Knowledge Acquisition System for Sentiment Analysis
Keywords:
Expert System, Knowledge Acquisition, Sentimental Analysis, Opinion Mining, Common-SenseAbstract
Human has aptitude to understand the knowledge from different life circumstances, experiences for acquire from these experiences and use itself for the erudition of Common Sense acquaintance for making the imperative conclusions in life. That is principal alteration between Out-dated Artificial Intelligence (AI), Expert Systems (ES) and Humans. Due to this capability, human could directly handle conclusion circumstances but in systems they have deficiency of generality, rules state of mind and Sentiments skills. Human circumstantial experiences communicate about how to live in social cultures where the common-sense knowledge is very vigorous. Subsequently Opinion Mining become very substantial method in now days. Two core categories for the system how to grow Sentimental Knowledge Acquisitions with the help of these two. Analyses around diverse methods used for Opinion Mining and forthcoming work might be ensue in approaching years for Sentimental Knowledge Acquisition.
References
P. Chauhan, N. Sharma, and G. Sikka, “The emergence of social media data and sentiment analysis in election prediction,” J. Ambient Intell. Humaniz. Comput., vol. 12, no. 2, pp. 2601–2627, 2021, doi: 10.1007/s12652-020-02423-y.
Y. Lin, X. Wang, and A. Zhou, “Opinion spam detection,” Opin. Anal. Online Rev., no. May, pp. 79–94, 2016, doi: 10.1142/9789813100459_0007.
Erik Cambria, D. Olsher, D. Rajagopal, and E. Cambria, “Proceedings of AAAI,” SenticNet 3 A common common-sense Knowl. base Cogn. Sentim. Anal., pp. 1515–1521, 2014.
E. Cambria, “Affective Computing and Sentiment Analysis,” IEEE Intell. Syst., vol. 31, no. 2, pp. 102–107, Mar. 2016, doi: 10.1109/MIS.2016.31.
G. B. Fischer, “Pneumocystis carinii, Aspergillus fumigatus ) •,” Empir. Methods Nat. Lang. Process., no. October, pp. 1631–1642, 2004.
H. Yu and V. Hatzivassiloglou, “Towards answering opinion questions,” pp. 129–136, 2003, doi: 10.3115/1119355.1119372.
B. Pang, L. Lee, and S. Vaithyanathan, “Thumbs up? Sentiment Classification using Machine Learning Techniques,” pp. 79–86, 2002, doi: 10.3115/1118693.1118704.
T. Durmus, J. Stöckel, T. Slowinski, A. Thomas, and T. Fischer, “The hyperechoic zone around breast lesions - An indirect parameter of malignancy,” Ultraschall der Medizin, vol. 35, no. 6, pp. 547–553, 2014, doi: 10.1055/s-0034-1385342.
D. Tang, F. Wei, B. Qin, T. Liu, and M. Zhou, “Coooolll: A Deep Learning System for Twitter Sentiment Classification,” 8th Int. Work. Semant. Eval. SemEval 2014 - co-located with 25th Int. Conf. Comput. Linguist. COLING 2014, Proc., no. SemEval, pp. 208–212, 2014, doi: 10.3115/v1/s14-2033.
D. Tang, F. Wei, N. Yang, M. Zhou, T. Liu, and B. Qin, “Learning Sentiment-Specific Word Embedding,” Acl, pp. 1555–1565, 2014.
S. Poria, E. Cambria, N. Howard, G. Bin Huang, and A. Hussain, “Fusing audio, visual and textual clues for sentiment analysis from multimodal content,” Neurocomputing, vol. 174, pp. 50–59, Jan. 2016, doi: 10.1016/J.NEUCOM.2015.01.095.
C. N. Dos Santos and M. Gatti, “Deep convolutional neural networks for sentiment analysis of short texts,” COLING 2014 - 25th Int. Conf. Comput. Linguist. Proc. COLING 2014 Tech. Pap., pp. 69–78, 2014.
S. Poria, E. Cambria, and A. Gelbukh, “Aspect extraction for opinion mining with a deep convolutional neural network,” Knowledge-Based Syst., vol. 108, pp. 42–49, Sep. 2016, doi: 10.1016/J.KNOSYS.2016.06.009.
S. Poria, E. Cambria, A. Gelbukh, F. Bisio, and A. Hussain, “Sentiment Data Flow Analysis by Means of Dynamic Linguistic Patterns,” IEEE Comput. Intell. Mag., vol. 10, no. 4, pp. 26–36, Nov. 2015, doi: 10.1109/MCI.2015.2471215.
A. Casey et al., “A systematic review of natural language processing applied to radiology reports,” BMC Med. Informatics Decis. Mak. 2021 211, vol. 21, no. 1, pp. 1–18, Jun. 2021, doi: 10.1186/S12911-021-01533-7.
K. Guo, L. Shi, W. Ye, and X. Li, “A survey of Internet public opinion mining,” PIC 2014 - Proc. 2014 IEEE Int. Conf. Prog. Informatics Comput., pp. 173–179, Dec. 2014, doi: 10.1109/PIC.2014.6972319.
H. Liu and P. Singh, “Commonsense reasoning in and over natural language,” Lect. Notes Comput. Sci. (including Subser. Lect. Notes Artif. Intell. Lect. Notes Bioinformatics), vol. 3215, pp. 293–306, 2004, doi: 10.1007/978-3-540-30134-9_40.
M. A. Arshed, S. Mumtaz, M. S. Liaqat, and I. Haq, “LSTM Based Sentiment Analysis Model to Monitor COVID-19 Emotion LSTM Based Sentiment Analysis Model to Monitor COVID-19 Emotion,” no. May, 2022.
M. A. Arshed, S. Mumtaz, O. Riaz, W. Sharif, and S. Abdullah, “A Deep Learning Framework for Multi-Drug Side Effects Prediction with Drug Chemical Substructure,” Int. J. Innov. Sci. Technol., vol. 4, no. 1, pp. 19–31, 2022.
M. T. Ubaid, A. Kiran, M. T. Raja, U. A. Asim, A. Darboe, and M. A. Arshed, “Automatic Helmet Detection using EfficientDet,” 4th Int. Conf. Innov. Comput. ICIC 2021, 2021, doi: 10.1109/ICIC53490.2021.9693093.
B. Liu et al., “Comparison of Machine Learning Classifiers for Breast Cancer Diagnosis Based on Feature Selection,” Proc. - 2018 IEEE Int. Conf. Syst. Man, Cybern. SMC 2018, pp. 4399–4404, Jan. 2019, doi: 10.1109/SMC.2018.00743.
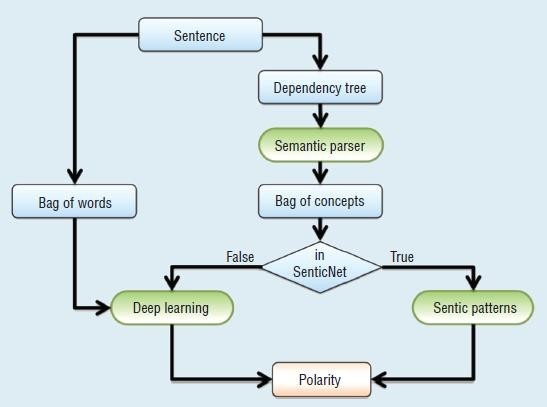
Published
How to Cite
Issue
Section
License
Copyright (c) 2022 50sea

This work is licensed under a Creative Commons Attribution 4.0 International License.