Bio fusion: Advancing Biometric Authentication by Fusion of Physiological Signals
Keywords:
Person Identification, Biometric Authentication , Machine Learning, Physiological Signals, MEL Frequency Cepstral CoefficientAbstract
Biometric authentication is becoming more popular due to its secure and reliable way of identifying individuals, offering clear advantages over traditional methods. Since physiological signals are unique and non-invasive, they have been widely researched for use in biometric systems. This study introduces a biometric identification system that combines machine learning with physiological signal fusion, using data from electromyography (EMG), phonocardiogram (PCG), and electrocardiogram (ECG). The data were collected from 32 participants using the BIOPAC MP-36 system. To remove power line interference and extract important frequency bands, Butterworth notch, and bandpass filters were applied to the raw signals. After pre-processing, two types of cepstral features were extracted: gamma tone cepstral coefficients (GTCCs) and Mel-frequency cepstral coefficients (MFCCs), which were analysed for their spectral properties. System performance was first tested by evaluating features from each signal individually. Then, the study examined the impact of combining pairs of signals— (ECG, PCG), (PCG, EMG), and (ECG, EMG)—using GTCC and MFCC features with different machine learning classifiers. Lastly, the GTCC and MFCC features from all three signals were combined to evaluate overall system performance. The results showed that MFCC-based features performed better than GTCC-based features for biometric authentication. The highest accuracy, 98.4%, was achieved using GTCC features with both the Fine K-nearest neighbour (KNN) and linear discriminant classifiers, while MFCC features reached 100% accuracy with the linear discriminant classifier. These findings highlight how effective cepstral features and signal fusion can be in enhancing biometric authentication performance.
References
A. R. Anil K. Jain, Karthik Nandakumar, “50 years of biometric research: Accomplishments, challenges, and opportunities,” Pattern Recognit. Lett., vol. 79, pp. 80–105, 2016, doi: https://doi.org/10.1016/j.patrec.2015.12.013.
K. Y. Moon, “Biometrics technology status and prospects,” Internet Serv. Inf. Secur., vol. 98, pp. 38–47, 2005.
P. Mi et al., “Driver Cognitive Architecture Based on EEG Signals: A Review,” IEEE Sens. J., 2024, doi: 10.1109/JSEN.2024.3471699.
A. N. Uwaechia and D. A. Ramli, “A Comprehensive Survey on ECG Signals as New Biometric Modality for Human Authentication: Recent Advances and Future Challenges,” IEEE Access, vol. 9, pp. 97760–97802, 2021, doi: 10.1109/ACCESS.2021.3095248.
S. S. Roberta Avanzato, Francesco Beritelli, “Robust Biometric Verification Using Phonocardiogram Fingerprinting and a Multilayer-Perceptron-Based Classifier,” Electronics, vol. 13, no. 22, p. 4377, 2024, doi: https://doi.org/10.3390/electronics13224377.
S. B. P. Jin Su Kim, “A Study on EMG-based Biometrics,” Journal of Internet Services and Information Security. Accessed: Mar. 17, 2025. [Online]. Available: https://jisis.org/article/jisis-2017-vol7-no2-02/69704/
Giwon Ku, Choeljun Choi, Chulseung Yang, Jiseong Jeong , Pilkyo Kim, Sangyong Park, “Electrocardiogram-Based Driver Authentication Using Autocorrelation and Convolutional Neural Network Techniques,” Electronics, vol. 13, no. 24, p. 4974, 2024, doi: https://doi.org/10.3390/electronics13244974.
C. Lipps, LeaBergkemper, J. Herbst, and H. D. Schotten, “I Know You by Heart: Biometric Authentication based on Electrocardiogram (ECG) signals,” Int. Conf. Cyber Warf. Secur., vol. 17, no. 1, 2022, doi: https://doi.org/10.34190/iccws.17.1.12.
M. Asim, M. Akhtar, M. Faraz, M. U. Khan, S. Aziz, and G. A. Montes, “Pattern Analysis for Biometric Authentication using Electrocardiogram Signal,” 2023 2nd Int. Conf. Emerg. Trends Electr. Control. Telecommun. Eng. ETECTE 2023 - Proc., 2023, doi: 10.1109/ETECTE59617.2023.10396767.
H. Gurkan, U. Guz, and B. S. Yarman, “A novel biometric authentication approach using electrocardiogram signals,” Proc. Annu. Int. Conf. IEEE Eng. Med. Biol. Soc. EMBS, pp. 4259–4262, 2013, doi: 10.1109/EMBC.2013.6610486.
M. U. Khan, S. Aziz, A. Zainab, H. Tanveer, K. Iqtidar, and A. Waseem, “Biometric System using PCG Signal Analysis: A New Method of Person Identification,” 2nd Int. Conf. Electr. Commun. Comput. Eng. ICECCE 2020, Jun. 2020, doi: 10.1109/ICECCE49384.2020.9179257.
G. Gautam and D. Kumar, “Biometric system from heart sound using wavelet based feature set,” Int. Conf. Commun. Signal Process. ICCSP 2013 - Proc., pp. 551–555, 2013, doi: 10.1109/ICCSP.2013.6577115.
M. Abo-Zahhad, S. M. Ahmed, and S. N. Abbas, “A new biometric authentication system using heart sounds based on wavelet packet features,” Proc. IEEE Int. Conf. Electron. Circuits, Syst., vol. 2016-March, pp. 17–20, Mar. 2016, doi: 10.1109/ICECS.2015.7440238.
M. U. Khan, Z. A. Choudry, S. Aziz, S. Z. H. Naqvi, A. Aymin, and M. A. Imtiaz, “Biometric Authentication based on EMG Signals of Speech,” 2nd Int. Conf. Electr. Commun. Comput. Eng. ICECCE 2020, Jun. 2020, doi: 10.1109/ICECCE49384.2020.9179354.
L. Lu, J. Mao, W. Wang, G. Ding, and Z. Zhang, “A Study of Personal Recognition Method Based on EMG Signal,” IEEE Trans. Biomed. Circuits Syst., vol. 14, no. 4, pp. 681–691, Aug. 2020, doi: 10.1109/TBCAS.2020.3005148.
A. Mehmood, A. Hannan, M. U. Khan, S. Z. H. Naqvi, M. Faraz, and S. Aziz, “Biofusion: Expanding Biometric Horizons with ECG and PCG Integration,” 2023 Int. Conf. Digit. Futur. Transform. Technol. ICoDT2 2023, 2023, doi: 10.1109/ICODT259378.2023.10325791.
N. A. I. M. Rosli, M. A. A. Rahman, S. A. Mazlan, and H. Zamzuri, “Electrocardiographic (ECG) and Electromyographic (EMG) signals fusion for physiological device in rehab application,” 2014 IEEE Student Conf. Res. Dev. SCOReD 2014, Mar. 2014, doi: 10.1109/SCORED.2014.7072965.
L. Y. Qun Ma, Yibo Wu, Muhammad Usman Shoukat, Yukai Yan, Jun Wang, Long Yang, Fuwu Yan, “Deep Reinforcement Learning-Based Wind Disturbance Rejection Control Strategy for UAV,” Drones, vol. 8, no. 11, p. 632, 2024, doi: https://doi.org/10.3390/drones8110632.
T. K. Montree Kumngern, Nattharinee Aupithak, Fabian Khateb, “0.5 V Fifth-Order Butterworth Low-Pass Filter Using Multiple-Input OTA for ECG Applications,” Sensors, vol. 20, no. 24, p. 7343, 2020, doi: https://doi.org/10.3390/s20247343.
S. Jayalakshmy and G. F. Sudha, “GTCC-based BiLSTM deep-learning framework for respiratory sound classification using empirical mode decomposition,” Neural Comput. Appl., vol. 33, no. 24, pp. 17029–17040, Dec. 2021, doi: 10.1007/S00521-021-06295-X/METRICS.
S. A. A. Yusuf and R. Hidayat, “MFCC feature extraction and KNN classification in ECG signals,” 2019 6th Int. Conf. Inf. Technol. Comput. Electr. Eng. ICITACEE 2019, Sep. 2019, doi: 10.1109/ICITACEE.2019.8904285.
I. Fahad, M. A. R. Apu, A. Ghosh, and S. A. Fattah, “Phonocardiogram Heartbeat Segmentation and Autoregressive Modeling for Person Identification,” IEEE Reg. 10 Annu. Int. Conf. Proceedings/TENCON, vol. 2019-October, pp. 1942–1946, Oct. 2019, doi: 10.1109/TENCON.2019.8929563.
C. S. Xiefeng Cheng, Pengfei Wang, “Biometric Identification Method for Heart Sound Based on Multimodal Multiscale Dispersion Entropy,” Entropy, vol. 22, no. 2, p. 238, 2020, doi: https://doi.org/10.3390/e22020238.
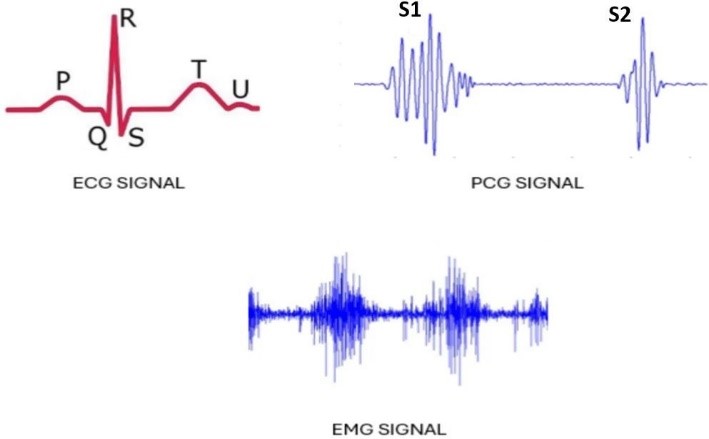
Downloads
Published
How to Cite
Issue
Section
License
Copyright (c) 2025 50SEA

This work is licensed under a Creative Commons Attribution 4.0 International License.