AlzheimerNet-V3: Automated Deep Learning Approach for Detecting Alzheimer's Disease
Keywords:
Early Detection, Dementia, Cognitive Decline, Diagnosis, Classification, AlzheimerNet-V3Abstract
Introduction/Importance of Study:
Alzheimer’s Disease (AD) stands as the highly prevalent form of dementia, culminating in a progressive neurological brain disorder characterized by deteriorating memory function and impaired daily activities due to brain cell damage. This singular ailment is both unique and fatal, underscoring the critical importance of early detection worldwide. Timely identification holds promise in preemptively addressing the future challenges faced by numerous individuals.
Novelty statement:
By scrutinizing the disease's ramifications via MRI imagery, Artificial Intelligence (AI) technology emerges as a valuable ally in categorizing AD patients, thus aiding in prognosticating the onset of this debilitating illness. In recent years, AI from Machine Learning (ML) tactics have proven instrumental in the diagnostic landscape of AD. This study employs a transfer learning methodology to accurately identify Alzheimer's patients using MRI examination. Specifically, we introduce an adapted deep learning model dubbed AlzheimerNet-V3, leveraging a tailored version of the Inception v3 architecture.
Material and Method:
Our investigation encompasses comprehensive experimentation and assesses the efficiency of AlzheimerNet-V3 in collaboration with further pre-trained specimens. Notably, AlzheimerNet-V3 achieves the conclusion of accuracy, the outcome of precision, recall, and development of F1-score was computed as 94.06% for all traits. Furthermore, comparative analysis against contemporary techniques underscores the efficacy of AlzheimerNet-V3 for Alzheimer's detection, highlighting its reliability for real-time implementation.
References
K. A. Matthews et al., “Racial and ethnic estimates of Alzheimer’s disease and related dementias in the United States (2015–2060) in adults aged ≥65 years,” Alzheimer’s Dement., vol. 15, no. 1, pp. 17–24, Jan. 2023, doi: 10.1016/J.JALZ.2018.06.3063.
“Dementia.” Accessed: Mar. 14, 2024. [Online]. Available: https://www.who.int/health-topics/dementia
P. Scheltens, “Imaging in Alzheimer’s disease,” Dialogues Clin. Neurosci., vol. 11, no. 2, p. 191, 2009, doi: 10.31887/DCNS.2009.11.2/PSCHELTENS.
M. Bari Antor et al., “A Comparative Analysis of Machine Learning Algorithms to Predict Alzheimer’s Disease,” J. Healthc. Eng., vol. 2021, 2021, doi: 10.1155/2021/9917919.
M. Shahbaz, S. Ali, A. Guergachi, A. Niazi, and A. Umer, “Classification of Alzheimer’s disease using machine learning techniques,” DATA 2019 - Proc. 8th Int. Conf. Data Sci. Technol. Appl., pp. 296–303, 2019, doi: 10.5220/0007949902960303.
S. Alam, G. R. Kwon, J. I. Kim, and C. S. Park, “Twin SVM-Based Classification of Alzheimer’s Disease Using Complex Dual-Tree Wavelet Principal Coefficients and LDA,” J. Healthc. Eng., vol. 2017, 2017, doi: 10.1155/2017/8750506.
S. Al-Shoukry, T. H. Rassem, and N. M. Makbol, “Alzheimer’s diseases detection by using deep learning algorithms: A mini-review,” IEEE Access, vol. 8, pp. 77131–77141, 2020, doi: 10.1109/ACCESS.2020.2989396.
T. A. Tuan, T. B. Pham, J. Y. Kim, and J. M. R. S. Tavares, “Alzheimer’s diagnosis using deep learning in segmenting and classifying 3D brain MR images,” Int. J. Neurosci., vol. 132, no. 7, pp. 689–698, 2022, doi: 10.1080/00207454.2020.1835900.
S. Murugan et al., “DEMNET: A Deep Learning Model for Early Diagnosis of Alzheimer Diseases and Dementia from MR Images,” IEEE Access, vol. 9, pp. 90319–90329, 2021, doi: 10.1109/ACCESS.2021.3090474.
C. Feng et al., “Deep Learning Framework for Alzheimer’s Disease Diagnosis via 3D-CNN and FSBi-LSTM,” IEEE Access, vol. 7, pp. 63605–63618, 2019, doi: 10.1109/ACCESS.2019.2913847.
S. Naz, A. Ashraf, and A. Zaib, “Transfer learning using freeze features for Alzheimer neurological disorder detection using ADNI dataset,” Multimed. Syst., vol. 28, no. 1, pp. 85–94, Feb. 2022, doi: 10.1007/S00530-021-00797-3/METRICS.
T. M. Ghazal et al., “Alzheimer Disease Detection Empowered with Transfer Learning,” Comput. Mater. Contin., vol. 70, no. 3, pp. 5005–5019, Oct. 2021, doi: 10.32604/CMC.2022.020866.
N. M. Khan, N. Abraham, and M. Hon, “Transfer Learning with Intelligent Training Data Selection for Prediction of Alzheimer’s Disease,” IEEE Access, vol. 7, pp. 72726–72735, 2019, doi: 10.1109/ACCESS.2019.2920448.
S. Savaş, “Detecting the Stages of Alzheimer’s Disease with Pre-trained Deep Learning Architectures,” Arab. J. Sci. Eng., vol. 47, no. 2, pp. 2201–2218, Feb. 2022, doi: 10.1007/S13369-021-06131-3/METRICS.
“Kaggle: Your Home for Data Science.” Accessed: Mar. 14, 2024. [Online]. Available: https://www.kaggle.com/code/tourist55/alzheimers-dataset-4-class-of-images
J. Liu, X. Tian, J. Wang, R. Guo, and H. Kuang, “MTFIL-Net: Automated Alzheimer’s disease detection and MMSE score prediction based on feature interactive learning,” Proc. - 2021 IEEE Int. Conf. Bioinforma. Biomed. BIBM 2021, pp. 1002–1007, 2021, doi: 10.1109/BIBM52615.2021.9669563.
J. Liu, M. Li, Y. Luo, S. Yang, W. Li, and Y. Bi, “Alzheimer’s disease detection using depthwise separable convolutional neural networks,” Comput. Methods Programs Biomed., vol. 203, p. 106032, May 2021, doi: 10.1016/J.CMPB.2021.106032.
S. Tabarestani et al., “Longitudinal prediction modeling of Alzheimer disease using recurrent neural networks,” 2019 IEEE EMBS Int. Conf. Biomed. Heal. Informatics, BHI 2019 - Proc., May 2019, doi: 10.1109/BHI.2019.8834556.
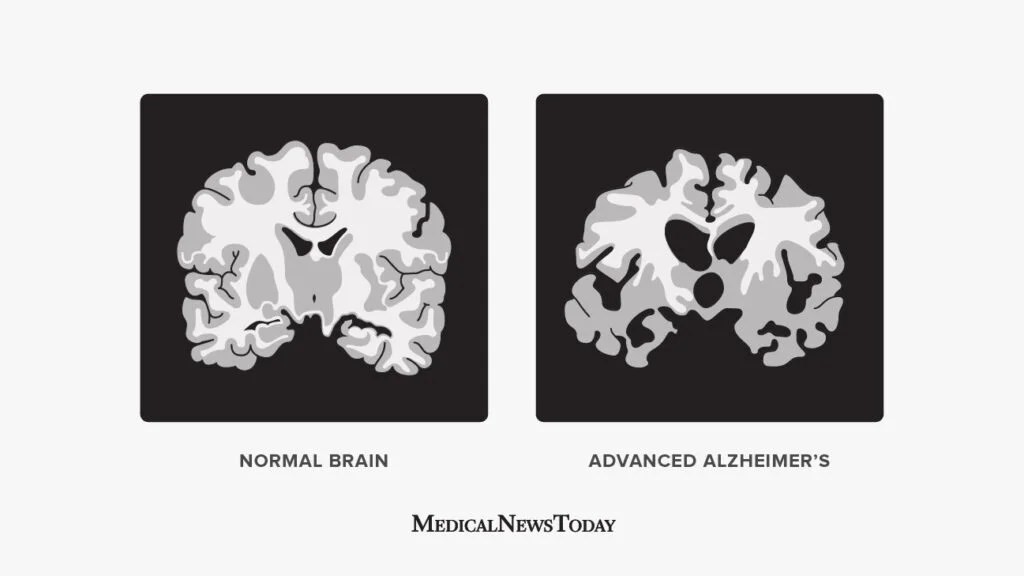
Published
How to Cite
Issue
Section
License
Copyright (c) 2024 50SEA

This work is licensed under a Creative Commons Attribution 4.0 International License.