A Systematic Review of Desertification Identification with Multispectral LANDSAT Image and Deep Learning Models
Keywords:
LANDSAT, Deep Learning, Desertification, GeoinformaticsAbstract
The use of multispectral Landsat images and deep learning models for desertification detection has been reviewed in this research. The role of deep learning models is found to significantly increase the identification accuracy of the researchers, complemented by the inclusion of Landsat imagery to capture key desertification indicators. The research reviews difficulties including geographical resolution, data variability, uncertainty, and validation, alongside different desertification identification methods, techniques, advancement, and limitations. The research also highlighted the necessity of historical data, data continuity, and data fusion, among other issues on data availability and quality. The research advocates for the combination of high-resolution photography, climate and weather data, and socioeconomic data for better desertification detection while the research has identified more complex deep learning architectures, better uncertainty estimation, explainability and interpretability improvement, and the integration of process-based models as potential areas of research. The research concludes by highlighting the importance of precise desertification identification in effective land administration and ecological preservation.
References
A. Baeza et al., “Long-lasting transition toward sustainable elimination of desert malaria under irrigation development,” Proc. Natl. Acad. Sci. U. S. A., vol. 110, no. 37, pp. 15157–15162, Sep. 2013, doi: 10.1073/PNAS.1305728110/SUPPL_FILE/SAPP.PDF.
L. Radonic, “Re-conceptualising Water Conservation: Rainwater Harvesting in the Desert of the Southwestern United States”, Accessed: Jan. 03, 2024. [Online]. Available: https://www.water-alternatives.org/index.php/alldoc/articles/vol12/v12issue2/499-a12-2-6/file
M. Abdelkareem and N. Al-Arifi, “The use of remotely sensed data to reveal geologic, structural, and hydrologic features and predict potential areas of water resources in arid regions,” Arab. J. Geosci., vol. 14, no. 8, pp. 1–15, Apr. 2021, doi: 10.1007/S12517-021-06942-6/METRICS.
M. E. H. Chowdhury et al., “Design, Construction and Testing of IoT Based Automated Indoor Vertical Hydroponics Farming Test-Bed in Qatar,” Sensors 2020, Vol. 20, Page 5637, vol. 20, no. 19, p. 5637, Oct. 2020, doi: 10.3390/S20195637.
J. Lee and K. Chung, “An Efficient Transmission Power Control Scheme for Temperature Variation in Wireless Sensor Networks,” Sensors 2011, Vol. 11, Pages 3078-3093, vol. 11, no. 3, pp. 3078–3093, Mar. 2011, doi: 10.3390/S110303078.
O. S. Mintaș, D. Mierliță, O. Berchez, A. Stanciu, A. Osiceanu, and A. G. Osiceanu, “Analysis of the Sustainability of Livestock Farms in the Area of the Southwest of Bihor County to Climate Change,” Sustain. 2022, Vol. 14, Page 8841, vol. 14, no. 14, p. 8841, Jul. 2022, doi: 10.3390/SU14148841.
P. Roy, “FOREST FIRE AND DEGRADATION ASSESSMENT USING SATELLITE REMOTE SENSING AND GEOGRAPHIC INFORMATION SYSTEM,” 2005, Accessed: Jan. 03, 2024. [Online]. Available: https://www.semanticscholar.org/paper/FOREST-FIRE-AND-DEGRADATION-ASSESSMENT-USING-REMOTE-Roy/a3ce1dff2fe6e1a5eb81eb5473b5e1114b9cd655
J. E. Lovich and J. R. Ennen, “Wildlife Conservation and Solar Energy Development in the Desert Southwest, United States,” Bioscience, vol. 61, no. 12, pp. 982–992, Dec. 2011, doi: 10.1525/BIO.2011.61.12.8.
“Sustainable agriculture and climate change An ActionAid rough guide Sustainable agriculture and climate change,” 2009, Accessed: Jan. 03, 2024. [Online]. Available: http://www.agassessment.org/
M. Belloumi, “Investigating the Impact of Climate Change on Agricultural Production in Eastern and Southern African Countries,” 2014, Accessed: Jan. 03, 2024. [Online]. Available: https://www.agrodep.org/sites/default/files/AGRODEPWP0003.pdf
M. Fujioka, “Mate and Nestling Desertion in Colonial Little Egrets,” Auk, vol. 106, no. 2, pp. 292–302, Apr. 1989, doi: 10.1093/AUK/106.2.292.
“Silent revolution through climate smart livestock farming in cold desert.” Accessed: Jan. 03, 2024. [Online]. Available: https://www.researchgate.net/publication/322298175_Silent_revolution_through_climate_smart_livestock_farming_in_cold_desert
D. Kushner, “The real story of stuxnet,” IEEE Spectr., vol. 50, no. 3, pp. 48–53, 2013, doi: 10.1109/MSPEC.2013.6471059.
K. M. R. Hunt, A. G. Turner, and L. C. Shaffrey, “Extreme Daily Rainfall in Pakistan and North India: Scale Interactions, Mechanisms, and Precursors,” Mon. Weather Rev., vol. 146, no. 4, pp. 1005–1022, Apr. 2018, doi: 10.1175/MWR-D-17-0258.1.
A. M. Hay, “The derivation of global estimates from a confusion matrix,” Int. J. Remote Sens., vol. 9, no. 8, pp. 1395–1398, 1988, doi: 10.1080/01431168808954945.
H. Gao, Z. Chen, and C. Li, “Sandwich Convolutional Neural Network for Hyperspectral Image Classification Using Spectral Feature Enhancement,” IEEE J. Sel. Top. Appl. Earth Obs. Remote Sens., vol. 14, pp. 3006–3015, 2021, doi: 10.1109/JSTARS.2021.3062872.
X. Yang, Y. Ye, X. Li, R. Y. K. Lau, X. Zhang, and X. Huang, “Hyperspectral image classification with deep learning models,” IEEE Trans. Geosci. Remote Sens., vol. 56, no. 9, pp. 5408–5423, Sep. 2018, doi: 10.1109/TGRS.2018.2815613.
J. Abdulridha, R. Ehsani, A. Abd-Elrahman, and Y. Ampatzidis, “A remote sensing technique for detecting laurel wilt disease in avocado in presence of other biotic and abiotic stresses,” Comput. Electron. Agric., vol. 156, pp. 549–557, Jan. 2019, doi: 10.1016/J.COMPAG.2018.12.018.
Q. Meng, X. Wen, L. Yuan, and H. Xu, “Factorization-Based Active Contour for Water-Land SAR Image Segmentation via the Fusion of Features,” IEEE Access, vol. 7, pp. 40347–40358, 2019, doi: 10.1109/ACCESS.2019.2905847.
C. Wu and X. Zhang, “Total Bregman divergence-based fuzzy local information C-means clustering for robust image segmentation,” Appl. Soft Comput., vol. 94, p. 106468, Sep. 2020, doi: 10.1016/J.ASOC.2020.106468.
P. Xiao, X. Tian, M. Liu, and W. Liu, “Multipath Smearing Suppression for Synthetic Aperture Radar Images of Harbor Scenes,” IEEE Access, vol. 7, pp. 20150–20162, 2019, doi: 10.1109/ACCESS.2019.2897779.
A. Asokan, J. Anitha, B. Patrut, D. Danciulescu, and D. J. Hemanth, “Deep Feature Extraction and Feature Fusion for Bi-Temporal Satellite Image Classification,” Comput. Mater. Contin., vol. 66, no. 1, pp. 373–388, Oct. 2020, doi: 10.32604/CMC.2020.012364.
D. Liu, F. Yang, H. Wei, and P. Hu, “Remote sensing image fusion method based on discrete wavelet and multiscale morphological transform in the IHS color space,” https://doi.org/10.1117/1.JRS.14.016518, vol. 14, no. 1, p. 016518, Mar. 2020, doi: 10.1117/1.JRS.14.016518.
“Master Thesis Tree Detection in Remote Sensing Imagery Baumerkennung in FernerkundungsBildmaterial”, [Online]. Available: https://www.uni-goettingen.de/de/document/download/e3004c6e53ca2fa0a30d53d98a52c24e.pdf/MA_Freudenberg.pdf
L. Costa, S. Kunwar, Y. Ampatzidis, and U. Albrecht, “Determining leaf nutrient concentrations in citrus trees using UAV imagery and machine learning,” Precis. Agric., vol. 23, no. 3, pp. 854–875, Jun. 2022, doi: 10.1007/S11119-021-09864-1/FIGURES/10.
J. Zhang, “Multi-source remote sensing data fusion: status and trends,” Int. J. Image Data Fusion, vol. 1, no. 1, pp. 5–24, 2010, doi: 10.1080/19479830903561035.
Y. Qian, M. Ye, and J. Zhou, “Hyperspectral image classification based on structured sparse logistic regression and three-dimensional wavelet texture features,” IEEE Trans. Geosci. Remote Sens., vol. 51, no. 4, pp. 2276–2291, 2013, doi: 10.1109/TGRS.2012.2209657.
S. Jozdani, D. Chen, D. Pouliot, and B. Alan Johnson, “A review and meta-analysis of Generative Adversarial Networks and their applications in remote sensing,” Int. J. Appl. Earth Obs. Geoinf., vol. 108, p. 102734, Apr. 2022, doi: 10.1016/J.JAG.2022.102734.
A. A. Sima and S. J. Buckley, “Optimizing SIFT for Matching of Short Wave Infrared and Visible Wavelength Images,” Remote Sens. 2013, Vol. 5, Pages 2037-2056, vol. 5, no. 5, pp. 2037–2056, Apr. 2013, doi: 10.3390/RS5052037.
T. Zhao, Y. Yang, H. Niu, D. Wang, and Y. Chen, “Comparing U-Net convolutional network with mask R-CNN in the performances of pomegranate tree canopy segmentation,” https://doi.org/10.1117/12.2325570, vol. 10780, pp. 210–218, Oct. 2018, doi: 10.1117/12.2325570.
Y. Lu, “Deep Learning for Remote Sensing Image Processing,” Comput. Model. Simul. Eng. Theses Diss., Aug. 2020, doi: 10.25777/9nwb-h685.
R. Kemker, C. Salvaggio, and C. Kanan, “Algorithms for semantic segmentation of multispectral remote sensing imagery using deep learning,” ISPRS J. Photogramm. Remote Sens., vol. 145, pp. 60–77, Nov. 2018, doi: 10.1016/J.ISPRSJPRS.2018.04.014.
P. Iyer, S. A, and S. Lal, “Deep learning ensemble method for classification of satellite hyperspectral images,” Remote Sens. Appl. Soc. Environ., vol. 23, p. 100580, Aug. 2021, doi: 10.1016/J.RSASE.2021.100580.
S. Malek, “Deep neural network models for image classification and regression,” May 2018.
S. Janarthanan, T. Ganesh Kumar, S. Janakiraman, R. K. Dhanaraj, and M. A. Shah, “An Efficient Multispectral Image Classification and Optimization Using Remote Sensing Data,” J. Sensors, vol. 2022, 2022, doi: 10.1155/2022/2004716.
R. Minetto, M. P. Segundo, G. Rotich, and S. Sarkar, “Measuring human and economic activity from satellite imagery to support city-scale decision-making during COVID-19 pandemic,” IEEE Trans. Big Data, vol. 7, no. 1, pp. 56–68, Mar. 2021, doi: 10.1109/TBDATA.2020.3032839.
“Mapping and classification of urban green spaces with object based image analysis and LiDAR data fusion.” Accessed: Jan. 03, 2024. [Online]. Available: https://sci-hub.se/10.4314/jfas.v10i1s.7
Y. Li, H. Zhang, X. Xue, Y. Jiang, and Q. Shen, “Deep learning for remote sensing image classification: A survey,” Wiley Interdiscip. Rev. Data Min. Knowl. Discov., vol. 8, no. 6, p. e1264, Nov. 2018, doi: 10.1002/WIDM.1264.
N. Laban, B. Abdellatif, H. M. Ebied, H. A. Shedeed, and M. F. Tolba, “Multiscale satellite image classification using deep learning approach,” Stud. Comput. Intell., vol. 836, pp. 165–186, 2020, doi: 10.1007/978-3-030-20212-5_9/COVER.
B. Trenčanová, V. Proença, and A. Bernardino, “Development of Semantic Maps of Vegetation Cover from UAV Images to Support Planning and Management in Fine-Grained Fire-Prone Landscapes,” Remote Sens. 2022, Vol. 14, Page 1262, vol. 14, no. 5, p. 1262, Mar. 2022, doi: 10.3390/RS14051262.
R. Goldblatt et al., “Using Landsat and nighttime lights for supervised pixel-based image classification of urban land cover,” Remote Sens. Environ., vol. 205, pp. 253–275, Feb. 2018, doi: 10.1016/J.RSE.2017.11.026.
B. Benjdira, Y. Bazi, A. Koubaa, and K. Ouni, “Unsupervised Domain Adaptation Using Generative Adversarial Networks for Semantic Segmentation of Aerial Images,” Remote Sens. 2019, Vol. 11, Page 1369, vol. 11, no. 11, p. 1369, Jun. 2019, doi: 10.3390/RS11111369.
Y. Chen, H. Jiang, C. Li, X. Jia, and P. Ghamisi, “Deep Feature Extraction and Classification of Hyperspectral Images Based on Convolutional Neural Networks,” IEEE Trans. Geosci. Remote Sens., vol. 54, no. 10, pp. 6232–6251, Oct. 2016, doi: 10.1109/TGRS.2016.2584107.
W. Ding and L. Zhang, “Building Detection in Remote Sensing Image Based on Improved YOLOV5,” Proc. - 2021 17th Int. Conf. Comput. Intell. Secur. CIS 2021, pp. 133–136, 2021, doi: 10.1109/CIS54983.2021.00036.
X. Li et al., “Prediction of Forest Fire Spread Rate Using UAV Images and an LSTM Model Considering the Interaction between Fire and Wind,” Remote Sens. 2021, Vol. 13, Page 4325, vol. 13, no. 21, p. 4325, Oct. 2021, doi: 10.3390/RS13214325.
H. Zhang, G. R. Arce, X. Zhao, and X. Ma, “Compressive hyperspectral image classification using a 3D coded convolutional neural network,” Opt. Express, Vol. 29, Issue 21, pp. 32875-32891, vol. 29, no. 21, pp. 32875–32891, Oct. 2021, doi: 10.1364/OE.437717.
Q. Gao, S. Lim, and X. Jia, “Hyperspectral Image Classification Using Convolutional Neural Networks and Multiple Feature Learning,” Remote Sens. 2018, Vol. 10, Page 299, vol. 10, no. 2, p. 299, Feb. 2018, doi: 10.3390/RS10020299.
F. H. Wagner, R. Dalagnol, Y. Tarabalka, T. Y. F. Segantine, R. Thomé, and M. C. M. Hirye, “U-Net-Id, an Instance Segmentation Model for Building Extraction from Satellite Images—Case Study in the Joanópolis City, Brazil,” Remote Sens. 2020, Vol. 12, Page 1544, vol. 12, no. 10, p. 1544, May 2020, doi: 10.3390/RS12101544.
S. S. Panda, D. P. Ames, and S. Panigrahi, “Application of Vegetation Indices for Agricultural Crop Yield Prediction Using Neural Network Techniques,” Remote Sens. 2010, Vol. 2, Pages 673-696, vol. 2, no. 3, pp. 673–696, Mar. 2010, doi: 10.3390/RS2030673.
R. L. Lawrence and A. Wrlght, “Rule-Based Classification Systems Using Classification and Regression Tree (CART) Analysis”.
J. Heikkonen, P. Jafarzadeh, Q. Zhou, and C. Yu, “Point RCNN: An Angle-Free Framework for Rotated Object Detection,” Remote Sens. 2022, Vol. 14, Page 2605, vol. 14, no. 11, p. 2605, May 2022, doi: 10.3390/RS14112605.
V. M. Sekhar and C. S. Kumar, “Laplacian: Reversible Data Hiding Technique,” Proc. IEEE Int. Conf. Image Inf. Process., vol. 2019-November, pp. 546–551, Nov. 2019, doi: 10.1109/ICIIP47207.2019.8985808.
M. Barbouchi, R. Abdelfattah, K. Chokmani, N. Ben Aissa, R. Lhissou, and A. El Harti, “Soil Salinity Characterization Using Polarimetric InSAR Coherence: Case Studies in Tunisia and Morocco,” IEEE J. Sel. Top. Appl. Earth Obs. Remote Sens., vol. 8, no. 8, pp. 3823–3832, Aug. 2015, doi: 10.1109/JSTARS.2014.2333535.
O. Tasar, S. L. Happy, Y. Tarabalka, and P. Alliez, “ColorMapGAN: Unsupervised Domain Adaptation for Semantic Segmentation Using Color Mapping Generative Adversarial Networks,” IEEE Trans. Geosci. Remote Sens., vol. 58, no. 10, pp. 7178–7193, Oct. 2020, doi: 10.1109/TGRS.2020.2980417.
J. Li, B. Liang, and Y. Wang, “A hybrid neural network for hyperspectral image classification,” Remote Sens. Lett., vol. 11, no. 1, pp. 96–105, Jan. 2020, doi: 10.1080/2150704X.2019.1686780.
L. Knopp, M. Wieland, M. Rättich, and S. Martinis, “A Deep Learning Approach for Burned Area Segmentation with Sentinel-2 Data,” Remote Sens. 2020, Vol. 12, Page 2422, vol. 12, no. 15, p. 2422, Jul. 2020, doi: 10.3390/RS12152422.
D. T. Meshesha and M. Abeje, “Developing crop yield forecasting models for four major Ethiopian agricultural commodities,” Remote Sens. Appl. Soc. Environ., vol. 11, pp. 83–93, Aug. 2018, doi: 10.1016/J.RSASE.2018.05.001.
T. Nishio, Y. Koda, J. Park, M. Bennis, and K. Doppler, “When Wireless Communications Meet Computer Vision in beyond 5G,” IEEE Commun. Stand. Mag., vol. 5, no. 2, pp. 76–83, Jun. 2021, doi: 10.1109/MCOMSTD.001.2000047.
Y. Lan, C. Shengde, and B. K. Fritz, “Current status and future trends of precision agricultural aviation technologies,” Int. J. Agric. Biol. Eng., vol. 10, no. 3, pp. 1–17, May 2017, doi: 10.25165/IJABE.V10I3.3088.
Y. Tian, G. Pan, and M. S. Alouini, “Applying Deep-Learning-Based Computer Vision to Wireless Communications: Methodologies, Opportunities, and Challenges,” IEEE Open J. Commun. Soc., vol. 2, pp. 132–143, 2021, doi: 10.1109/OJCOMS.2020.3042630.
W. Yao, Z. Zeng, C. Lian, and H. Tang, “Pixel-wise regression using U-Net and its application on pansharpening,” Neurocomputing, vol. 312, pp. 364–371, Oct. 2018, doi: 10.1016/J.NEUCOM.2018.05.103.
J. Ai, L. Yang, Y. Liu, K. Yu, and J. Liu, “Dynamic Landscape Fragmentation and the Driving Forces on Haitan Island, China,” L. 2022, Vol. 11, Page 136, vol. 11, no. 1, p. 136, Jan. 2022, doi: 10.3390/LAND11010136.
U. Shafi, R. Mumtaz, J. García-Nieto, S. A. Hassan, S. A. R. Zaidi, and N. Iqbal, “Precision Agriculture Techniques and Practices: From Considerations to Applications,” Sensors 2019, Vol. 19, Page 3796, vol. 19, no. 17, p. 3796, Sep. 2019, doi: 10.3390/S19173796.
M. Hameed et al., “Urbanization Detection Using LiDAR-Based Remote Sensing Images of Azad Kashmir Using Novel 3D CNNs,” J. Sensors, vol. 2022, 2022, doi: 10.1155/2022/6430120.
“TEMPORAL CHANGE DETECTION OF LAND USE/LAND COVER USING GIS AND REMOTE SENSING TECHNIQUES IN SOUTH GHOR REGIONS, AL-KARAK, JORDAN”, [Online]. Available: https://sci-hub.se/10.4314/jfas.v10i1s.7
D. Gao, Q. Sun, B. Hu, and S. Zhang, “A Framework for Agricultural Pest and Disease Monitoring Based on Internet-of-Things and Unmanned Aerial Vehicles,” Sensors 2020, Vol. 20, Page 1487, vol. 20, no. 5, p. 1487, Mar. 2020, doi: 10.3390/S20051487.
S. T. Seydi, V. Saeidi, B. Kalantar, N. Ueda, and A. A. Halin, “Fire-Net: A Deep Learning Framework for Active Forest Fire Detection,” J. Sensors, vol. 2022, 2022, doi: 10.1155/2022/8044390.
M. Ahangarha, R. Shah-Hosseini, and M. Saadatseresht, “Deep Learning-Based Change Detection Method for Environmental Change Monitoring Using Sentinel-2 Datasets,” Environ. Sci. Proc. 2021, Vol. 5, Page 15, vol. 5, no. 1, p. 15, Nov. 2020, doi: 10.3390/IECG2020-08544.
K. K. Patel, A. Kar, S. N. Jha, and M. A. Khan, “Machine vision system: A tool for quality inspection of food and agricultural products,” J. Food Sci. Technol., vol. 49, no. 2, pp. 123–141, Apr. 2012, doi: 10.1007/S13197-011-0321-4/METRICS.
T. Brosnan and D. W. Sun, “Improving quality inspection of food products by computer vision––a review,” J. Food Eng., vol. 61, no. 1, pp. 3–16, Jan. 2004, doi: 10.1016/S0260-8774(03)00183-3.
S. Xu, J. Wang, W. Shou, T. Ngo, A. M. Sadick, and X. Wang, “Computer Vision Techniques in Construction: A Critical Review,” Arch. Comput. Methods Eng. 2020 285, vol. 28, no. 5, pp. 3383–3397, Oct. 2020, doi: 10.1007/S11831-020-09504-3.
S. Du et al., “Controllable Image Captioning with Feature Refinement and Multilayer Fusion,” Appl. Sci. 2023, Vol. 13, Page 5020, vol. 13, no. 8, p. 5020, Apr. 2023, doi: 10.3390/APP13085020.
H. Waheed, N. Zafar, W. Akram, A. Manzoor, A. Gani, and S. U. Islam, “Deep Learning Based Disease, Pest Pattern and Nutritional Deficiency Detection System for ‘Zingiberaceae’ Crop,” Agric. 2022, Vol. 12, Page 742, vol. 12, no. 6, p. 742, May 2022, doi: 10.3390/AGRICULTURE12060742.
L. W. Kuswidiyanto, H. H. Noh, and X. Han, “Plant Disease Diagnosis Using Deep Learning Based on Aerial Hyperspectral Images: A Review,” Remote Sens. 2022, Vol. 14, Page 6031, vol. 14, no. 23, p. 6031, Nov. 2022, doi: 10.3390/RS14236031.
M. Z. Hossain, F. Sohel, M. F. Shiratuddin, H. Laga, and M. Bennamoun, “Text to Image Synthesis for Improved Image Captioning,” IEEE Access, vol. 9, pp. 64918–64928, 2021, doi: 10.1109/ACCESS.2021.3075579.
J. R. E. Jeffrey E. Lovich, “Wildlife Conservation and Solar Energy Development in the Desert Southwest, United States”, [Online]. Available: https://academic.oup.com/bioscience/article/61/12/982/392612
B. Zhu et al., “Gradient Structure Information-Guided Attention Generative Adversarial Networks for Remote Sensing Image Generation,” Remote Sens. 2023, Vol. 15, Page 2827, vol. 15, no. 11, p. 2827, May 2023, doi: 10.3390/RS15112827.
M. E. Paoletti, J. M. Haut, J. Plaza, and A. Plaza, “Deep learning classifiers for hyperspectral imaging: A review,” ISPRS J. Photogramm. Remote Sens., vol. 158, pp. 279–317, Dec. 2019, doi: 10.1016/J.ISPRSJPRS.2019.09.006.
K. M. and J. N. Ng’ombe, “Climate Change Impacts on Sustainable Maize Production in Sub-Saharan Africa: A Review”, [Online]. Available: https://www.intechopen.com/chapters/70098
“Water Extraction in SAR Images Using Features Analysis and Dual-Threshold Graph Cut Model”, [Online]. Available: https://www.mdpi.com/2072-4292/13/17/3465
A. A. Sima and S. J. Buckley, “Optimizing SIFT for Matching of Short Wave Infrared and Visible Wavelength Images,” Remote Sens. 2013, Vol. 5, Pages 2037-2056, vol. 5, no. 5, pp. 2037–2056, Apr. 2013, doi: 10.3390/RS5052037.
K. Zhang et al., “Panchromatic and multispectral image fusion for remote sensing and earth observation: Concepts, taxonomy, literature review, evaluation methodologies and challenges ahead,” Inf. Fusion, vol. 93, pp. 227–242, May 2023, doi: 10.1016/J.INFFUS.2022.12.026.
“Mapping and classification of urban green spaces with object based image analysis and LiDAR data fusion.” Accessed: Jan. 03, 2024. [Online]. Available: https://helda.helsinki.fi/items/082da038-fb48-4a9c-bb85-b4a89cad0c96
V. G. Narendra and K. S. Hareesh, “Quality Inspection and Grading of Agricultural and Food Products by Computer Vision-a Review,” Int. J. Comput. Appl., vol. 2, no. 1, pp. 43–65, May 2010, doi: 10.5120/612-863.
J. Cui, “Computer Artificial Intelligence Technology in the Visual Transmission System of Urban Lake Water Quality Inspection and Detection Images,” Wirel. Commun. Mob. Comput., vol. 2022, 2022, doi: 10.1155/2022/2119505.
K. Mulungu, J. N. Ng’ombe, K. Mulungu, and J. N. Ng’ombe, “Climate Change Impacts on Sustainable Maize Production in Sub-Saharan Africa: A Review,” Maize - Prod. Use, Nov. 2019, doi: 10.5772/INTECHOPEN.90033.
A. Voulodimos, N. Doulamis, A. Doulamis, and E. Protopapadakis, “Deep Learning for Computer Vision: A Brief Review,” Comput. Intell. Neurosci., vol. 2018, 2018, doi: 10.1155/2018/7068349.
D. Merkle and M. Middendorf, “Swarm intelligence,” Search Methodol. Introd. Tutorials Optim. Decis. Support Tech., pp. 401–435, 2005, doi: 10.1007/0-387-28356-0_14/COVER.
O. Coban, G. B. de Deyn, and M. van der Ploeg, “Soil microbiota as game-changers in restoration of degraded lands,” Science (80-. )., vol. 375, no. 6584, Mar. 2022, doi: 10.1126/SCIENCE.ABE0725/ASSET/87962C22-9471-4ED5-A872-9CB734500694/ASSETS/IMAGES/LARGE/SCIENCE.ABE0725-F4.JPG.
G. Shi, Y. Wu, T. Li, Q. Fu, and Y. Wei, “Mid- and long-term effects of biochar on soil improvement and soil erosion control of sloping farmland in a black soil region, China,” J. Environ. Manage., vol. 320, p. 115902, Oct. 2022, doi: 10.1016/J.JENVMAN.2022.115902.
X. Wang, L. Wang, S. Li, Z. Wang, M. Zheng, and K. Song, “Remote estimates of soil organic carbon using multi-temporal synthetic images and the probability hybrid model,” Geoderma, vol. 425, p. 116066, Nov. 2022, doi: 10.1016/J.GEODERMA.2022.116066.
L. Duarte, M. Cunha, and A. C. Teodoro, “Comparing Hydric Erosion Soil Loss Models in Rainy Mountainous and Dry Flat Regions in Portugal,” L. 2021, Vol. 10, Page 554, vol. 10, no. 6, p. 554, May 2021, doi: 10.3390/LAND10060554.
A. B. McBratney, M. L. Mendonça Santos, and B. Minasny, “On digital soil mapping,” Geoderma, vol. 117, no. 1–2, pp. 3–52, Nov. 2003, doi: 10.1016/S0016-7061(03)00223-4.
P. A. Sanchez et al., “Digital soil map of the world,” Science (80-. )., vol. 325, no. 5941, pp. 680–681, Aug. 2009, doi: 10.1126/SCIENCE.1175084/SUPPL_FILE/SANCHEZ.SOM.REVISION1.PDF.
B. Minasny and A. B. McBratney, “Digital soil mapping: A brief history and some lessons,” Geoderma, vol. 264, pp. 301–311, Feb. 2016, doi: 10.1016/J.GEODERMA.2015.07.017.
J. Padarian, B. Minasny, and A. B. McBratney, “Machine learning and soil sciences: A review aided by machine learning tools,” SOIL, vol. 6, no. 1, pp. 35–52, Feb. 2020, doi: 10.5194/SOIL-6-35-2020.
Y. Zhou, W. Wu, H. Wang, X. Zhang, C. Yang, and H. Liu, “Identification of Soil Texture Classes Under Vegetation Cover Based on Sentinel-2 Data With SVM and SHAP Techniques,” IEEE J. Sel. Top. Appl. Earth Obs. Remote Sens., vol. 15, pp. 3758–3770, 2022, doi: 10.1109/JSTARS.2022.3164140.
J. D. D. Jayaseeli and D. Malathi, “An Efficient Automated Road Region Extraction from High Resolution Satellite Images using Improved Cuckoo Search with Multi-Level Thresholding Schema,” Procedia Comput. Sci., vol. 167, pp. 1161–1170, Jan. 2020, doi: 10.1016/J.PROCS.2020.03.418.
M. E. Paoletti, J. M. Haut, J. Plaza, and A. Plaza, “A new deep convolutional neural network for fast hyperspectral image classification,” ISPRS J. Photogramm. Remote Sens., vol. 145, pp. 120–147, Nov. 2018, doi: 10.1016/J.ISPRSJPRS.2017.11.021.
M. Shoaib et al., “An advanced deep learning models-based plant disease detection: A review of recent research,” Front. Plant Sci., vol. 14, p. 1158933, Mar. 2023, doi: 10.3389/FPLS.2023.1158933/BIBTEX.
R. Chin, C. Catal, and A. Kassahun, “Plant disease detection using drones in precision agriculture,” Precis. Agric., vol. 24, no. 5, pp. 1663–1682, Oct. 2023, doi: 10.1007/S11119-023-10014-Y/TABLES/8.
R. Sathyavani, K. Jaganmohan, and B. Kalaavathi, “SAILFISH OPTIMIZATION ALGORITHM WITH DEEP CONVOLUTIONAL NEURAL NETWORK FOR NUTRIENT DEFICIENCY DETECTION IN RICE PLANTS,” J. Pharm. Negat. Results, vol. 14, pp. 1713–1728, Jan. 2023, doi: 10.47750/PNR.2023.14.02.217.
M. Khalid, M. S. Sarfraz, U. Iqbal, M. U. Aftab, G. Niedbała, and H. T. Rauf, “Real-Time Plant Health Detection Using Deep Convolutional Neural Networks,” Agric. 2023, Vol. 13, Page 510, vol. 13, no. 2, p. 510, Feb. 2023, doi: 10.3390/AGRICULTURE13020510.
D. Kumar and D. Kumar, “Hyperspectral Image Classification Using Deep Learning Models: A Review,” J. Phys. Conf. Ser., vol. 1950, no. 1, p. 012087, Aug. 2021, doi: 10.1088/1742-6596/1950/1/012087.
S. S. Néia, A. O. Artero, and C. B. Da Cunha, “QUALITY ANALYSIS FOR THE VRP SOLUTIONS USING COMPUTER VISION TECHNIQUES,” Pesqui. Operacional, vol. 37, no. 2, pp. 387–402, May 2017, doi: 10.1590/0101-7438.2017.037.02.0387.
D. Demir et al., “Quality inspection in PCBs and SMDs using computer vision techniques,” IECON Proc. (Industrial Electron. Conf., vol. 2, pp. 857–861, 1994, doi: 10.1109/IECON.1994.397899.
B. Zhang et al., “Principles, developments and applications of computer vision for external quality inspection of fruits and vegetables: A review,” Food Res. Int., vol. 62, pp. 326–343, Aug. 2014, doi: 10.1016/J.FOODRES.2014.03.012.
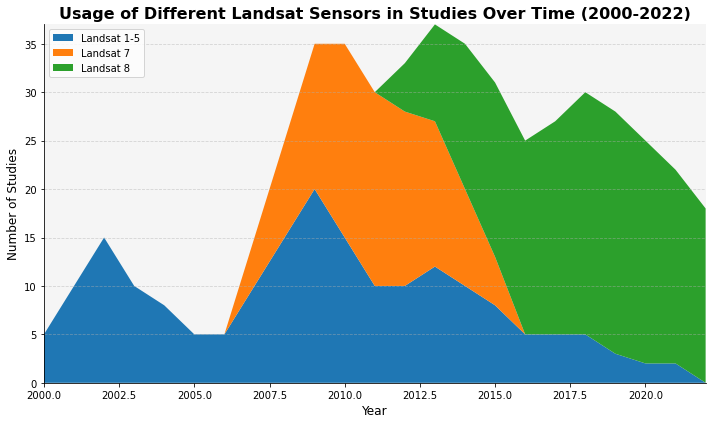
Published
How to Cite
Issue
Section
License
Copyright (c) 2024 50sea

This work is licensed under a Creative Commons Attribution 4.0 International License.