Investigating the Impact of Socio-Economic Factors on Agricultural Productivity: A Case Study of Bahawalpur, Pakistan
Keywords:
Socio Economic Factors, Consumer Price Index, Agricultural ProductivityAbstract
This study investigates the intricate relationship between socio-economic factors and agricultural productivity in Bahawalpur, Pakistan, an agriculturally significant region. Through a comprehensive analysis of key indicators such as literacy rate, number of households, inflation, and consumer price index, the research aims to provide insights into the dynamics shaping agricultural outcomes. Methodologically, a combination of secondary data sources and primary data collection methods, including surveys and field observations, were employed to ensure the reliability and validity of the findings. Results highlight the significant influence of socio-economic factors on agricultural productivity, with notable trends observed in variables such as yield, area under cultivation, literacy rate, and inflation. Despite the complexity of these relationships, the study underscores the importance of considering socio-economic dynamics in agricultural development efforts. Recommendations stemming from the findings emphasize the need for holistic approaches to promote agricultural sustainability and socio-economic development in the region. Overall, this study contributes valuable insights for informed decision-making and policy formulation aimed at enhancing agricultural productivity and rural livelihoods in Bahawalpur, Pakistan.
References
Y. He, B. J. Revel, B. Leng, and Z. Feng, “The effects of weather on oilseed rape (OSR) yield in China: Future implications of climate change,” Sustain., vol. 9, no. 3, Mar. 2017, doi: 10.3390/SU9030418.
M. Zheng, W. Terzaghi, H. Wang, and W. Hua, “Integrated strategies for increasing rapeseed yield,” Trends Plant Sci., vol. 27, no. 8, pp. 742–745, Aug. 2022, doi: 10.1016/J.TPLANTS.2022.03.008.
W. Crop yield and production responses to climate disasters in ChinaShi, M. Wang, and Y. Liu, “Crop yield and production responses to climate disasters in China,” Sci. Total Environ., vol. 750, Jan. 2021, doi: 10.1016/J.SCITOTENV.2020.141147.
Y. Kang, S. Khan, and X. Ma, “Climate change impacts on crop yield, crop water productivity and food security - A review,” Prog. Nat. Sci., vol. 19, no. 12, pp. 1665–1674, 2009, doi: 10.1016/J.PNSC.2009.08.001.
B. Liu et al., “Comparison of yield prediction models and estimation of the relative importance of main agronomic traits affecting rice yield formation in saline-sodic paddy fields,” Eur. J. Agron., vol. 148, Aug. 2023, doi: 10.1016/J.EJA.2023.126870.
C. González, J. Mira-McWilliams, and I. Juárez, “Important variable assessment and electricity price forecasting based on regression tree models: Classification and regression trees, Bagging and Random Forests,” IET Gener. Transm. Distrib., vol. 9, no. 11, pp. 1120–1128, Aug. 2015, doi: 10.1049/IET-GTD.2014.0655.
S. Lu, X. Bai, W. Li, and N. Wang, “Impacts of climate change on water resources and grain production,” Technol. Forecast. Soc. Change, vol. 143, pp. 76–84, Jun. 2019, doi: 10.1016/J.TECHFORE.2019.01.015.
D. Liu, X. Zhu, and Y. Wang, “China’s agricultural green total factor productivity based on carbon emission: An analysis of evolution trend and influencing factors,” J. Clean. Prod., vol. 278, Jan. 2021, doi: 10.1016/J.JCLEPRO.2020.123692.
“View of Conversion of Fertile Agricultural Land into Built-Up by Estimation of Pixel Based Land Surface Temperature (LST).” Accessed: Feb. 22, 2024. [Online]. Available: https://journal.50sea.com/index.php/IJASD/article/view/469/957
J. Zhang and Y. Liu, “Decoupling of impact factors reveals the response of cash crops phenology to climate change and adaptive management practice,” Agric. For. Meteorol., vol. 322, Jul. 2022, doi: 10.1016/J.AGRFORMET.2022.109010.
M. Shahhosseini, G. Hu, and S. V. Archontoulis, “Forecasting Corn Yield With Machine Learning Ensembles,” Front. Plant Sci., vol. 11, Jul. 2020, doi: 10.3389/FPLS.2020.01120.
W. Li and P. Zhang, “Relationship and integrated development of low-carbon economy, food safety, and agricultural mechanization,” Environ. Sci. Pollut. Res., vol. 28, no. 48, pp. 68679–68689, Dec. 2021, doi: 10.1007/S11356-021-15465-2.
G. Lischeid, H. Webber, M. Sommer, C. Nendel, and F. Ewert, “Machine learning in crop yield modelling: A powerful tool, but no surrogate for science,” Agric. For. Meteorol., vol. 312, Jan. 2022, doi: 10.1016/J.AGRFORMET.2021.108698.
J. Liang, H. Li, N. Li, Q. Yang, and L. Li, “Analysis and Prediction of the Impact of Socio-Economic and Meteorological Factors on Rapeseed Yield Based on Machine Learning,” Agron. 2023, Vol. 13, Page 1867, vol. 13, no. 7, p. 1867, Jul. 2023, doi: 10.3390/AGRONOMY13071867.
D. hui FU et al., “Research progress and strategies for multifunctional rapeseed: A case study of China,” J. Integr. Agric., vol. 15, no. 8, pp. 1673–1684, Aug. 2016, doi: 10.1016/S2095-3119(16)61384-9.
M. F. Dreccer, J. Fainges, J. Whish, F. C. Ogbonnaya, and V. O. Sadras, “Comparison of sensitive stages of wheat, barley, canola, chickpea and field pea to temperature and water stress across Australia,” Agric. For. Meteorol., vol. 248, pp. 275–294, Jan. 2018, doi: 10.1016/J.AGRFORMET.2017.10.006.
X. Li, C. Chen, X. Yang, J. Xiong, and N. Ma, “The optimisation of rapeseed yield and growth duration through adaptive crop management in climate change: evidence from China,” Ital. J. Agron., vol. 17, no. 4, Dec. 2022, doi: 10.4081/IJA.2022.2104.
A. C. Hampf et al., “The biophysical and socio-economic dimension of yield gaps in the southern Amazon – A bio-economic modelling approach,” Agric. Syst., vol. 165, pp. 1–13, Sep. 2018, doi: 10.1016/J.AGSY.2018.05.009.
J. Cao et al., “Integrating Multi-Source Data for Rice Yield Prediction across China using Machine Learning and Deep Learning Approaches,” Agric. For. Meteorol., vol. 297, Feb. 2021, doi: 10.1016/J.AGRFORMET.2020.108275.
M. Shahhosseini, R. A. Martinez-Feria, G. Hu, and S. V. Archontoulis, “Maize yield and nitrate loss prediction with machine learning algorithms,” Environ. Res. Lett., vol. 14, no. 12, Dec. 2019, doi: 10.1088/1748-9326/AB5268.
M. Naghdyzadegan Jahromi et al., “Developing machine learning models for wheat yield prediction using ground-based data, satellite-based actual evapotranspiration and vegetation indices,” Eur. J. Agron., vol. 146, May 2023, doi: 10.1016/J.EJA.2023.126820.
M. Shahhosseini, G. Hu, I. Huber, and S. V. Archontoulis, “Coupling machine learning and crop modeling improves crop yield prediction in the US Corn Belt,” Sci. Rep., vol. 11, no. 1, Dec. 2021, doi: 10.1038/S41598-020-80820-1.
J. Han et al., “Prediction of winter wheat yield based on mul[1] J. Han et al., ‘Prediction of winter wheat yield based on multi-source data and machine learning in China,’ Remote Sens., vol. 12, no. 2, Jan. 2020, doi: 10.3390/RS12020236.ti-source data and machine learni,” Remote Sens., vol. 12, no. 2, Jan. 2020, doi: 10.3390/RS12020236.
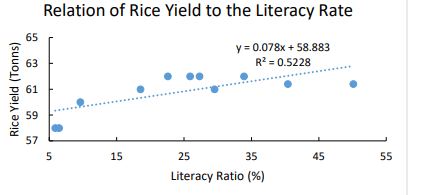