Addressing Illicit Tobacco Growth in Pakistan: Leveraging AI and Satellite Technology for Precise Monitoring and Effective Solutions
Keywords:
Tobacco, Artificial Intelligence, Deep Learning, Recurrent Neural Networks, Remote Sensing, Sentinel-2, Planet-ScopeAbstract
The market share of illicit tobacco products in Pakistan has seen a significant surge in recent years. In 2022, it reached a staggering 42.5%. Since January 2023, there has been a sharp 32.5% increase in volumes of Duty Not Paid (DNP) products and a remarkable 67% surge in the quantities of smuggled cigarettes. This rise can be attributed to the unregistered and unlicensed tobacco cultivation in Pakistan. This sector has largely relied on conventional methods for data collection in the field, primarily managed by the country's crop statistical departments. The utilization of cutting-edge artificial intelligence techniques and satellite imagery for generating crop statistics has the potential to address this issue effectively. We established a synergy by combining images from two remote sensing satellites and collected field data to detect tobacco crops using Recurrent Neural Networks (RNN). The results affirm the effectiveness of these techniques in detecting and estimating the acreage of tobacco crops in the observed areas, particularly in a union council of the Swabi region. We conducted surveys to collect training and validation data through our proprietary smartphone application, GeoSurvey. The collected data was subsequently refined, preprocessed, and organized to prepare it for use with our deep learning algorithm. The model we developed for the detection and acreage estimation of tobacco crops is called Convolutional Long Short-Term Memory (ConvLSTM). We created two datasets from the acquired satellite images for comparison. Our experimentation results demonstrated that the use of ConvLSTM for the synergy of Sentinel-2 and Planet-Scope imagery yields higher training and validation accuracy, reaching 98.09% and 96.22%, respectively. In comparison, the use of time series Sentinel-2 images alone achieved training and testing accuracy of 97.78% and 95.56%.
References
R. T. Y. and R. Khalid, “Impact of tobacco generated income on Pakistan economy (a case study of khyber Pakhtunkhwa),” J. Basic Appl. Sci. Res., vol. 7, no. 4, pp. 1–17, 2017.
and M. A. I. M. Sabir, W. Saleem, “Estimating the Under-Reporting of Cigarette Production in Pakistan,” 2022.
W. K. et Al, “On the performance of temporal stacking and vegetation indices for detection and estimation of tobacco crop,” IEEE Access, vol. 8, pp. 103020–103033, 2020.
and H. A. J. C. Antenucci, K. Brown, P. L. Croswell, M. J. Kevany, “Geographic Information Systems: a guide to the technology,” Springer, vol. 115, 1991.
and P. J. P. Shanmugapriya, S. Rathika, T. Ramesh, “Applications of remote sensing in agriculture-A Review,” Int. J. Curr. Microbiol. Appl. Sci, vol. 8, no. 1, pp. 2270–2283, 2019.
V. Klemas, “Fisheries applications of remote sensing: An overview,” Fish. Res., vol. 148, pp. 124–136, 2013.
and G. A. D. Poli, F. Remondino, E. Angiuli, “Radiometric and geometric evaluation of GeoEye-1, World View-2 and Pléiades-1A stereo images for 3D information extraction,” ISPRS J. Photogramm. Remote Sens., pp. 35–47, 2015.
M.-C. C. and C. Zhang, ““Formosat-2 for international societal benefits,” Remote Sens., pp. 1–7, 2016.
M. Drusch et al., “Sentinel-2: ESA’s Optical High-Resolution Mission for GMES Operational Services,” Remote Sens. Environ., vol. 120, pp. 25–36, May 2012, doi: 10.1016/J.RSE.2011.11.026.
C. San Francisco, “Planet application program interface: In space for life on Earth,” Team, P., 2017.
C. Liao et al, “Synergistic use of multi-temporal RADARSAT-2 and VENμS data for crop classification based on 1D convolutional neural network,” Remote Sens., vol. 12, no. 5, 2020.
and Y. H. J. Zhang, Y. He, L. Yuan, P. Liu, X. Zhou, “Machine learning-based spectral library for crop classification and status monitoring,” Agronomy, vol. 9, no. 9, 2019.
G. B. and E. Scornet, “A random forest guided tour,” vol. 25, pp. 197–227, 2016.
C.-C. C. and C.-J. Lin, “LIBSVM: a library for support vector machines,” ACM Trans. Intell. Syst. Technol., vol. 2, no. 3, pp. 1–27, 2011.
and H. K. X. Sun, J. Peng, Y. Shen, “Tobacco plant detection in RGB aerial images,” Agriculture, vol. 10, no. 3, 2020.
and M. S.-S. Y. Palchowdhuri, R. Valcarce-Diñeiro, P. King, “Classification of multi-temporal spectral indices for crop type mapping: a case study in Coalville, UK,” J. Agric. Sci., vol. 156, no. 1, pp. 24–36, 2018.
and X. L. D. Li, Y. Ke, H. Gong, “Object-based urban tree species classification using bi-temporal WorldView-2 and WorldView-3 images,” Remote Sens., vol. 7, no. 12, pp. 16917–16937, 2015.
J. R. Jensen, “Biophysical remote sensing,” Ann. Assoc. Am. Geogr., vol. 73, no. 1, pp. 111–132, 1983.
L. P. V. et Al., “Forecasting corn yield at the farm level in Brazil based on the FAO-66 approach and soil-adjusted vegetation index (SAVI),” Agric. Water Manag., vol. 225, 2019.
and V. D. A. K. Verma, P. K. Garg, K. Hari Prasad, “CLASSIFICATION OF LISS IV IMAGERY USING DECISION TREE METHODS.,”,” Int. Arch. Photogramm. Remote Sens. Spat. Inf. Sci., vol. 41, 2016.
and E. D. G. Z. Fan, J. Lu, M. Gong, H. Xie, “Automatic tobacco plant detection in UAV images via deep neural networks,” IEEE J. Sel. Top. Appl. Earth Obs. Remote Sens., vol. 11, no. 3, pp. 876–887, 2018.
Y. B. Y. LeCun, “Convolutional networks for images, speech, and time series,” Handb. brain theory neural networks, vol. 3361, no. 10, 1995.
and F. H. L. Wang, J. Zhang, P. Liu, K.-K. R. Choo, “Spectral–spatial multi-feature-based deep learning for hyperspectral remote sensing image classification,” Soft Comput., vol. 21, pp. 213–221, 2017.
X. Z. et Al., “Deep learning in remote sensing: a review december,” 2017.
and A. S. N. Kussul, M. Lavreniuk, S. Skakun, “Deep learning classification of land cover and crop types using remote sensing data,” IEEE Geosci. Remote Sens. Lett., vol. 14, no. 5, pp. 778–782, 2017.
and Y. D. S. Ji, C. Zhang, A. Xu, Y. Shi, “3D convolutional neural networks for crop classification with multi-temporal remote sensing images,” Remote Sens., vol. 10, no. 1, 2018.
S. Hochreiter and J. Schmidhuber, “Long Short-Term Memory,” Neural Comput., vol. 9, no. 8, pp. 1735–1780, Nov. 1997, doi: 10.1162/NECO.1997.9.8.1735.
and A. M. A. Graves, N. Jaitly, “Hybrid speech recognition with deep bidirectional LSTM,” 2013 IEEE Work. Autom. speech Recognit. understanding, IEEE, pp. 273–278, 2013.
L. M. and L. C. Jain, “Recurrent neural networks: design and applications.,” CRC Press, 1999.
M. Boden, “A guide to recurrent neural networks and backpropagation,” Dallas Proj., 2002.
and F. S. T. M. Breuel, A. Ul-Hasan, M. A. Al-Azawi, “High-performance OCR for printed English and Fraktur using LSTM networks,” 2013 12th Int. Conf. Doc. Anal. recognition, IEEE, pp. 683–687, 2013.
and J. Y. Z. Ding, R. Xia, J. Yu, X. Li, “Densely connected bidirectional lstm with applications to sentence classification,” CCF Int. Conf. Nat. Lang. Process. Chinese Comput. Springer, pp. 278–287, 2018.
X. Z. Y. Chen, K. Zhong, J. Zhang, Q. Sun, “LSTM networks for mobile human activity recognition,” Proc. 2016 Int. Conf. Artif. Intell. Technol. Appl. Bangkok, Thail., pp. 24–25, 2016.
S. K. and R. Casper, “Applications of convolution in image processing with MATLAB,” Univ. Washingt., pp. 1–20, 2013.
and J. Z. Z. Li, F. Liu, W. Yang, S. Peng, “A survey of convolutional neural networks: analysis, applications, and prospects,” IEEE Trans. neural networks Learn. Syst., 2021.
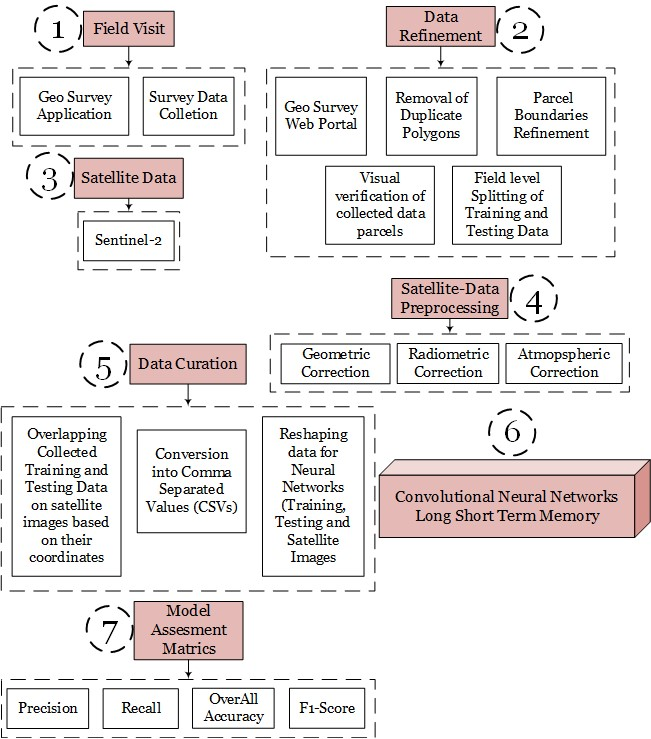
Published
How to Cite
Issue
Section
License
Copyright (c) 2023 50SEA

This work is licensed under a Creative Commons Attribution 4.0 International License.