023, Vol. 13, Page 714, vol. 13, no. 5, p. 714, Apr. 2023, doi: 10.3390/BRAINSCI13050714.
[10] C. S. Wu, C. J. Kuo, C. H. Su, S. H. Wang, and H. J. Dai, “Using text mining to extract depressive symptoms and to validate the diagnosis of major depressive disorder from electronic health records,” J. Affect. Disord., vol. 260, pp. 617–623, Jan. 2020, doi: 10.1016/J.JAD.2019.09.044.
[11] R. Vanlalawmpuia and M. Lalhmingliana, “Prediction of Depression in Social Network Sites Using Data Mining,” Proc. Int. Conf. Intell. Comput. Control Syst. ICICCS 2020, pp. 489–495, May 2020, doi: 10.1109/ICICCS48265.2020.9120899.
[12] C. K. Ettman, S. M. Abdalla, G. H. Cohen, L. Sampson, P. M. Vivier, and S. Galea, “Prevalence of Depression Symptoms in US Adults Before and During the COVID-19 Pandemic,” JAMA Netw. Open, vol. 3, no. 9, pp. e2019686–e2019686, Sep. 2020, doi: 10.1001/JAMANETWORKOPEN.2020.19686.
[13] L. Smith et al., “Association between depression and subjective cognitive complaints in 47 low- and middle-income countries,” J. Psychiatr. Res., vol. 154, pp. 28–34, Oct. 2022, doi: 10.1016/J.JPSYCHIRES.2022.07.021.
[14] S. L. Dubovsky, B. M. Ghosh, J. C. Serotte, and V. Cranwell, “Psychotic Depression: Diagnosis, Differential Diagnosis, and Treatment,” Psychother. Psychosom., vol. 90, no. 3, pp. 160–177, Apr. 2021, doi: 10.1159/000511348.
[15] Ö. DEMİRCİ and E. INAN, “Postpartum Paternal Depression: Its Impact on Family and Child Development,” Curr. Approaches Psychiatry, vol. 15, no. 3, pp. 498–507, Sep. 2023, doi: 10.18863/PGY.1153712.
[16] L. Sforzini et al., “A Delphi-method-based consensus guideline for definition of treatment-resistant depression for clinical trials,” Mol. Psychiatry 2021 273, vol. 27, no. 3, pp. 1286–1299, Dec. 2021, doi: 10.1038/s41380-021-01381-x.