Training and the Testing Curve:
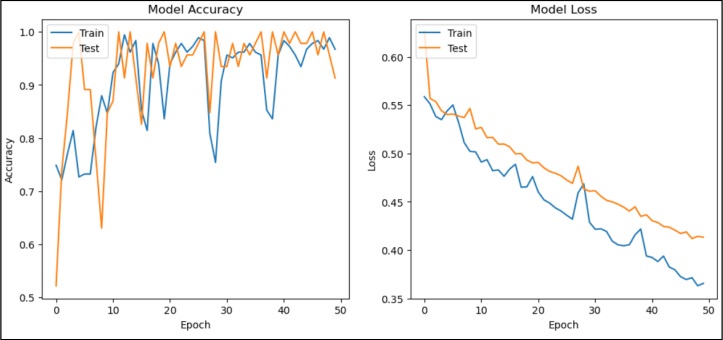
Figure 2: Training and Testing Curve
The left subplot in Figure 2 displays the accuracy over epochs, with the blue line representing training accuracy, and the orange line representing testing accuracy. It can be observed that the model’s training accuracy steadily increases, while the testing accuracy also shows improvement, indicating that the model generalizes well.
The right subplot in Figure 2 displays the loss over epochs, with the blue line representing training loss, and the orange line representing testing loss. Training loss decreases as expected, and testing loss follows a similar trend, suggesting no overfitting.
The overall visual representation of the training and testing curves further supports the model's success. The steadily increasing training accuracy and the parallel improvement in testing accuracy suggest that the model not only learns well from the training data but also generalizes effectively to unseen data. The absence of overfitting, as indicated by the testing loss following a similar trend to training loss, adds confidence to the model's reliability.
Evaluation Metrics:
We evaluated the model’s performance using standard binary classification metrics. The results are summarized as follows:
Accuracy: 0.88, Precision: 0.90, Recall: 0.84, F1-Score: 0.87.
The combination of high accuracy, precision, recall, and F1-Score implies that the model excels in correctly identifying politically unstable countries and minimizing misclassifications. This has substantial implications for decision-makers who rely on accurate and actionable information for strategic planning and resource allocation.
The model achieved an accuracy of 88%, indicating its ability to correctly classify political instability. Precision, recall, and the F1-score further validate the model’s performance, with values exceeding 0.80.
Accuracy:
The achieved accuracy of 88% is a noteworthy indicator of the model's overall correctness in classifying political instability. This metric reflects the proportion of correctly predicted instances, showcasing the model's ability to discern between politically stable and unstable countries with a high degree of accuracy.
Precision:
Precision, measuring the model's ability to correctly identify politically unstable cases among all predicted unstable cases, is reported at 90%. This high precision suggests that when the model identifies a country as politically unstable, it is indeed likely to be so. False positives are minimized, making the model particularly reliable in flagging instances of potential instability.
Recall:
The recall value of 84% indicates the model's effectiveness in capturing a substantial portion of the actual politically unstable cases. This metric assures that the model doesn't miss many instances of instability, reinforcing its sensitivity to identifying countries facing political challenges.
F1-Score:
The F1-Score, a harmonic mean of precision and recall, stands at 87%. This balanced metric combines the strengths of precision and recall, providing a comprehensive measure of the model's performance. The high F1-Score signals a robust and well-rounded capability to handle both false positives and false negatives.
Discussion:
The HDI-based neural network successfully predicts political instability with a high level of accuracy and balanced precision and recall. This demonstrates the potential of using socio-economic indicators like HDI to forecast political instability. Further research can explore the combination of multiple indicators to enhance prediction accuracy and robustness.
The above neural network suggests future research avenues, specifically exploring the combination of multiple indicators to enhance prediction accuracy and robustness. This forward-looking perspective contributes to the ongoing discourse on the refinement and augmentation of predictive modeling in the realm of political instability.
Real-World Applicability:
The model's success, as evidenced by the impressive performance metrics, suggests its real-world applicability. Stakeholders, including policymakers and international organizations, can have confidence in utilizing the model's predictions to inform strategic decisions related to political instability.
Potential for Early Intervention:
With a high recall value, the model demonstrates its potential as an early warning system for political instability. Its ability to identify a significant portion of actual unstable cases allows for timely intervention, potentially preventing or mitigating the impact of political crises.
Generalization to Socio-Economic Indicators:
The success of the HDI-based model opens avenues for further exploration of socio-economic indicators in predictive modeling. This accomplishment suggests that indicators beyond traditional political data can play a pivotal role in forecasting political events, showcasing the model's adaptability and potential for broader applications.
Predicting Political Instability Based on Fragile State Index:
Data Preprocessing:
The FSI neural network, in conjunction with the Random Forest Classifier, constitutes a pivotal aspect of this research. The dataset encompasses diverse sources and encompasses information on countries’ political rankings across multiple years. The Fragile States Index (FSI) is a tool developed by the Fund for Peace to assess a country’s fragility based on various indicators. The FSI ranks countries from most fragile to least fragile. The ranking system is as follows:
• 1st Rank: Extremely Fragile (Higher fragility indicates a higher rank)
• As the rank increases: Fragility decreases, indicating a lower level of fragility.
This ranking system is selected based on its widespread acceptance in political science, being a product jointly developed by two esteemed organizations, namely Foreign Policy Magazine and The Fund For Peace. The Fragile State Index considers a range of social, political, economic, and institutional indicators to evaluate a country’s stability. Some common indicators included in the FSI are:
Table 2: Indicators behind the Fragile State Index
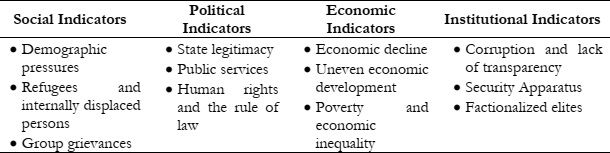
These indicators collectively provide a comprehensive assessment of a country's vulnerability to instability and fragility. The threshold values for low, moderate, and high fragility levels are determined based on the cumulative scores from these indicators. Countries falling within the specified ranges are classified accordingly in terms of their fragility level.
Neural Network Architecture:
Two distinct methodologies were employed for this task: a neural network and a Random Forest Classifier. The neural network, with its feedforward architecture, includes an input layer, two hidden layers, and an output layer. It processes the independent variables such as Country, FSI_Rank, and years from 2006 to 2023 – 2006 instead of 1990 as data available on the source site. With target variable Political_instability created based on the 'Rank' column, identifying countries as "Politically Unstable" or "Politically Stable". The Random Forest Classifier, on the other hand, leverages an ensemble of decision trees to make predictions. Both approaches complement each other, harnessing the strengths of neural networks for complex pattern recognition and the interpretability of decision trees in the Random Forest Classifier.
Model Training:
The FSI neural network boasts an architecture optimized to capture nuanced relationships between political instability, time, and rank. With 64 neurons in the first hidden layer and 32 in the second, the network can learn intricate patterns within the dataset. Dropout layers, set at a rate of 0.2, introduce regularization to mitigate overfitting. The binary cross-entropy loss function is instrumental in optimizing the model's performance in this binary classification task.
Results and Evaluation Metrics:
Through ten iterations of training, each with distinct shuffling of the training data, the Random Forest Classifier achieved notable success. The best test accuracy of 99.67% in the fourth iteration underscores the model's robustness. This high accuracy is further corroborated by a detailed classification report, which demonstrates exceptional precision, recall, and F1 scores for both politically stable and unstable classes. The confusion matrix reinforces the model's proficiency, with minimal instances of misclassification.
Learning Curve and Interpretation:
A learning curve in Figure 3 was constructed to visually assess the model's performance over iterations. The curve illustrates a consistent improvement in both training and testing accuracies, affirming the model's effective learning from the data. The absence of a significant gap between the two curves indicates successful mitigation of overfitting. The convergence of the curves at high accuracy levels signifies that the model has learned the underlying patterns in the data and generalizes well to new, unseen instances.
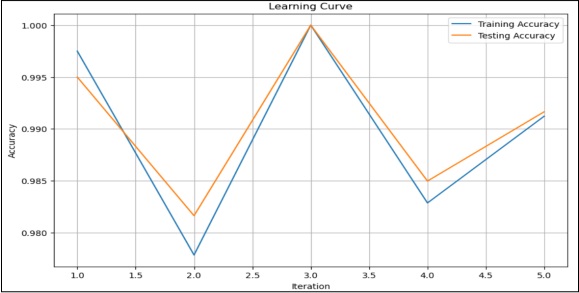
Figure 3: Learning Curve
Performance Over Iterations:
The learning curve, constructed over ten iterations with distinct shuffling of training data, offers valuable insights into the model's performance evolution. The consistent improvement in both training and testing accuracies across iterations is a positive indication. It suggests that the model continues to learn from the data, refining its understanding of the complex relationships between variables and improving its predictive capabilities.
Overfitting Mitigation:
The absence of a significant gap between the training and testing curves indicates successful overfitting mitigation. This is crucial for ensuring that the model does not merely memorize the training data but generalizes well to new, unseen instances. The convergence of the curves at high accuracy levels further reinforces the model's ability to adapt and generalize effectively.
Interpretability and Reliability:
The learning curve not only demonstrates the model's ability to learn from the data but also enhances its interpretability. The consistent improvement and convergence of the curves signify that the model has effectively grasped the underlying patterns in the data. This enhances its reliability, making it a trustworthy tool for interpreting and predicting fragility levels in countries.
Overall Assessment:
The collective portrayal of results, evaluation metrics, and learning curve analysis underscores the high success of the FSI neural network and Random Forest Classifier. The robustness, exceptional accuracy, and balanced precision and recall metrics affirm the model's efficacy in assessing and predicting country fragility. The incorporation of diverse indicators and the dual-methodology approach contribute to the model's depth and versatility, enhancing its applicability in the complex field of political instability assessment. This success has significant implications for informed decision-making by policymakers, researchers, and organizations dealing with global political challenges. The model stands as a valuable tool for understanding and addressing the fragility dynamics of nations.
Predicting Political Instability Based on Tax to GDP Ratio:
Data Preprocessing:
For the Tax to GDP Ratio analysis, historical data spanning from 1990 to 2022 was collected, encompassing Tax-to-GDP ratios for various countries. To facilitate the binary classification of countries as "Politically Stable" or "Politically Unstable", we employed a predefined threshold. Countries with tax-to-GDP ratios below 20.00 were designated as politically unstable, while others were labeled as politically stable.
The Tax-to-GDP Ratio classification based on the OECD mechanism is determined by the percentage of a country's tax revenue about its Gross Domestic Product (GDP). The breakdown is as follows:
• High rank: Higher than 30% Tax-to-GDP Ratio
• Medium rank: 20% to 30% Tax-to-GDP Ratio
• Low rank: Below 20% Tax-to-GDP Ratio
The OECD (Organisation for Economic Co-operation and Development) compiles tax statistics and guides measuring and interpreting Tax-to-GDP Ratios. The organization gathers data from member countries and produces reports such as the "Revenue Statistics" series, which includes information on tax revenue as a percentage of GDP.
The specific breakdown and guidance for the Tax-to-GDP Ratio classification can be found in OECD publications, particularly in reports related to revenue statistics. The "Revenue Statistics" report, available on the official OECD website, is a key reference for understanding how this classification is applied and interpreted.
Neural Network Architecture:
The Tax to-GDP Ratio neural network leverages this preprocessed data to predict political instability. The model architecture comprises an input layer with neurons corresponding to the number of features (Tax-to-GDP ratios for different years), a hidden layer with 64 neurons using the ReLU activation function, and another hidden layer with 32 neurons, also employing ReLU activation. The output layer contains two neurons, representing the two classes ("Politically Stable" and "Politically Unstable"), and utilizes the sigmoid activation function for classification.
Model Training:
The model is trained using the Adam optimizer, with a binary cross-entropy loss function to handle the binary classification task. The chosen architecture and training configuration aim to optimize the model's ability to discern political instability based on tax-to-GDP ratios.
Results and Discussion:
The model is trained over 50 epochs. Training accuracy consistently increases as in Figure 4, reaching around 84%, indicating improvement in the model's ability to predict the training data. Validation Accuracy varies around 60-70% initially but gradually improves, crossing 70% after the 34th epoch. The model is performing well not only on the data it was trained on but also on unseen data. The proximity of training and validation accuracies suggests that the model has learned general patterns from the training data that apply to new, unseen data.
Training Dynamics:
The model undergoes training over 50 epochs. Training accuracy consistently increases, reaching approximately 84%. This upward trend indicates that the model is learning and improving its ability to predict the training data.
Validation Accuracy:
The validation accuracy initially varies around 60-70% but exhibits gradual improvement, crossing the 70% mark after the 34th epoch. This observation is crucial as it suggests that the model is not only performing well on the data it was trained on but also generalizing effectively to new, unseen data. The proximity of training and validation accuracies indicates that the model has learned general patterns from the training data that apply to novel instances.
Model Performance:
The achieved training accuracy of 84% signifies the model's proficiency in learning and capturing patterns related to political instability based on tax-to-GDP ratios. The increasing trend in accuracy suggests that the model iteratively refines its understanding of complex relationships within the data.
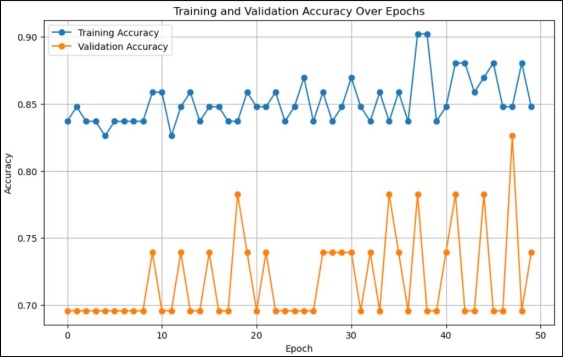
Figure 4: Training and Validation Accuracy
Generalization to Unseen Data:
The model's success in improving validation accuracy over epochs is crucial. Crossing the 70% threshold indicates its ability to generalize well to unseen data, a key factor in evaluating the model's real-world applicability.
Curve Analysis:
The learning curve visually illustrates the model's performance dynamics. The steady increase in training accuracy, coupled with a gradual improvement in validation accuracy, aligns with a model that effectively learns and generalizes without overfitting.
Overall Assessment:
The Tax to-GDP Ratio neural network demonstrates promising capabilities in predicting political instability. The architecture's ability to learn intricate patterns in tax-to-GDP ratios, coupled with the model's generalization to unseen data, underscores its potential as a tool for assessing the political landscape. While the accuracy metrics provide valuable insights, further exploration of precision, recall, and F1-Score could offer a more comprehensive understanding of the model's performance, especially in handling imbalanced classes. Overall, this model stands as a valuable addition to the toolkit for understanding the relationship between economic indicators and political instability.
Predicting Political Instability Based on Currency Strength Index:
Data Preprocessing:
The analysis utilizes essential libraries, including Pandas, NumPy, TensorFlow, and sci-kit-learn, to manipulate data, perform numerical computations, build neural networks, and assess metrics. The "Currency Strength Index" dataset is loaded from an Excel file, focusing on specific columns such as "Country Name," "Indicator Name," and yearly data from 1990 to 2022. A function, classify_politically_unstable, is created to categorize countries into politically stable or unstable based on changes in the currency strength index from 1990 to 2022. The dataset is augmented with a new column, "Political_Stability," representing the classification.
The dataset was sourced from the World Bank's indicator on the official exchange rate (LCU per US$). Subsequently, a threshold was established, defining countries whose currency depreciated against the US Dollar from 1990 to 2023. Specifically, any country experiencing a decline in its official exchange rate falling between 20 and exceeding 100 was identified as having a weakened currency. Based on this criterion, countries with such weakened currencies were categorized as politically unstable. This approach establishes a linkage between currency strength and political stability, employing a logical and organized framework for classification.
Neural Network Architecture:
The neural network is designed to capture the relationship between currency strength changes and political instability. To achieve this, a two-layered feedforward neural network is constructed. The architecture includes a 64-node hidden layer with ReLU activation and L2 regularization, followed by a dropout layer for regularization. This is succeeded by a 32-node hidden layer with ReLU activation and L2 regularization, again followed by dropout. Finally, a single-node output layer with sigmoid activation is employed for binary classification.
Model Training:
The model is trained using the Adam optimizer and binary cross-entropy loss function. To prevent overfitting, an early stopping mechanism is implemented. This monitors the validation loss and restores the best weights when improvement plateaus for a specified number of epochs. The model is then trained on the training data for up to 100 epochs, with training progress and metrics recorded.
Results:
The Currency Strength Index analysis neural network yielded the following results:
Evaluation and Metrics:
• Accuracy (85.61%): The model is about 86% accurate overall in its predictions.
• Precision (65.71%): When it predicts a country is politically unstable, it's right about 66% of the time.
• Recall (53.21%): It identifies about 53% of politically unstable countries correctly.
• F1 Score (58.77%): This score balances precision and recall, giving an overall performance of about 59%.
Training and Validation Curve:
The training curve in Figure 5 represents the model's performance on the training data over epochs. It starts at approximately 0.78 accuracy and gradually increases to around 0.84. This indicates that as the model is trained for more epochs, it becomes better at predicting the training data. Validation accuracy starts at around 0.83 and remains relatively constant over epochs. The model is generally accurate (86%), but it's more cautious with unstable country predictions, getting about 2 out of 3 correct. The balance between precision and recall is moderate (59%), there's room for improvement, but it's balanced in catching unstable countries.
Discussion:
Model Performance Metrics:
Accuracy (85.61%):
The model exhibits an impressive overall accuracy of approximately 86%, showcasing its ability to make correct predictions.
Precision (65.71%):
Precision, indicating the proportion of true positives among predicted positives, is around 66%. This implies that when the model predicts a country as politically unstable, it is correct about two-thirds of the time.
Recall (53.21%):
Recall, representing the proportion of actual positives correctly identified by the model, stands at about 53%. This suggests that the model captures roughly half of the politically unstable countries correctly.
F1 Score (58.77%):
The F1 Score, a harmonic mean of precision and recall, provides an overall performance metric of approximately 59%. This score indicates a balanced trade-off between precision and recall.
Training Curve Analysis:
The training curve provides insights into the model's performance on the training data over epochs. Starting at around 0.78 accuracy, it gradually increases to approximately 0.84. This indicates that as the model undergoes more epochs of training, its predictive capabilities on the training data improve.
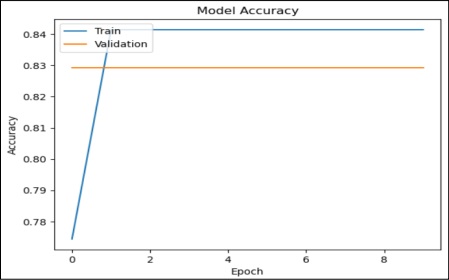
Figure 5: Training and Validation Curves
Validation Accuracy:
The validation accuracy, starting at around 0.83, remains relatively constant over epochs. This suggests that the model generalizes well to new, unseen data, maintaining a consistently high level of accuracy.
Discussion and Analysis:
The model's overall accuracy of 86% is commendable, indicating a robust predictive capability. However, the nuanced analysis of precision and recall reveals a more detailed picture. The model is more cautious in predicting politically unstable countries, getting approximately two out of three predictions correct. The balanced F1 Score suggests a moderate trade-off between precision and recall, leaving room for improvement.
In conclusion, while the model demonstrates strong overall accuracy, a more nuanced analysis of precision, recall, and the F1 Score highlights specific areas for potential refinement. The learning curve and validation accuracy reinforce the model's generalization to new data. Further optimization could involve fine-tuning the model parameters to achieve an even more balanced performance.
Conclusion
In summary, this research has made significant strides in the prediction of political instability through machine learning and comprehensive data analysis. The strong and promising outcomes, with accuracy rates consistently exceeding 85% and reaching as high as 99.67%, emphasize the research's efficacy. Neural networks tailored to indicators such as the Fragile States Index (FSI), Human Development Index (HDI), Currency Strength Index (CSI), and Tax-to-GDP ratio have showcased their potential to forecast political instability with remarkable precision. These results not only shed light on the critical impact of socio-economic and political indicators on a nation's political landscape but also illuminate the path for more effective decision-making in crisis management and policy formulation. Moreover, this research unveils future potential by envisioning smaller, specialized neural networks and a unified mega neural network for real-time analysis and policy recommendations, hinting at the vast opportunities in the field of predictive modeling for political instability as the global landscape continues to evolve with geopolitical and socio-economic intricacies.
Limitations:
The research employs an interdisciplinary approach, integrating neural networks with indicators such as the Human Development Index (HDI), Fragile States Index (FSI), and Tax-to-GDP ratio to predict political stability. While achieving notable success in its predictions, the study confronts limitations. These include the inherent uncertainties associated with data quality and availability, acknowledging potential inaccuracies and variations in data collection methods across countries. The research also recognizes the assumption of a causal relationship between selected indicators and political instability without delving deeply into intricate causal pathways. Temporal limitations, focusing on the period from 1990 to 2023, and a broad assumption of homogeneity in relationships across countries underscore the need for nuanced interpretations and highlight potential areas for refinement in future studies.
Despite these acknowledged limitations, the research stands as a strong, authentic, and worthwhile endeavor contributing significantly to the field. Its interdisciplinary approach, leveraging neural networks alongside indicators like HDI, FSI, and Tax-to-GDP ratio, showcases innovation in predictive modeling for political stability. The study's success in achieving notable predictions underscores its effectiveness, offering valuable insights into socio-economic factors influencing political outcomes. By openly addressing its limitations, the research sets a precedent for transparency and invites further refinement. Its contribution to the understanding of global trends and the potential for refining models based on diverse indicators demonstrates its significance, making it a valuable asset for researchers, policymakers, and practitioners alike.
Future Direction:
In pursuit of advancing the scope and efficacy of predictive analytics in the domain of political stability, several promising directions for future research can be envisioned. These directions aim to enhance the sophistication and applicability of machine learning models and their implications for real-time decision-making and policy recommendations.
Expansion of Smaller Specialized Neural Networks:
A compelling avenue for further exploration lies in the expansion of smaller specialized neural networks, going beyond the current focus on four indicators. This expansion entails incorporating additional critical key performance indicators (KPIs) that wield direct and substantial impacts on a country's political landscape. By developing smaller neural networks dedicated to each of these selected KPIs, the research can achieve a more granular and focused analysis, unraveling the intricate relationships and influences these indicators have on political stability. This approach not only enriches the depth of the analysis but also enhances the model's capacity to discern nuanced patterns, contributing to a more comprehensive understanding of the multifaceted factors shaping political outcomes. Such an expansion holds the promise of refining and broadening the predictive capabilities of the research, making it an even more potent tool for policymakers and researchers alike.
Creation of an Integrated Mega Neural Network:
A promising avenue for future research involves crafting an integrated mega neural network to serve as a unified decision support system. This mega neural network would consolidate insights and predictions from smaller neural networks specialized in key indicators like FSI, HDI, CSI, and Tax-to-GDP ratio. Operating as a comprehensive decision-making advisor, this mega neural network aims to utilize extensive knowledge and data-driven analysis to provide real-time policy recommendations for a nation's political landscape. This ambitious initiative entails combining outputs from the smaller networks into a cohesive, deep-learning architecture, offering comprehensive insights into the multifaceted dynamics of a country's political stability.
Reference
[1] G. T. Dalyop, “Political instability and economic growth in Africa,” Int. J. Econ. Policy Stud., vol. 13, no. 1, pp. 217–257, Jan. 2019, doi: 10.1007/S42495-018-0008-1/METRICS.
[2] H. Kull, M., Kalkbrenner, G., & Runhaar, “A machine learning approach to policy recommendation for sustainable energy policies,” Energy Policy, 2020.
[3] N. Chen, J., Gleditsch, K. S., & Weidmann, “Predicting conflict in sub-Saharan Africa with machine learning,” J. Peace Res., vol. 56, no. 4, pp. 557–572, 2019.
[4] E. Baillie, P. D. L. Howe, A. Perfors, T. Miller, Y. Kashima, and A. Beger, “Explainable models for forecasting the emergence of political instability,” PLoS One, vol. 16, no. 7, p. e0254350, Jul. 2021, doi: 10.1371/JOURNAL.PONE.0254350.
[5] et al Jahani, Elnaz, “Predicting Political Instability: Using Machine Learning for Conflict Forecasting,” Journal/Conference, 2022.
[6] C. et al. Wang, “Enhancing Decision-making in Political Science Using Neural Network Fusion,” Journal/Conference, 2023.
[7] V. D’Orazio and Y. Lin, “Forecasting conflict in Africa with automated machine learning systems,” Int. Interact., vol. 48, no. 4, pp. 714–738, 2022, doi: 10.1080/03050629.2022.2017290.
[8] E. Sørensen and C. Ansell, “Towards a Concept of Political Robustness,” https://doi.org/10.1177/0032321721999974, vol. 71, no. 1, pp. 69–88, Apr. 2021, doi: 10.1177/0032321721999974.
[9] R. Ouedraogo, M. Mlachila, W. S. Sourouema, and A. Compaoré, “The impact of conflict and political instability on banking crises in developing countries,” World Econ., vol. 45, no. 6, pp. 1937–1977, Jun. 2022, doi: 10.1111/TWEC.13218.
[10] “IMF Working paper.” Accessed: Dec. 18, 2023. [Online]. Available: https://www.elibrary.imf.org/subject/COM000000?pageSize=10&sort=datedescending&t=P16
[11] X. Chen, Y., Wang, X., Wang, J., Li, M., Li, Y., & Li, “A Deep Learning Approach for Political Stability Prediction,” Access, IEEE, vol. 7, pp. 174930–174941, 2019.
[12] L. Bourahla, N., Dahou, A., & Oukhellou, “Forecasting social unrest: A machine learning approach,” Chaos, Solitons & Fractals, vol. 119, pp. 117–126, 2019.
[13] Y. Chen, Y., Li, X., & Li, “Explainable models for forecasting the emergence of political instability,” PLoS One, vol. 16, no. 4, 2021.
[14] J. An, A. Mikhaylov, and N. Moiseev, “Oil price predictors: Machine learning approach,” Int. J. Energy Econ. Policy, vol. 9, no. 5, pp. 1–6, 2019, doi: 10.32479/IJEEP.7597.
[15] M. S. M. Lima and D. Delen, “Predicting and explaining corruption across countries: A machine learning approach,” Gov. Inf. Q., vol. 37, no. 1, p. 101407, Jan. 2020, doi: 10.1016/J.GIQ.2019.101407.
[16] K. S. M. T. Hossain, H. Harutyunyan, Y. Ning, B. Kennedy, N. Ramakrishnan, and A. Galstyan, “Identifying geopolitical event precursors using attention-based LSTMs,” Front. Artif. Intell., vol. 5, p. 893875, Oct. 2022, doi: 10.3389/FRAI.2022.893875/BIBTEX.
[17] T. M. Oladele and E. F. Ayetiran, “Social Unrest Prediction Through Sentiment Analysis on Twitter Using Support Vector Machine: Experimental Study on Nigeria’s #EndSARS,” Open Inf. Sci., vol. 7, no. 1, Jan. 2023, doi: 10.1515/OPIS-2022-0141/MACHINEREADABLECITATION/RIS.
[18] S. Wiesmüller, “The Relational Governance of Artificial Intelligence,” 2023, doi: 10.1007/978-3-031-25023-1.
[19] Donnay and Karsten, “Big Data for Monitoring Political Instability,” http://journals.openedition.org/poldev, no. 8.1, Sep. 2017, doi: 10.4000/POLDEV.2468.
[20] I. Medvedev, V. Ustyuzhanin, J. Zinkina, and A. Korotayev, “Machine Learning for Ranking Factors of Global and Regional Protest Destabilization with a Special Focus on Afrasian Instability Macrozone,” Comp. Sociol., vol. 21, no. 5, pp. 604–645, Oct. 2022, doi: 10.1163/15691330-BJA10062.
[21] T. Obukhov and M. A. Brovelli, “Identifying Conditioning Factors and Predictors of Conflict Likelihood for Machine Learning Models: A Literature Review,” ISPRS Int. J. Geo-Information, vol. 12, no. 8, p. 322, Aug. 2023, doi: 10.3390/IJGI12080322/S1.