Synergizing Digital Twin Technology for Advanced Depression Categorization in Social-Media through Data Mining Analysis
Keywords:
Digital twin technology, Data Mining, Sentiment Analysis, Social Media, Depression, Categorization.Abstract
The progression from negative emotions to depression is a significant concern, marked by persistent sadness and an inability to cope with challenging circumstances. Regrettably, it can lead to the extreme step of suicide. According to the World Health Organization (WHO), 4.4% of the global population currently grapples with depression. Shockingly, 700,000 individuals worldwide took their own lives in 2023, and this tragic number continues to escalate. Our objective is to detect signs of depression in individuals through their social media posts, SMS, or comments. We collected nearly 10,000 pieces of information from Twitter comments, Facebook posts, and remarks. Employing data mining and machine learning algorithms has proven instrumental in swiftly discerning individuals' emotional states. To predict depression versus non-depression, we employed six classifiers, with support vector machines (SVMs) demonstrating the highest accuracy. A comparison between SVM and Naïve Bayes revealed that Naïve Bayes yielded superior results in our study.
References
M.-Y. Fan et al., “Acupoints compatibility rules of acupuncture for depression disease based on data mining technology,” Zhongguo Zhen Jiu, vol. 43, no. 3, pp. 269–76, 2023, doi: 10.13703/J.0255-2930.20221103-K0001.
D. Xue, Y. Zhang, Z. Song, X. Jie, R. Jia, and A. Zhu, “Integrated meta-analysis, data mining, and animal experiments to investigate the efficacy and potential pharmacological mechanism of a TCM tonic prescription, Jianpi Tongmai formula, in depression,” Phytomedicine, vol. 105, p. 154344, Oct. 2022, doi: 10.1016/J.PHYMED.2022.154344.
“Meme Detection of Journalists from Social Media by Using Data Mining Techniques | International Journal of Innovations in Science & Technology.” Accessed: Nov. 26, 2023. [Online]. Available: https://journal.50sea.com/index.php/IJIST/article/view/404
F. Alhussain, A. Bin Onayq, D. Ismail, M. Alduayj, T. Alawbathani, and M. Aljaffer, “Adjustment disorder among first year medical students at King Saud University, Riyadh, Saudi Arabia, in 2020,” J. Fam. Community Med., vol. 30, no. 1, p. 59, 2023, doi: 10.4103/JFCM.JFCM_227_22.
Ö. Baltacı, “The Predictive Relationships between the Social Media Addiction and Social Anxiety, Loneliness, and Happiness,” Int. J. Progress. Educ., vol. 15, no. 4, pp. 73–82, Aug. 2019, doi: 10.29329/IJPE.2019.203.6.
W. Lu et al., “Differences in cognitive functions of atypical and non-atypical depression based on propensity score matching,” J. Affect. Disord., vol. 325, pp. 732–738, Mar. 2023, doi: 10.1016/J.JAD.2023.01.071.
M. P. Valerio, J. Lomastro, A. Igoa, and D. J. Martino, “Clinical Characteristics of Melancholic and Nonmelancholic Depressions,” J. Nerv. Ment. Dis., vol. 211, no. 3, pp. 248–252, Mar. 2023, doi: 10.1097/NMD.0000000000001616.
F. Tasnim, S. U. Habiba, N. Nafisa, and A. Ahmed, “Depressive Bangla Text Detection from Social Media Post Using Different Data Mining Techniques,” Lect. Notes Electr. Eng., vol. 834, pp. 237–247, 2022, doi: 10.1007/978-981-16-8484-5_21/COVER.
M. Maes and A. F. Almulla, “Research and Diagnostic Algorithmic Rules (RADAR) and RADAR Plots for the First Episode of Major Depressive Disorder: Effects of Childhood and Recent Adverse Experiences on Suicidal Behaviors, Neurocognition and Phenome Features,” Brain Sci. 2023, Vol. 13, Page 714, vol. 13, no. 5, p. 714, Apr. 2023, doi: 10.3390/BRAINSCI13050714.
C. S. Wu, C. J. Kuo, C. H. Su, S. H. Wang, and H. J. Dai, “Using text mining to extract depressive symptoms and to validate the diagnosis of major depressive disorder from electronic health records,” J. Affect. Disord., vol. 260, pp. 617–623, Jan. 2020, doi: 10.1016/J.JAD.2019.09.044.
R. Vanlalawmpuia and M. Lalhmingliana, “Prediction of Depression in Social Network Sites Using Data Mining,” Proc. Int. Conf. Intell. Comput. Control Syst. ICICCS 2020, pp. 489–495, May 2020, doi: 10.1109/ICICCS48265.2020.9120899.
C. K. Ettman, S. M. Abdalla, G. H. Cohen, L. Sampson, P. M. Vivier, and S. Galea, “Prevalence of Depression Symptoms in US Adults Before and During the COVID-19 Pandemic,” JAMA Netw. Open, vol. 3, no. 9, pp. e2019686–e2019686, Sep. 2020, doi: 10.1001/JAMANETWORKOPEN.2020.19686.
L. Smith et al., “Association between depression and subjective cognitive complaints in 47 low- and middle-income countries,” J. Psychiatr. Res., vol. 154, pp. 28–34, Oct. 2022, doi: 10.1016/J.JPSYCHIRES.2022.07.021.
S. L. Dubovsky, B. M. Ghosh, J. C. Serotte, and V. Cranwell, “Psychotic Depression: Diagnosis, Differential Diagnosis, and Treatment,” Psychother. Psychosom., vol. 90, no. 3, pp. 160–177, Apr. 2021, doi: 10.1159/000511348.
Ö. DEMİRCİ and E. INAN, “Postpartum Paternal Depression: Its Impact on Family and Child Development,” Curr. Approaches Psychiatry, vol. 15, no. 3, pp. 498–507, Sep. 2023, doi: 10.18863/PGY.1153712.
L. Sforzini et al., “A Delphi-method-based consensus guideline for definition of treatment-resistant depression for clinical trials,” Mol. Psychiatry 2021 273, vol. 27, no. 3, pp. 1286–1299, Dec. 2021, doi: 10.1038/s41380-021-01381-x.
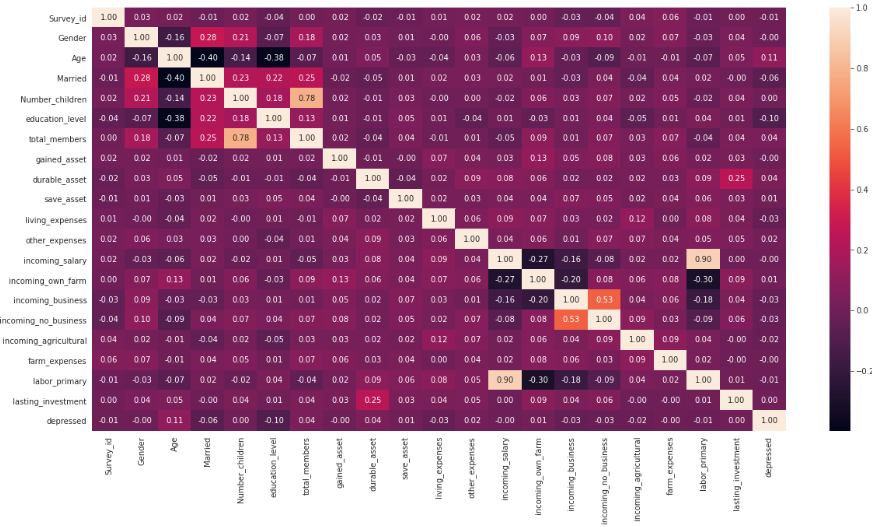
Downloads
Published
How to Cite
Issue
Section
License
Copyright (c) 2024 50SEA

This work is licensed under a Creative Commons Attribution 4.0 International License.