A Comparative Analysis of Camera, LiDAR and Fusion Based Deep Neural Networks for Vehicle Detection
Keywords:
sensor fusion, object detection, 3D object detection, LiDAR point cloud, self-driving cars.Abstract
Self-driving cars are an active area of interdisciplinary research spanning Artificial Intelligence (AI), Internet of Things (IoT), embedded systems, and control engineering. One crucial component needed in ensuring autonomous navigation is to accurately detect vehicles, pedestrians, or other obstacles on the road and ascertain their distance from the self-driving vehicle. The primary algorithms employed for this purpose involve the use of cameras and Light Detection and Ranging (LiDAR) data. Another category of algorithms consists of a fusion between these two sensor data. Sensor fusion networks take input as 2D camera images and LiDAR point clouds to output 3D bounding boxes as detection results. In this paper, we experimentally evaluate the performance of three object detection methods based on the input data type. We offer a comparison of three object detection networks by considering the following metrics - accuracy, performance in occluded environment, and computational complexity. YOLOv3, BEV network, and Point Fusion were trained and tested on the KITTI benchmark dataset. The performance of a sensor fusion network was shown to be superior to single-input networks.
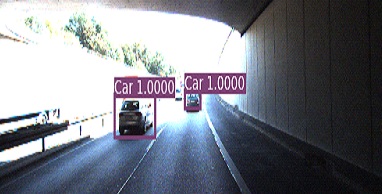
Published
How to Cite
Issue
Section
License
Copyright (c) 2022 50Sea

This work is licensed under a Creative Commons Attribution 4.0 International License.