Predictive Maintenance in Industrial Internet of Things: Current Status
Keywords:
Predictive Maintenance (PdM), Industrial Internet of Things (IIoT), Artificial Intelligence, Machine Learning (ML), Industry 4.0.Abstract
Introduction/Importance of Study: Predictive Maintenance (PdM) is a key challenge within the Industrial Internet of Things (IIoT). It aims to enhance system operations by minimizing equipment failures, leading to smoother operations and increased productivity. By anticipating maintenance needs before failures occur, PdM ensures more reliable and efficient industrial processes.
Novelty Statement: This study examines maintenance techniques and datasets that leverage AI and ML for predictive maintenance in the context of industrial IoT. The primary goal is to enhance productivity, identify faults before failures occur, and minimize downtime. By utilizing advanced algorithms, the study aims to improve the efficiency and reliability of industrial systems.
Material and Method: A systematic literature review of state-of-the-art predictive maintenance in the context of industrial IoT, incorporating machine learning (ML) and artificial intelligence (AI) methods, is conducted. This review is based on research articles retrieved from the Dimensions.ai database, covering publications from 2018 to 2024.
Result and Discussion: This comprehensive analysis offers valuable insights for advancing Predictive Maintenance (PdM) strategies in the Industrial Internet of Things (IIoT), ultimately contributing to more efficient manufacturing processes. The study highlights leading publication venues and top keywords in this research area, providing a clear picture of emerging trends. It also explores the prognosis of PdM within the manufacturing industry. Additionally, the review discusses relevant models, methods, input variables, and datasets in the PdM and IIoT domain, with a particular focus on machine learning (ML) and artificial intelligence (AI) techniques. Among the most widely used techniques for PdM in IIoT are deep learning, artificial neural networks, and random forest.
Concluding Remarks: Subsequently, the study highlights various challenges, offering future research directions aimed at refining predictive maintenance techniques.
References
C. Krupitzer et al., “A Survey on Predictive Maintenance for Industry 4.0,” Feb. 2020, [Online]. Available: http://arxiv.org/abs/2002.08224
P. Poór, D. Ženíšek, and J. Basl, “Historical Overview of Maintenance Management Strategies: Development from Breakdown Maintenance to Predictive Maintenance in Accordance with Four Industrial Revolutions.”
P. Mallioris, E. Aivazidou, and D. Bechtsis, “Predictive maintenance in Industry 4.0: A systematic multi-sector mapping,” CIRP J Manuf Sci Technol, vol. 50, pp. 80–103, 2024, doi: https://doi.org/10.1016/j.cirpj.2024.02.003.
A. Sahli, R. Evans, and A. Manohar, “Predictive Maintenance in Industry 4.0: Current Themes,” in Procedia CIRP, Elsevier B.V., 2021, pp. 1948–1953. doi: 10.1016/j.procir.2021.11.329.
M. Alabadi, A. Habbal, and M. Guizani, “An Innovative Decentralized and Distributed Deep Learning Framework for Predictive Maintenance in the Industrial Internet of Things,” IEEE Internet Things J, Jun. 2024, doi: 10.1109/JIOT.2024.3372375.
“PREDICT | meaning in the Cambridge English Dictionary.” Accessed: May 08, 2022. [Online]. Available: https://dictionary.cambridge.org/dictionary/english/predict
V. Rastogi, S. Srivastava, M. Mishra, and R. Thukral, “Predictive Maintenance for SME in Industry 4.0,” in Proceedings - 2020 Global Smart Industry Conference, GloSIC 2020, Institute of Electrical and Electronics Engineers Inc., Nov. 2020, pp. 382–390. doi: 10.1109/GloSIC50886.2020.9267844.
Y. K. Teoh, S. S. Gill, and A. K. Parlikad, “IoT and Fog Computing based Predictive Maintenance Model for Effective Asset Management in Industry 4.0 using Machine Learning,” IEEE Internet Things J, 2021, doi: 10.1109/JIOT.2021.3050441.
B. van Oudenhoven, P. Van de Calseyde, R. Basten, and E. Demerouti, “Predictive maintenance for industry 5.0: behavioural inquiries from a work system perspective,” Int J Prod Res, vol. 61, no. 22, pp. 7846–7865, 2023, doi: 10.1080/00207543.2022.2154403.
N. Donthu, S. Kumar, D. Mukherjee, N. Pandey, and W. M. Lim, “How to conduct a bibliometric analysis: An overview and guidelines,” J Bus Res, vol. 133, pp. 285–296, Sep. 2021, doi: 10.1016/j.jbusres.2021.04.070.
B. Kitchenham and S. Charters, “Guidelines for performing Systematic Literature Reviews in Software Engineering,” Guidelines for performing Systematic Literature Reviews in SE, pp. 1–44, 2007, [Online]. Available: https://userpages.uni-koblenz.de/%7B~%7Dlaemmel/esecourse/slides/slr.pdf
D. Moher, A. Liberati, J. Tetzlaff, and D. G. Altman, “Guidelines and Guidance Preferred Reporting Items for Systematic Reviews and Meta-Analyses: The PRISMA Statement”, doi: 10.1371/journal.pmed.1000097.
S. Werbińska-Wojciechowska and K. Winiarska, “Maintenance Performance in the Age of Industry 4.0: A Bibliometric Performance Analysis and a Systematic Literature Review,” Sensors, vol. 23, no. 3. MDPI, Feb. 01, 2023. doi: 10.3390/s23031409.
A. Martín-Martín, M. Thelwall, E. Orduna-Malea, and E. Delgado López-Cózar, “Google Scholar, Microsoft Academic, Scopus, Dimensions, Web of Science, and OpenCitations’ COCI: a multidisciplinary comparison of coverage via citations,” Scientometrics, vol. 126, no. 1, pp. 871–906, Jan. 2021, doi: 10.1007/s11192-020-03690-4.
N. Donthu, S. Kumar, D. Mukherjee, N. Pandey, and W. M. Lim, “How to conduct a bibliometric analysis: An overview and guidelines,” J Bus Res, vol. 133, pp. 285–296, Sep. 2021, doi: 10.1016/j.jbusres.2021.04.070.
S. Cavalieri and M. G. Salafia, “A model for predictive maintenance based on asset administration shell,” Sensors (Switzerland), vol. 20, no. 21, pp. 1–20, Nov. 2020, doi: 10.3390/s20216028.
P. Moens et al., “Scalable fleet monitoring and visualization for smart machine maintenance and industrial iot applications,” Sensors (Switzerland), vol. 20, no. 15, pp. 1–15, Aug. 2020, doi: 10.3390/s20154308.
P. Tanuska, L. Spendla, M. Kebisek, R. Duris, and M. Stremy, “Smart anomaly detection and prediction for assembly process maintenance in compliance with industry 4.0,” Sensors, vol. 21, no. 7, Apr. 2021, doi: 10.3390/s21072376.
E. Sanz, J. Blesa, and V. Puig, “BiDrac Industry 4.0 framework: Application to an Automotive Paint Shop Process,” Control Eng Pract, vol. 109, Apr. 2021, doi: 10.1016/j.conengprac.2021.104757.
A. T. Prihatno, H. Nurcahyanto, and Y. M. Jang, “Predictive Maintenance of Relative Humidity Using Random Forest Method,” in 3rd International Conference on Artificial Intelligence in Information and Communication, ICAIIC 2021, Institute of Electrical and Electronics Engineers Inc., Apr. 2021, pp. 497–499. doi: 10.1109/ICAIIC51459.2021.9415213.
I. Abdullahi, S. Longo, and M. Samie, “Towards a Distributed Digital Twin Framework for Predictive Maintenance in Industrial Internet of Things (IIoT),” Sensors, vol. 24, no. 8, Apr. 2024, doi: 10.3390/s24082663.
S. O. Alhuqay, A. T. Alenazi, H. A. Alabduljabbar, and M. A. Haq, “Improving Predictive Maintenance in Industrial Environments via IIoT and Machine Learning,” International Journal of Advanced Computer Science and Applications, vol. 15, no. 4, pp. 627–636, 2024, doi: 10.14569/IJACSA.2024.0150464.
N. A. Mohammed, O. F. Abdulateef, and A. H. Hamad, “An IoT and Machine Learning-Based Predictive Maintenance System for Electrical Motors,” Journal Europeen des Systemes Automatises, vol. 56, no. 4, pp. 651–656, Sep. 2023, doi: 10.18280/jesa.560414.
K. Velmurugan, P. Venkumar, and P. R. Sudhakara, “SME 4.0: Machine learning framework for real-time machine health monitoring system,” in Journal of Physics: Conference Series, IOP Publishing Ltd, Jun. 2021. doi: 10.1088/1742-6596/1911/1/012026.
D. Navakauskas, Vilniaus Gedimino technikos universitetas, Institute of Electrical and Electronics Engineers. Lithuania Section, and Institute of Electrical and Electronics Engineers, “Machine Learning Application in Predictive Maintenance,” in IEEE Open Conference of Electrical, Electronic and Information Sciences (eStream), 2019.
S. Márquez Sánchez, “Integral Support Predictive Platform for Industry 4.0,” ADCAIJ: Advances in Distributed Computing and Artificial Intelligence Journal, vol. 9, no. 4, pp. 71–82, Dec. 2020, doi: 10.14201/adcaij2020947182.
M. Cakir, M. A. Guvenc, and S. Mistikoglu, “The experimental application of popular machine learning algorithms on predictive maintenance and the design of IIoT based condition monitoring system,” Comput Ind Eng, vol. 151, Jan. 2021, doi: 10.1016/j.cie.2020.106948.
M. Fikar, M. Kvasnica, S. F. chemickej a potravinárskej technológie. I. of I. E. Slovenská technická univerzita (Bratislava, IEEE Control Systems Society, IEEE Czechoslovakia Section, and Institute of Electrical and Electronics Engineers, “Intelligent predictive maintenance control using augmented reality,” Proceedings of the 2019 22nd International Conference on Process Control (PC19) : IEEE, 2019, pp. 131–135.
A. Angelopoulos et al., “Tackling faults in the industry 4.0 era—a survey of machine-learning solutions and key aspects,” Sensors (Switzerland), vol. 20, no. 1. MDPI AG, Jan. 01, 2020. doi: 10.3390/s20010109.
P. Strauß, M. Schmitz, R. Wöstmann, and J. Deuse, “Enabling of Predictive Maintenance in the Brownfield through Low-Cost Sensors, an IIoT-Architecture and Machine Learning,” in Proceedings - 2018 IEEE International Conference on Big Data, Big Data 2018, Institute of Electrical and Electronics Engineers Inc., Jan. 2019, pp. 1474–1483. doi: 10.1109/BigData.2018.8622076.
R. De Luca, A. Ferraro, A. Galli, M. Gallo, V. Moscato, and G. Sperlì, “A deep attention based approach for predictive maintenance applications in IoT scenarios,” Journal of Manufacturing Technology Management, vol. 34, no. 4, pp. 535–556, May 2023, doi: 10.1108/JMTM-02-2022-0093.
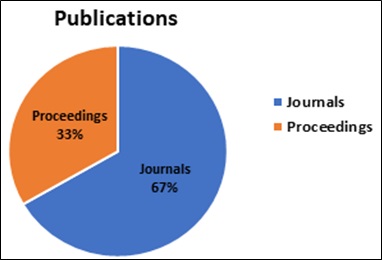
Downloads
Published
How to Cite
Issue
Section
License
Copyright (c) 2024 50sea

This work is licensed under a Creative Commons Attribution 4.0 International License.