Prediction of Political Instability by Using Pre-Trained Neural Networks
Keywords:
Machine Learning, Artificial Neural Network, Big Data, BD, Big Data challenges, Big data tools and techniques, Analysis of bigdata., Decision Support, ANN forecastingAbstract
This research aims to enhance and optimise the decision-making process in the political science domain by exploring the potential of machine learning. The aim was to create a pre-trained neural network to predict the political instability in any country (a prediction that aids decision-makers in handling government affairs and crisis prevention). We constructed four pre-trained neural networks, each tailored to a specific indicator (Human Development Index, Currency Strength Index, Tax to GDP Ratio, and Fragile States Index). These indicators are selected based on their strong correlation and how their concurrent performance impacts the political landscape of any country. The neural networks exhibited exceptional performance, achieving accuracy rates above 85%. The model built on the FSI demonstrated an astonishing accuracy of 99.67%, underscoring its potential for comprehensive assessments. The prospect envisions amalgamating the outputs of these pre-trained neural networks into a unified, deep-learning network, poised to yield collective decisions and recommend policy initiatives.
References
G. T. Dalyop, “Political instability and economic growth in Africa,” Int. J. Econ. Policy Stud., vol. 13, no. 1, pp. 217–257, Jan. 2019, doi: 10.1007/S42495-018-0008-1/METRICS.
H. Kull, M., Kalkbrenner, G., & Runhaar, “A machine learning approach to policy recommendation for sustainable energy policies,” Energy Policy, 2020.
N. Chen, J., Gleditsch, K. S., & Weidmann, “Predicting conflict in sub-Saharan Africa with machine learning,” J. Peace Res., vol. 56, no. 4, pp. 557–572, 2019.
E. Baillie, P. D. L. Howe, A. Perfors, T. Miller, Y. Kashima, and A. Beger, “Explainable models for forecasting the emergence of political instability,” PLoS One, vol. 16, no. 7, p. e0254350, Jul. 2021, doi: 10.1371/JOURNAL.PONE.0254350.
et al Jahani, Elnaz, “Predicting Political Instability: Using Machine Learning for Conflict Forecasting,” Journal/Conference, 2022.
C. et al. Wang, “Enhancing Decision-making in Political Science Using Neural Network Fusion,” Journal/Conference, 2023.
V. D’Orazio and Y. Lin, “Forecasting conflict in Africa with automated machine learning systems,” Int. Interact., vol. 48, no. 4, pp. 714–738, 2022, doi: 10.1080/03050629.2022.2017290.
E. Sørensen and C. Ansell, “Towards a Concept of Political Robustness,” https://doi.org/10.1177/0032321721999974, vol. 71, no. 1, pp. 69–88, Apr. 2021, doi: 10.1177/0032321721999974.
R. Ouedraogo, M. Mlachila, W. S. Sourouema, and A. Compaoré, “The impact of conflict and political instability on banking crises in developing countries,” World Econ., vol. 45, no. 6, pp. 1937–1977, Jun. 2022, doi: 10.1111/TWEC.13218.
“IMF Working paper.” Accessed: Dec. 18, 2023. [Online]. Available: https://www.elibrary.imf.org/subject/COM000000?pageSize=10&sort=datedescending&t=P16
X. Chen, Y., Wang, X., Wang, J., Li, M., Li, Y., & Li, “A Deep Learning Approach for Political Stability Prediction,” Access, IEEE, vol. 7, pp. 174930–174941, 2019.
L. Bourahla, N., Dahou, A., & Oukhellou, “Forecasting social unrest: A machine learning approach,” Chaos, Solitons & Fractals, vol. 119, pp. 117–126, 2019.
Y. Chen, Y., Li, X., & Li, “Explainable models for forecasting the emergence of political instability,” PLoS One, vol. 16, no. 4, 2021.
J. An, A. Mikhaylov, and N. Moiseev, “Oil price predictors: Machine learning approach,” Int. J. Energy Econ. Policy, vol. 9, no. 5, pp. 1–6, 2019, doi: 10.32479/IJEEP.7597.
M. S. M. Lima and D. Delen, “Predicting and explaining corruption across countries: A machine learning approach,” Gov. Inf. Q., vol. 37, no. 1, p. 101407, Jan. 2020, doi: 10.1016/J.GIQ.2019.101407.
K. S. M. T. Hossain, H. Harutyunyan, Y. Ning, B. Kennedy, N. Ramakrishnan, and A. Galstyan, “Identifying geopolitical event precursors using attention-based LSTMs,” Front. Artif. Intell., vol. 5, p. 893875, Oct. 2022, doi: 10.3389/FRAI.2022.893875/BIBTEX.
T. M. Oladele and E. F. Ayetiran, “Social Unrest Prediction Through Sentiment Analysis on Twitter Using Support Vector Machine: Experimental Study on Nigeria’s #EndSARS,” Open Inf. Sci., vol. 7, no. 1, Jan. 2023, doi: 10.1515/OPIS-2022-0141/MACHINEREADABLECITATION/RIS.
S. Wiesmüller, “The Relational Governance of Artificial Intelligence,” 2023, doi: 10.1007/978-3-031-25023-1.
Donnay and Karsten, “Big Data for Monitoring Political Instability,” http://journals.openedition.org/poldev, no. 8.1, Sep. 2017, doi: 10.4000/POLDEV.2468.
I. Medvedev, V. Ustyuzhanin, J. Zinkina, and A. Korotayev, “Machine Learning for Ranking Factors of Global and Regional Protest Destabilization with a Special Focus on Afrasian Instability Macrozone,” Comp. Sociol., vol. 21, no. 5, pp. 604–645, Oct. 2022, doi: 10.1163/15691330-BJA10062.
T. Obukhov and M. A. Brovelli, “Identifying Conditioning Factors and Predictors of Conflict Likelihood for Machine Learning Models: A Literature Review,” ISPRS Int. J. Geo-Information, vol. 12, no. 8, p. 322, Aug. 2023, doi: 10.3390/IJGI12080322/S1.
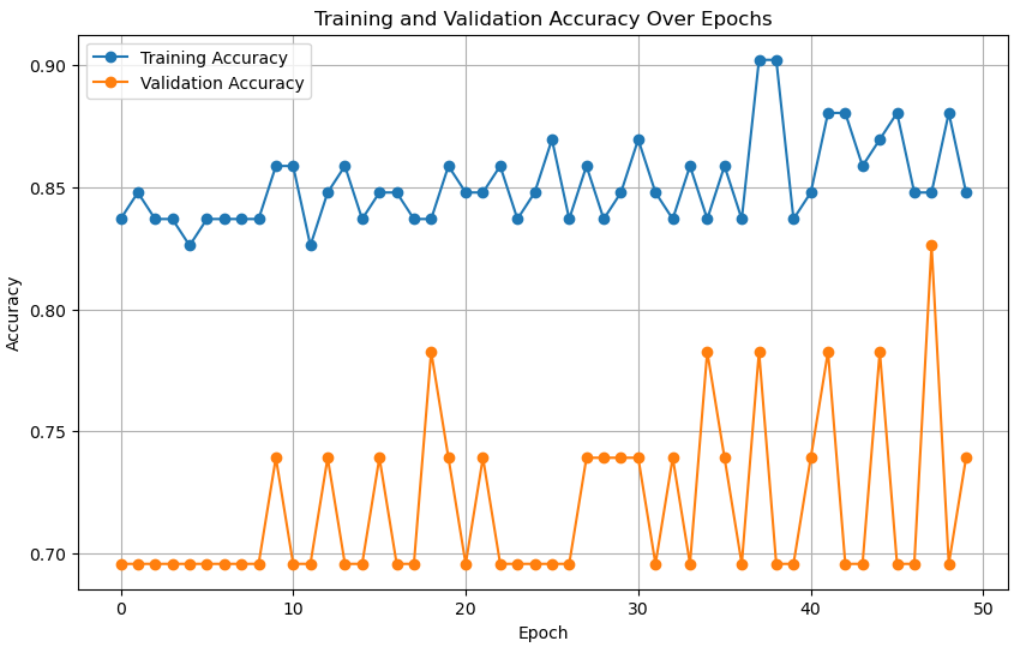
Published
How to Cite
Issue
Section
License
Copyright (c) 2023 50SEA

This work is licensed under a Creative Commons Attribution 4.0 International License.