Dataset Description:
The Alzheimer's disease dataset that was used in this research is crucial for the development and evaluation of machine-learning models aimed at detecting and diagnosing Alzheimer's disease. Based on the degree of dementia, the individuals in the dataset are divided into four different classes: mildly demented, moderately demented, non-demented, and very mildly demented. Details are given in Table 2.
Training Set:
The training set consists of a diverse range of individuals, each categorized into one of the four dementia severity classes.
Mild Demented:
This category includes 717 individuals exhibiting mild symptoms of dementia. These individuals
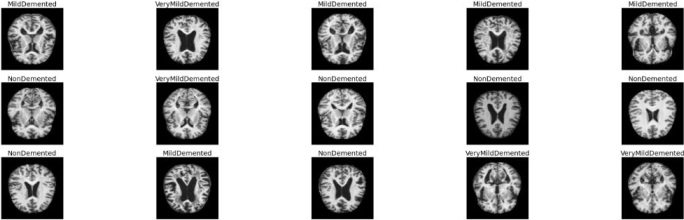
Figure 4: Image from Dataset [15].exhibited modest memory loss and cognitive decline, which are common indicators of subtle cognitive deficits.
Moderate Demented:
A smaller subset of individuals, comprising 52 cases, falls under this category. These individuals demonstrate more pronounced symptoms of dementia, with noticeable cognitive impairments affecting their daily functioning.
Non-Demented:
The largest category within the training set, consisting of 2560 individuals, encompasses those who do not exhibit symptoms of dementia. These individuals serve as the control group, providing a baseline for comparison with individuals in the dementia categories.
Very Mild Demented:
This category comprises 1792 individuals exhibiting early signs of dementia. Despite their relatively mild symptoms, individuals in this category may experience subtle memory lapses and cognitive deficits.
Test Set:
The test set follows a similar structure, encompassing individuals across the four dementia severity categories for the purpose of model evaluation.
Mild Demented:
The test set includes 179 individuals presenting with mild symptoms of dementia, mirroring the distribution observed in the training set.
Moderate Demented:
A smaller subset of individuals, totaling 12 cases, falls under this category in the test set.
Non-Demented:
Similar to the training set, the non-demented category in the test set comprises the largest subset, with 640 individuals serving as controls.
Very Mild Demented:
This category in the test set includes 448 individuals exhibiting early signs of dementia, providing a valuable cohort for evaluating model performance on early-stage dementia detection. The comprehensive nature of this dataset, encompassing individuals across various dementia severity levels, enables robust machine learning models' creation and assessment for the identification of Alzheimer's disease. The balanced distribution of individuals across the training and test sets ensures reliable model training and evaluation, ultimately contributing to advancements in Alzheimer's disease diagnosis and patient care.
Table 2: Details of Dataset.
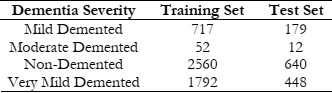
Results and Discussion:
We conducted a thorough evaluation process by dividing the dataset into separate training and testing subsets to gauge the efficacy of our suggested study. This division ensured a robust assessment of our model's performance across different data samples. The training set included eighty percent of the entire dataset, facilitating the model to learn patterns and features from a significant portion of the data. In contrast, the testing set, constituting 20% of the dataset, served as an independent validation cohort to evaluate the model's generalization ability. We utilized four key evaluation metrics including accuracy, precision, recall, and F1-score to measure the effectiveness of our suggested model, AlzheimerNet-V3. The capacity of the model to accurately categorize instances is just one of the many aspects of performance that these metrics shed light on to minimize false positives, and capture all relevant instances of a given class. Table 3 presents a detailed summary of performance metrics achieved by AlzheimerNet-V3. The model exhibited an impressive accuracy of 94.06 %, acknowledging its capability to correctly classify a vast majority of instances across all classes. Furthermore, the model demonstrated a high precision of 94.06%, highlighting its proficiency in minimizing false positives by accurately identifying true positive instances. The recall metric, which measures the model's ability to capture all relevant instances of a class, was also notable, with AlzheimerNet-V3 achieving a recall of 94.06%. Additionally, the F1-score, which represents the harmonic mean of precision and recall, further underscored the robustness of our model, reaching a value of 94.06%. In addition to evaluating our proposed model, we conducted comparative analyses with several other pre-trained models as well as ResNet-101, VGG-16, VGG-19, Mobile-Net-v2, and Mobile-Net-v3. These models were applied to the same Alzheimer's dataset to benchmark their performance against AlzheimerNet-V3 [15].
The results of our comparative analysis revealed notable performance variations across the different pre-trained models. While each model exhibited certain strengths, AlzheimerNet-V3 consistently outperformed its counterparts across all evaluated metrics. Notably, our model compared to VGG-16, VGG-19, Mobile-Net-v2, Mobile-Net-v3, and ResNet-101, attained superior accuracy, precision, recall, and F1-score. Overall, the superior performance of AlzheimerNet-V3 underscores its efficacy as a robust and reliable model for Alzheimer's disease. Our suggested approach shows great potential for improving patient outcomes and diagnostic accuracy in the management of Alzheimer's disease by exceeding current pre-trained models.
Table 3: Overall Functioning of the Suggested Model.
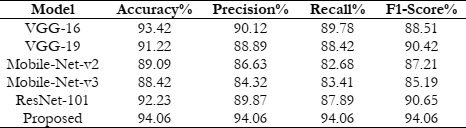
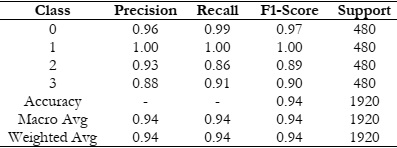
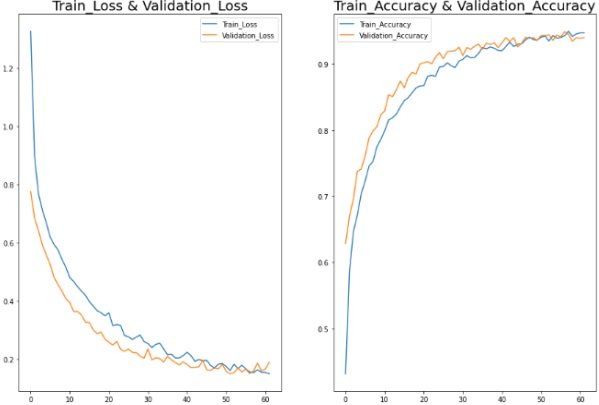
Figure 5: (a) Train Loss and Validation Loss, (b) Train Accuracy and Validation Accuracy.
Figure 5 (a) shows the train loss and validation loss while Figure 5 (b) shows the train accuracy and validation accuracy of the proposed AlzheimerNet-V3 approach. Next, we created a confusion matrix for multiple classes. Confusion matrices serve as valuable tools for quantitatively assessing the classification performance of a system on test data. They offer insights into various types of classification errors, enabling the calculation of multiple evaluation metrics. We used the confusion matrix in our study to calculate measures like recall, accuracy, precision, and F1-score. Table 4 presents the confusion matrix produced by our suggested system's testing data, which aims to differentiate between people with Alzheimer's disease and healthy people using the AlzheimerNet-V3 architecture. These results underscore the efficacy of the AlzheimerNet-V3 architecture in efficiently detecting Alzheimer's patients, indicating its potential utility in healthcare settings for Alzheimer's detection.
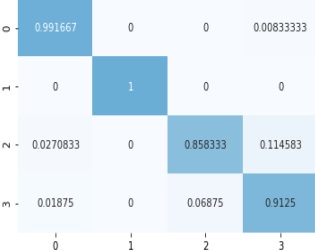
Figure 6: Normalized Confusion Matrix.
Here are the details shown in the table, the correct prediction, and the misclassification results.
Table 4: Confusion Matrix.
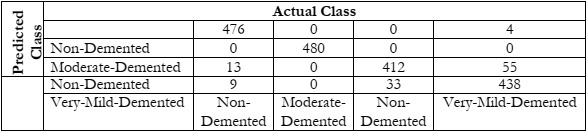
Here are the details of the other metrics such as precision, recall, and F1-score in Table 5.
Table 5: Performance of AlzheimerNet-V3.
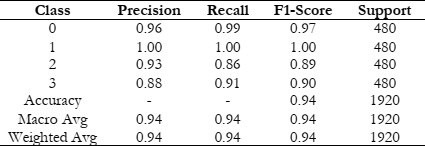
For Class 0, a precision of 0.96 suggested that when the model predicts a sample as belonging to Class 0, it is accurate approximately 96% of the time. The recall of 0.99 suggested that the model identifies about 99% of the actual Class 0 samples. The F1-score of 0.97, which is the harmonic mean of precision and recall, provided a balanced measure of the model's performance for Class 0. A similar interpretation applies to Class 1, with precision, recall, and F1-score all having 1.00, the model performs exceptionally well in identifying samples belonging to Class 1, achieving perfect scores for precision, recall, and F1-score. For these classes, the precision, recall, and F1 scores are slightly lower compared to Class 0 and 1, indicating that the model may have some difficulty in accurately identifying samples in these classes. However, the overall performance is still respectable, with F1 scores above 0.88 for both classes. However, the performance of a thorough comparative analysis between our proposed AlzheimerNet-V3 system and other contemporary methods is essential to gauge its effectiveness. Through this comparison, we aim to highlight the strengths and advantages of our approach to Alzheimer's detection. In the realm of Alzheimer's detection, CNNs have emerged as a popular choice among researchers. Table 6 provides an overview of alternative methods utilized by various authors for this purpose. For instance, Tian et al. [16] presented the MTFIL-NET model, which had an 86.00% accuracy rate in identifying Alzheimer's patients. LSTM+FS architecture, causing an accuracy of 88.00%. Using the EfficientNetB0 pre-trained CNN model, Savas et al. [14] were able to achieve a 92.98% accuracy rate. Moreover, Liu et al. [17] designed a deep separable CNN model, detecting Alzheimer's patients with 78.02% accuracy. Conversely, our AlzheimerNet-V3 model outperformed these findings, exhibiting a remarkable 94.06% accuracy, as illustrated in Table 6. This notable performance enhancement underscores the efficacy and potential of our proposed architecture, when it comes to identifying Alzheimer's illness. Furthermore, our approach offers several advantages, including robustness, scalability, and efficiency, making it a promising candidate for real-world applications in healthcare settings. Through this comprehensive comparative analysis, we aim to provide compelling evidence supporting the superiority of our AlzheimerNet-V3 system over other contemporary methods. We believe that our approach represents a significant advancement in Alzheimer's detection and holds promise for improving patient outcomes and healthcare practices [16].
Table 6: Comparing Performance Using Different Methods.
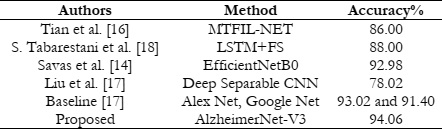
Conclusion
The proposed study addresses the pressing issue of Alzheimer's disease detection, a condition that represents a significant burden on global healthcare systems and individuals' quality of life. It impacts millions of people every year in the early stages of dementia. Early diagnosis is crucial for Alzheimer's sufferers, as symptoms may not worsen significantly if the disease is detected in its early stages. Considering the importance of this problem, creating an automated system to diagnose Alzheimer's is essential, especially in light of the rising number of older people who are suffering from dementia. In this study, we introduced a novel approach leveraging transfer learning, namely AlzheimerNet-V3, designed specifically for Alzheimer's disease detection. Built upon the Inception v3 pre-trained model, our proposed AlzheimerNet-V3 system aims to offer robust and efficient detection capabilities. In-depth, testing was also done to compare AlzheimerNet-V3's performance to other trained models that are frequently used to identify Alzheimer’s disease. Our experimental results demonstrated that AlzheimerNet-V3 outperforms all other pre-trained models in terms of accuracy, precision, recall, and F1-score. Specifically, AlzheimerNet-V3 achieved an accuracy of 94.06%. These findings highlight how well our suggested method works to detect Alzheimer’s patients with accuracy while reducing false positives. Additionally, we performed a comparison between AlzheimerNet-V3 and other cutting-edge methods to demonstrate the superiority of our methodology. The robust performance indicators achieved by AlzheimerNet-V3 indicate its potential utility in medical facilities and mental health clinics. By providing a dependable and effective method for Alzheimer’s detection, AlzheimerNet-V3 shows promise in improving patient care and management strategies in the future.
Reference
[1] K. A. Matthews et al., “Racial and ethnic estimates of Alzheimer’s disease and related dementias in the United States (2015–2060) in adults aged ≥65 years,” Alzheimer’s Dement., vol. 15, no. 1, pp. 17–24, Jan. 2023, doi: 10.1016/J.JALZ.2018.06.3063.
[2] “Dementia.” Accessed: Mar. 14, 2024. [Online]. Available: https://www.who.int/health-topics/dementia
[3] P. Scheltens, “Imaging in Alzheimer’s disease,” Dialogues Clin. Neurosci., vol. 11, no. 2, p. 191, 2009, doi: 10.31887/DCNS.2009.11.2/PSCHELTENS.
[4] M. Bari Antor et al., “A Comparative Analysis of Machine Learning Algorithms to Predict Alzheimer’s Disease,” J. Healthc. Eng., vol. 2021, 2021, doi: 10.1155/2021/9917919.
[5] M. Shahbaz, S. Ali, A. Guergachi, A. Niazi, and A. Umer, “Classification of Alzheimer’s disease using machine learning techniques,” DATA 2019 - Proc. 8th Int. Conf. Data Sci. Technol. Appl., pp. 296–303, 2019, doi: 10.5220/0007949902960303.
[6] S. Alam, G. R. Kwon, J. I. Kim, and C. S. Park, “Twin SVM-Based Classification of Alzheimer’s Disease Using Complex Dual-Tree Wavelet Principal Coefficients and LDA,” J. Healthc. Eng., vol. 2017, 2017, doi: 10.1155/2017/8750506.
[7] S. Al-Shoukry, T. H. Rassem, and N. M. Makbol, “Alzheimer’s diseases detection by using deep learning algorithms: A mini-review,” IEEE Access, vol. 8, pp. 77131–77141, 2020, doi: 10.1109/ACCESS.2020.2989396.
[8] T. A. Tuan, T. B. Pham, J. Y. Kim, and J. M. R. S. Tavares, “Alzheimer’s diagnosis using deep learning in segmenting and classifying 3D brain MR images,” Int. J. Neurosci., vol. 132, no. 7, pp. 689–698, 2022, doi: 10.1080/00207454.2020.1835900.
[9] S. Murugan et al., “DEMNET: A Deep Learning Model for Early Diagnosis of Alzheimer Diseases and Dementia from MR Images,” IEEE Access, vol. 9, pp. 90319–90329, 2021, doi: 10.1109/ACCESS.2021.3090474.
[10] C. Feng et al., “Deep Learning Framework for Alzheimer’s Disease Diagnosis via 3D-CNN and FSBi-LSTM,” IEEE Access, vol. 7, pp. 63605–63618, 2019, doi: 10.1109/ACCESS.2019.2913847.
[11] S. Naz, A. Ashraf, and A. Zaib, “Transfer learning using freeze features for Alzheimer neurological disorder detection using ADNI dataset,” Multimed. Syst., vol. 28, no. 1, pp. 85–94, Feb. 2022, doi: 10.1007/S00530-021-00797-3/METRICS.
[12] T. M. Ghazal et al., “Alzheimer Disease Detection Empowered with Transfer Learning,” Comput. Mater. Contin., vol. 70, no. 3, pp. 5005–5019, Oct. 2021, doi: 10.32604/CMC.2022.020866.
[13] N. M. Khan, N. Abraham, and M. Hon, “Transfer Learning with Intelligent Training Data Selection for Prediction of Alzheimer’s Disease,” IEEE Access, vol. 7, pp. 72726–72735, 2019, doi: 10.1109/ACCESS.2019.2920448.
[14] S. Savaş, “Detecting the Stages of Alzheimer’s Disease with Pre-trained Deep Learning Architectures,” Arab. J. Sci. Eng., vol. 47, no. 2, pp. 2201–2218, Feb. 2022, doi: 10.1007/S13369-021-06131-3/METRICS.
[15] “Kaggle: Your Home for Data Science.” Accessed: Mar. 14, 2024. [Online]. Available: https://www.kaggle.com/code/tourist55/alzheimers-dataset-4-class-of-images
[16] J. Liu, X. Tian, J. Wang, R. Guo, and H. Kuang, “MTFIL-Net: Automated Alzheimer’s disease detection and MMSE score prediction based on feature interactive learning,” Proc. - 2021 IEEE Int. Conf. Bioinforma. Biomed. BIBM 2021, pp. 1002–1007, 2021, doi: 10.1109/BIBM52615.2021.9669563.
[17] J. Liu, M. Li, Y. Luo, S. Yang, W. Li, and Y. Bi, “Alzheimer’s disease detection using depthwise separable convolutional neural networks,” Comput. Methods Programs Biomed., vol. 203, p. 106032, May 2021, doi: 10.1016/J.CMPB.2021.106032.
[18] S. Tabarestani et al., “Longitudinal prediction modeling of Alzheimer disease using recurrent neural networks,” 2019 IEEE EMBS Int. Conf. Biomed. Heal. Informatics, BHI 2019 - Proc., May 2019, doi: 10.1109/BHI.2019.8834556.