Classification of Medical Images Through Convolutional Neural Network Modification Method
Keywords:
Image Classification, Fuzzy Membership, VGG-19 Modified ModelAbstract
The COVID-19 positive, tuberculosis and pneumonia, share the trait of being able to be identified using radiological investigations, such as Chest X-ray (CXR) images. This paper aims to distinguish between four classes, including tuberculosis (TB), COVID-19 positive, healthy, and pneumonia using CXR images. Many deep-learning models such as a Convolutional Neural Network (CNN) have been developed for the Classification of CXR images. Deep learning-based models such as CNN offer significant advantages over traditional methods in the classification of diseases like TB, COVID-19, pneumonia, and healthy states. They provide higher accuracy, automation, early detection, reduced subjectivity, and resource efficiency, ultimately leading to improved patient care and outcomes. However, well-liked CNNs are massive models that require a lot of data to achieve optimal accuracy. In this paper, we propose a new CNN model that can be used to distinguish between different classes of CXR images. This model proves to be effective in classifying different diseases such as pneumonia, COVID-19, and tuberculosis. This study has used 6326 CXR images dataset containing COVID-19 positive, tuberculosis, and pneumonia and has normal images. In this dataset, 80% of the CXR images are taken for the training purpose and 20% are taken for the validation purpose, of the proposed CNN model. The proposed CNN modified model with parameter adjustment as well as using categorical cross-entropy as a loss function obtains the highest classification accuracy of 98.51% with a precision, recall, and F1 score of 0.98, 0.985, and 0.98 respectively.
References
J. E. Luján-García, Y. Villuendas-Rey, I. López-Yáñez, O. Camacho-Nieto, and C. Yáñez-Márquez, “NanoChest-Net: A Simple Convolutional Network for Radiological Studies Classification,” Diagnostics 2021, Vol. 11, Page 775, vol. 11, no. 5, p. 775, Apr. 2021, doi: 10.3390/DIAGNOSTICS11050775.
J. W. Song et al., “Omics-Driven Systems Interrogation of Metabolic Dysregulation in COVID-19 Pathogenesis,” Cell Metab., vol. 32, no. 2, pp. 188-202.e5, Aug. 2020, doi: 10.1016/J.CMET.2020.06.016.
S. Lalmuanawma, J. Hussain, and L. Chhakchhuak, “Applications of machine learning and artificial intelligence for Covid-19 (SARS-CoV-2) pandemic: A review,” Chaos, Solitons & Fractals, vol. 139, p. 110059, Oct. 2020, doi: 10.1016/J.CHAOS.2020.110059.
D. Das, K. C. Santosh, and U. Pal, “Truncated inception net: COVID-19 outbreak screening using chest X-rays,” Phys. Eng. Sci. Med., vol. 43, no. 3, pp. 915–925, Sep. 2020, doi: 10.1007/S13246-020-00888-X/TABLES/6.
L. Sarker, M. M. Islam, T. Hannan, and Z. Ahmed, “COVID-DenseNet: A Deep Learning Architecture to Detect COVID-19 from Chest Radiology Images,” May 2020, doi: 10.20944/PREPRINTS202005.0151.V1.
K. Li et al., “CT image visual quantitative evaluation and clinical classification of coronavirus disease (COVID-19),” Eur. Radiol., vol. 30, no. 8, pp. 4407–4416, Aug. 2020, doi: 10.1007/S00330-020-06817-6/FIGURES/5.
J. Chen et al., “Deep learning-based model for detecting 2019 novel coronavirus pneumonia on high-resolution computed tomography: a prospective study,” medRxiv, p. 2020.02.25.20021568, Mar. 2020, doi: 10.1101/2020.02.25.20021568.
A. K. Jaiswal, P. Tiwari, S. Kumar, D. Gupta, A. Khanna, and J. J. P. C. Rodrigues, “Identifying pneumonia in chest X-rays: A deep learning approach,” Measurement, vol. 145, pp. 511–518, Oct. 2019, doi: 10.1016/J.MEASUREMENT.2019.05.076.
S. Bharati, P. Podder, and M. R. H. Mondal, “Hybrid deep learning for detecting lung diseases from X-ray images,” Informatics Med. Unlocked, vol. 20, p. 100391, Jan. 2020, doi: 10.1016/J.IMU.2020.100391.
R. M. Pereira, D. Bertolini, L. O. Teixeira, C. N. Silla, and Y. M. G. Costa, “COVID-19 identification in chest X-ray images on flat and hierarchical classification scenarios,” Comput. Methods Programs Biomed., vol. 194, p. 105532, Oct. 2020, doi: 10.1016/J.CMPB.2020.105532.
T. Ozturk, M. Talo, E. A. Yildirim, U. B. Baloglu, O. Yildirim, and U. Rajendra Acharya, “Automated detection of COVID-19 cases using deep neural networks with X-ray images,” Comput. Biol. Med., vol. 121, p. 103792, Jun. 2020, doi: 10.1016/J.COMPBIOMED.2020.103792.
T. Rahman et al., “Transfer Learning with Deep Convolutional Neural Network (CNN) for Pneumonia Detection Using Chest X-ray,” Appl. Sci. 2020, Vol. 10, Page 3233, vol. 10, no. 9, p. 3233, May 2020, doi: 10.3390/APP10093233.
M. Mamalakis et al., “DenResCov-19: A deep transfer learning network for robust automatic classification of COVID-19, pneumonia, and tuberculosis from X-rays,” Comput. Med. Imaging Graph., vol. 94, p. 102008, Dec. 2021, doi: 10.1016/J.COMPMEDIMAG.2021.102008.
K. Verma, G. Sikka, A. Swaraj, S. Kumar, and A. Kumar, “Classification of COVID-19 on Chest X-Ray Images Using Deep Learning Model with Histogram Equalization and Lung Segmentation,” SN Comput. Sci., vol. 5, no. 4, pp. 1–15, Apr. 2024, doi: 10.1007/S42979-024-02695-7/METRICS.
“Rich Feature Hierarchies for Accurate Object Detection and Semantic Segmentation | IEEE Conference Publication | IEEE Xplore.” Accessed: May 05, 2024. [Online]. Available: https://ieeexplore.ieee.org/document/6909475
“Binary cross-entropy with deep learning technique for image classification.” Accessed: May 05, 2024. [Online]. Available: https://www.warse.org/IJATCSE/static/pdf/file/ijatcse175942020.pdf
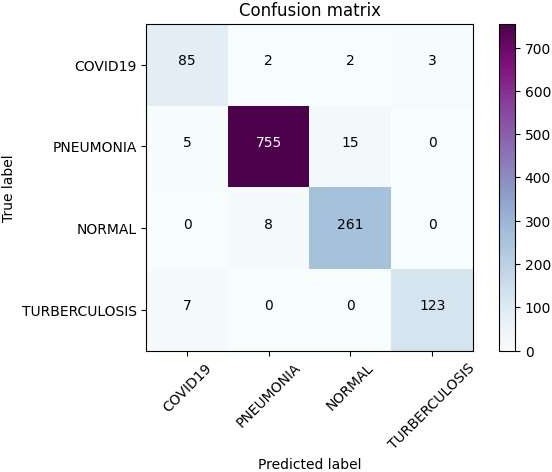
Downloads
Published
How to Cite
Issue
Section
License
Copyright (c) 2024 50SEA

This work is licensed under a Creative Commons Attribution 4.0 International License.