Bard and ChatGPT are two significant large language models, both trained on a vast amount of data and code. This article compares Google Bard and ChatGPT by analyzing topic-wise comparable documents on oncology generated with these models. During the study, 50 documents were generated by Google Bard, and the same topic-wise documents were generated with ChatGPT. On average, Google Bard generated documents with 394.60 words, of which 100.68 were identified as difficult. In contrast, documents generated with ChatGPT had a mean value of 394.80 words, with 119.40 identified as difficult. The 17.01% difference in difficult words suggests that Google Bard.
An examination of documents related to oncology generated by Google Bard and ChatGPT unveiled a notable degree of similarity. The analysis of cosine similarity yielded a score of 0.66, indicating a robust alignment between the documents. Further exploration using Jaccard similarity resulted in an even higher score of 0.88, signifying a substantial level of resemblance. These findings imply that, despite being developed by distinct research teams, both models were likely trained on analogous datasets or sources of oncology-related information, such as medical literature, research papers, or publicly available data.
The observed similarity can be ascribed to the probabilistic nature of language models, wherein predictions of the next word or sequence are made based on patterns learned during training. Without specific fine-tuning for uniqueness or novelty, these models have a tendency to generate similar content contingent on the provided input and context.
Furthermore, the architecture and design of language models may also play a role in the similarities observed in the generated content. Models sharing akin architectures or training methodologies are predisposed to producing similar outputs. Moreover, overfitting to specific patterns or information during training may contribute to similarities that do not necessarily reflect the broader diversity of oncology-related content. In its entirety, the study reached the conclusion that, with regard to the analyzed pages on oncology, Google Bard and ChatGPT exhibit a notable degree of similarity in terms of document generation.
Google Bard and ChatGPT have been analyzed from distinct perspectives in this study, representing a novel approach as it quantitatively analyzes and compares documents, an aspect that has not been studied before. The findings indicate that, despite being distinct language models, the content they generate on oncology exhibits noteworthy similarities. This observed similarity could be ascribed to the probabilistic nature of language models and the potential for overfitting during their training processes. Consequently, these results implicitly validate the originality and novelty of this work.
However, this work is subject to several limitations and threats to validity: i) The study is based on a very small sample of analyzed documents, ii) Only two methods were employed to assess the similarity of documents, iii) The comparison of the two large language models focuses exclusively on oncology documents, iv) The reliance on similarity scores may naturally overlook qualitative differences in generated content, such as variations in writing style and depth of analysis, and v) The suggestion of potential overfitting lacks concrete evidence or a detailed analysis of model training dynamics, introducing uncertainty to the claim and necessitating further investigation. As a part of further study, a more detailed analysis will be performed by considering a large sample of documents on diverse topics with more methods for text similarity.
[1] P. Hamet, and J. Trembla, “Artificial Intelligence in Medicine | Journal | ScienceDirect.com by Elsevier.” Accessed: Dec. 16, 2023. [Online]. Available: https://www.sciencedirect.com/journal/artificial-intelligence-in-medicine
[2] R. E. Lopez-Martinez and G. Sierra, “Research trends in the international literature on natural language processing, 2000-2019 - A bibliometric study,” J. Scientometr. Res., vol. 9, no. 3, pp. 310–318, Sep. 2020, doi: 10.5530/JSCIRES.9.3.38.
[3] Y. Shen et al., “ChatGPT and Other Large Language Models Are Double-edged Swords,” Radiology, vol. 307, no. 2, p. 2023, Apr. 2023, doi: 10.1148/RADIOL.230163/ASSET/IMAGES/LARGE/RADIOL.230163.FIG1.JPEG.
[4] M. C. Rillig, M. Ågerstrand, M. Bi, K. A. Gould, and U. Sauerland, “Risks and Benefits of Large Language Models for the Environment,” Environ. Sci. Technol., vol. 57, no. 9, pp. 3464–3466, Mar. 2023, doi: 10.1021/ACS.EST.3C01106/ASSET/IMAGES/LARGE/ES3C01106_0004.JPEG.
[5] A. Bryant et al., “Qualitative Research Methods for Large Language Models: Conducting Semi-Structured Interviews with ChatGPT and BARD on Computer Science Education,” Informatics 2023, Vol. 10, Page 78, vol. 10, no. 4, p. 78, Oct. 2023, doi: 10.3390/INFORMATICS10040078.
[6] A. Egli and A. Egli, “ChatGPT, GPT-4, and Other Large Language Models: The Next Revolution for Clinical Microbiology?,” Clin. Infect. Dis., vol. 77, no. 9, pp. 1322–1328, Nov. 2023, doi: 10.1093/CID/CIAD407.
[7] C. Tan Yip Ming et al., “The Potential Role of Large Language Models in Uveitis Care: Perspectives After ChatGPT and Bard Launch,” Ocul. Immunol. Inflamm., Aug. 2023, doi: 10.1080/09273948.2023.2242462.
[8] S. Thapa and S. Adhikari, “ChatGPT, Bard, and Large Language Models for Biomedical Research: Opportunities and Pitfalls,” Ann. Biomed. Eng., vol. 51, no. 12, pp. 2647–2651, Dec. 2023, doi: 10.1007/S10439-023-03284-0/METRICS.
[9] T. B. Brown et al., “Language Models are Few-Shot Learners,” Adv. Neural Inf. Process. Syst., vol. 2020-December, May 2020, Accessed: Dec. 16, 2023. [Online]. Available: https://arxiv.org/abs/2005.14165v4
[10] Ö. AYDIN, “Google Bard Generated Literature Review: Metaverse,” J. AI, vol. 7, no. 1, pp. 1–14, Dec. 2023, doi: 10.61969/JAI.1311271.
[11] R. C. T. Cheong et al., “Artificial intelligence chatbots as sources of patient education material for obstructive sleep apnoea: ChatGPT versus Google Bard,” Eur. Arch. Oto-Rhino-Laryngology, pp. 1–9, Nov. 2023, doi: 10.1007/S00405-023-08319-9/METRICS.
[12] N. Fijačko, G. Prosen, B. S. Abella, S. Metličar, and G. Štiglic, “Can novel multimodal chatbots such as Bing Chat Enterprise, ChatGPT-4 Pro, and Google Bard correctly interpret electrocardiogram images?,” Resuscitation, vol. 193, p. 110009, Dec. 2023, doi: 10.1016/j.resuscitation.2023.110009.
[13] N. S. Patil, R. S. Huang, C. B. van der Pol, and N. Larocque, “Comparative Performance of ChatGPT and Bard in a Text-Based Radiology Knowledge Assessment,” Can. Assoc. Radiol. J., Aug. 2023, doi: 10.1177/08465371231193716/ASSET/IMAGES/LARGE/10.1177_08465371231193716-FIG1.JPEG.
[14] F. Y. Al-Ashwal, M. Zawiah, L. Gharaibeh, R. Abu-Farha, and A. N. Bitar, “Evaluating the Sensitivity, Specificity, and Accuracy of ChatGPT-3.5, ChatGPT-4, Bing AI, and Bard Against Conventional Drug-Drug Interactions Clinical Tools,” Drug. Healthc. Patient Saf., vol. 15, pp. 137–147, Sep. 2023, doi: 10.2147/DHPS.S425858.
[15] R. K. Gan, J. C. Ogbodo, Y. Z. Wee, A. Z. Gan, and P. A. González, “Performance of Google bard and ChatGPT in mass casualty incidents triage,” Am. J. Emerg. Med., vol. 75, pp. 72–78, Jan. 2024, doi: 10.1016/J.AJEM.2023.10.034.
[16] R. Ali et al., “Performance of ChatGPT, GPT-4, and Google Bard on a Neurosurgery Oral Boards Preparation Question Bank,” Neurosurgery, vol. 93, no. 5, pp. 1090–1098, Nov. 2023, doi: 10.1227/NEU.0000000000002551.
[17] A. K. D. Dhanvijay et al., “Performance of Large Language Models (ChatGPT, Bing Search, and Google Bard) in Solving Case Vignettes in Physiology,” Cureus, vol. 15, no. 8, Aug. 2023, doi: 10.7759/CUREUS.42972.
[18] D. E. O and C. E. Daniel O, “An analysis of Watson vs. BARD vs. ChatGPT: The Jeopardy! Challenge,” AI Mag., vol. 44, no. 3, pp. 282–295, Sep. 2023, doi: 10.1002/AAAI.12118.
[19] V. Plevris, G. Papazafeiropoulos, and A. Jiménez Rios, “Chatbots Put to the Test in Math and Logic Problems: A Comparison and Assessment of ChatGPT-3.5, ChatGPT-4, and Google Bard,” AI, vol. 4, no. 4, pp. 949–969, Oct. 2023, doi: 10.3390/ai4040048.
[20] S. Koga, N. B. Martin, and D. W. Dickson, “Evaluating the performance of large language models: ChatGPT and Google Bard in generating differential diagnoses in clinicopathological conferences of neurodegenerative disorders,” Brain Pathol., p. e13207, 2023, doi: 10.1111/BPA.13207.
[21] I. Seth et al., “Comparing the Efficacy of Large Language Models ChatGPT, BARD, and Bing AI in Providing Information on Rhinoplasty: An Observational Study,” Aesthetic Surg. J. Open Forum, vol. 5, Jan. 2023, doi: 10.1093/ASJOF/OJAD084.
[22] “Natural Language Processing with Python and spaCy: A Practical Introduction - Yuli Vasiliev - Google Books.” Accessed: Dec. 16, 2023. [Online]. Available: https://books.google.com.pk/books?id=w_ZqywEACAAJ&printsec=copyright&redir_esc=y#v=onepage&q&f=false
[23] R. Spring and M. Johnson, “The possibility of improving automated calculation of measures of lexical richness for EFL writing: A comparison of the LCA, NLTK and SpaCy tools,” System, vol. 106, p. 102770, Jun. 2022, doi: 10.1016/J.SYSTEM.2022.102770.
[24] R. Verma and A. Mittal, “Multiple attribute group decision-making based on novel probabilistic ordered weighted cosine similarity operators with Pythagorean fuzzy information,” Granul. Comput., vol. 8, no. 1, pp. 111–129, Jan. 2023, doi: 10.1007/S41066-022-00318-1/METRICS.
[25] R. Zhang, Z. Xu, and X. Gou, “ELECTRE II method based on the cosine similarity to evaluate the performance of financial logistics enterprises under double hierarchy hesitant fuzzy linguistic environment,” Fuzzy Optim. Decis. Mak., vol. 22, no. 1, pp. 23–49, Mar. 2023, doi: 10.1007/S10700-022-09382-3/METRICS.
[26] D. Dede Şener, H. Ogul, and S. Basak, “Text-based experiment retrieval in genomic databases,” J. Inf. Sci., Sep. 2022, doi: 10.1177/01655515221118670/ASSET/IMAGES/LARGE/10.1177_01655515221118670-FIG4.JPEG.
[27] R. M. Suleman and I. Korkontzelos, “Extending latent semantic analysis to manage its syntactic blindness,” Expert Syst. Appl., vol. 165, p. 114130, Mar. 2021, doi: 10.1016/J.ESWA.2020.114130.
[28] Y. Chen, S. Nan, Q. Tian, H. Cai, H. Duan, and X. Lu, “Automatic RadLex coding of Chinese structured radiology reports based on text similarity ensemble,” BMC Med. Inform. Decis. Mak., vol. 21, no. 9, pp. 1–11, Nov. 2021, doi: 10.1186/S12911-021-01604-9/TABLES/3.
[29] T. Bin Sarwar, N. M. Noor, and M. S. U. Miah, “Evaluating keyphrase extraction algorithms for finding similar news articles using lexical similarity calculation and semantic relatedness measurement by word embedding,” PeerJ Comput. Sci., vol. 8, p. e1024, Jul. 2022, doi: 10.7717/PEERJ-CS.1024/SUPP-1.
[30] D. Vogler, L. Udris, and M. Eisenegger, “Measuring Media Content Concentration at a Large Scale Using Automated Text Comparisons,” Journal. Stud., vol. 21, no. 11, pp. 1459–1478, Aug. 2020, doi: 10.1080/1461670X.2020.1761865.
Appendix A. Selected Topics on Oncology
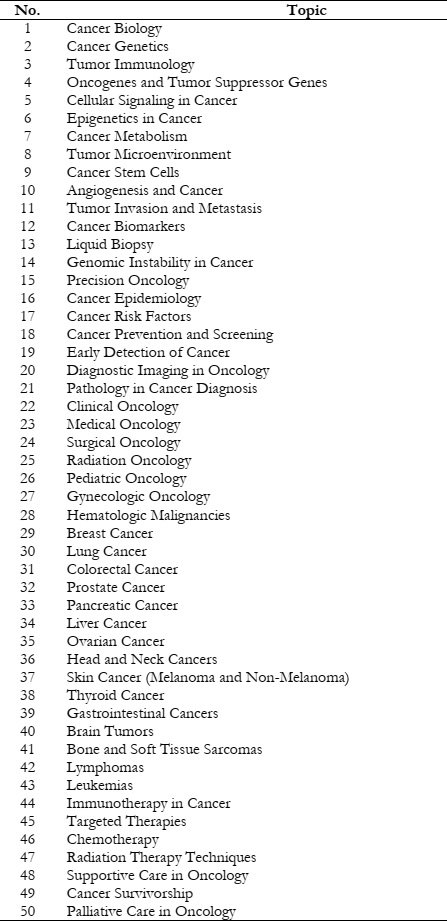