Modeling of Post-Myocardial Infarction and Its Solution Through Artificial Neural Network
Keywords:
Artificial Neural Network, Myocardial Infarction, Mathematical Modeling.Abstract
Cardiovascular diseases, particularly myocardial infarction (MI) constitute a significant health concern globally. A myocardial infarction, which is commonly known as a heart attack, happens when a part of the heart muscle doesn’t get enough blood because of a blockage. Studying MI is complex and it requires looking at it from different angles. In recent years the fusion of mathematical modeling and artificial intelligence (AI) techniques has emerged as a promising avenue for understanding the complexities associated with MI. The primary goal of this study is to provide an AI-based solution for a new nonlinear mathematical model related to myocardial infarction phenomena. To obtain the solution we will use a well-known deep learning technique, known as artificial neural networks (ANNs) with the combination of the optimization technique Levenberg-Marquardt back propagation (LMB). This combined method is referred to as ANNs-LMB. The results obtained from the model using ANNs-LMB are compared with a reference dataset constructed through the adaptive MATLAB solver ode45. The numerical performance is validated through a reduction in mean square error (MSE). The MSE is around and the obtained results by ANNs-LMB almost overlapped with the reference dataset, which shows the accuracy and efficiency of the proposed methodology.
References
T. I. Siddiqui, A. K. K. S., and D. K. Dikshit, “Platelets and Atherothrombosis: Causes, Targets and Treatments for Thrombosis,” Curr. Med. Chem., vol. 20, no. 22, pp. 2779–2797, Jun. 2013, doi: 10.2174/0929867311320220004.
R. Hajar, “Risk Factors for Coronary Artery Disease: Historical Perspectives,” Heart Views, vol. 18, no. 3, p. 109, 2017, doi: 10.4103/HEARTVIEWS.HEARTVIEWS_106_17.
M. Lafci Büyükkahraman, G. K. Sabine, H. V. Kojouharov, B. M. Chen-Charpentier, S. R. McMahan, and J. Liao, “Using models to advance medicine: mathematical modeling of post-myocardial infarction left ventricular remodeling,” Comput. Methods Biomech. Biomed. Engin., vol. 25, no. 3, pp. 298–307, 2022, doi: 10.1080/10255842.2021.1953487.
Y. F. Jin, H. C. Han, J. Berger, Q. Dai, and M. L. Lindsey, “Combining experimental and mathematical modeling to reveal mechanisms of macrophage-dependent left ventricular remodeling,” BMC Syst. Biol., vol. 5, no. 1, pp. 1–14, May 2011, doi: 10.1186/1752-0509-5-60/FIGURES/6.
Y. Wang et al., “Mathematical modeling and stability analysis of macrophage activation in left ventricular remodeling post-myocardial infarction.,” BMC Genomics, vol. 13 Suppl 6, no. 6, pp. 1–8, Oct. 2012, doi: 10.1186/1471-2164-13-S6-S21/FIGURES/4.
U. Pagalay, L. Handayani, and A. Azzam, “Dynamics of Macrofages and Cytokines after Myocardial Infarction,” Jun. 2019, doi: 10.4108/EAI.2-5-2019.2284674.
A. C. Zeigler, A. R. Nelson, A. S. Chandrabhatla, O. Brazhkina, J. W. Holmes, and J. J. Saucerman, “Computational Model Predicts Paracrine and Intracellular Drivers of Fibroblast Phenotype After Myocardial Infarction,” bioRxiv, p. 840017, Nov. 2019, doi: 10.1101/840017.
L. C. Lee, G. S. Kassab, and J. M. Guccione, “Mathematical modeling of cardiac growth and remodeling,” Wiley Interdiscip. Rev. Syst. Biol. Med., vol. 8, no. 3, pp. 211–226, May 2016, doi: 10.1002/WSBM.1330.
N. Moise and A. Friedman, “A mathematical model of immunomodulatory treatment in myocardial infarction,” J. Theor. Biol., vol. 544, p. 111122, Jul. 2022, doi: 10.1016/J.JTBI.2022.111122.
A. D. Rouillard and J. W. Holmes, “Mechanical regulation of fibroblast migration and collagen remodelling in healing myocardial infarcts,” J. Physiol., vol. 590, no. 18, pp. 4585–4602, Sep. 2012, doi: 10.1113/JPHYSIOL.2012.229484.
S. M. Rikard et al., “Multiscale Coupling of an Agent-Based Model of Tissue Fibrosis and a Logic-Based Model of Intracellular Signaling,” Front. Physiol., vol. 10, p. 479813, Dec. 2019, doi: 10.3389/FPHYS.2019.01481/BIBTEX.
P. Sáez and E. Kuhl, “Computational modeling of acute myocardial infarction,” Comput. Methods Biomech. Biomed. Engin., vol. 19, no. 10, pp. 1107–1115, Jul. 2016, doi: 10.1080/10255842.2015.1105965.
E. Cutrì, A. Meoli, G. Dubini, F. Migliavacca, T. Y. Hsia, and G. Pennati, “Patient-specific biomechanical model of hypoplastic left heart to predict post-operative cardio-circulatory behaviour,” Med. Eng. Phys., vol. 47, pp. 85–92, Sep. 2017, doi: 10.1016/J.MEDENGPHY.2017.06.024.
M. Lo Schiavo, B. Prinari, J. A. Gronski, and A. V. Serio, “An artificial neural network approach for modeling the ward atmosphere in a medical unit,” Math. Comput. Simul., vol. 116, pp. 44–58, Oct. 2015, doi: 10.1016/J.MATCOM.2015.04.006.
J. L. G. Guirao, “On the stochastic observation for the nonlinear system of the emigration and migration effects via artificial neural networks,” Int. J. Math. Comput. Eng., vol. 1, no. 2, pp. 177–186, Dec. 2023, doi: 10.2478/IJMCE-2023-0014.
M. Shoaib, R. Kainat, M. Ijaz Khan, B. C. Prasanna Kumara, R. Naveen Kumar, and M. A. Zahoor Raja, “Darcy-Forchheimer entropy based hybrid nanofluid flow over a stretchable surface: intelligent computing approach,” Waves in Random and Complex Media, Sep. 2022, doi: 10.1080/17455030.2022.2122627.
Z. Sabir, R. Sadat, M. R. Ali, S. Ben Said, and M. Azhar, “A numerical performance of the novel fractional water pollution model through the Levenberg-Marquardt backpropagation method,” Arab. J. Chem., vol. 16, no. 2, p. 104493, Feb. 2023, doi: 10.1016/J.ARABJC.2022.104493.
T. Botmart, Z. Sabir, M. A. Z. Raja, W. weera, R. Sadat, and M. R. Ali, “Stochastic procedures to solve the nonlinear mass and heat transfer model of Williamson nanofluid past over a stretching sheet,” Ann. Nucl. Energy, vol. 181, p. 109564, Feb. 2023, doi: 10.1016/J.ANUCENE.2022.109564.
W. Weera et al., “Fractional Order Environmental and Economic Model Investigations Using Artificial Neural Network,” Comput. Mater. Contin., vol. 74, no. 1, pp. 1735–1748, Sep. 2022, doi: 10.32604/CMC.2023.032950.
“Stability Analysis Susceptible, Exposed, Infected, Recovered (SEIR) Model for Spread Model for Spread of Dengue Fever in Medan”, [Online]. Available: https://iopscience.iop.org/article/10.1088/1742-6596/954/1/012018
M. Umar et al., “Numerical Investigations through ANNs for Solving COVID-19 Model,” Int. J. Environ. Res. Public Heal. 2021, Vol. 18, Page 12192, vol. 18, no. 22, p. 12192, Nov. 2021, doi: 10.3390/IJERPH182212192.
Z. Sabir, M. A. Z. Raja, S. E. Alhazmi, M. Gupta, A. Arbi, and I. A. Baba, “Applications of artificial neural network to solve the nonlinear COVID-19 mathematical model based on the dynamics of SIQ,” J. Taibah Univ. Sci., vol. 16, no. 1, pp. 874–884, Dec. 2022, doi: 10.1080/16583655.2022.2119734.
Q. Haider, A. Hassan, and S. M. Eldin, “Artificial neural network scheme to solve the hepatitis B virus model,” Front. Appl. Math. Stat., vol. 9, p. 1072447, Mar. 2023, doi: 10.3389/FAMS.2023.1072447/BIBTEX.
Z. Sabir et al., “Artificial neural network scheme to solve the nonlinear influenza disease model,” Biomed. Signal Process. Control, vol. 75, p. 103594, May 2022, doi: 10.1016/J.BSPC.2022.103594.
S. Mall and S. Chakraverty, “Comparison of Artificial Neural Network Architecture in Solving Ordinary Differential Equations,” Adv. Artif. Neural Syst., vol. 2013, pp. 1–12, Dec. 2013, doi: 10.1155/2013/181895.
Y. Wen, T. Chaolu, and X. Wang, “Solving the initial value problem of ordinary differential equations by Lie group based neural network method,” PLoS One, vol. 17, no. 4, p. e0265992, Apr. 2022, doi: 10.1371/JOURNAL.PONE.0265992.
M. Bujak and N. G. Frangogiannis, “The role of TGF-β signaling in myocardial infarction and cardiac remodeling,” Cardiovasc. Res., vol. 74, no. 2, pp. 184–195, May 2007, doi: 10.1016/J.CARDIORES.2006.10.002/2/74-2-184-FIG4.GIF.
D. I. Jang et al., “The Role of Tumor Necrosis Factor Alpha (TNF-α) in Autoimmune Disease and Current TNF-α Inhibitors in Therapeutics,” Int. J. Mol. Sci. 2021, Vol. 22, Page 2719, vol. 22, no. 5, p. 2719, Mar. 2021, doi: 10.3390/IJMS22052719.
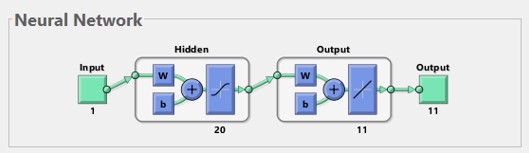
Downloads
Published
How to Cite
Issue
Section
License
Copyright (c) 2024 50SEA

This work is licensed under a Creative Commons Attribution 4.0 International License.