Model-Based vs Model Free Deep Reinforcement Learning Models for Cancer Treatment: A Critical Analysis with a Solution DRL Model
Keywords:
Deep Reinforcement Learning (DRL), Model-based learning, Model-free learning, Deep Learning, Neural Network (NN)Abstract
In the field of artificial intelligence, deep reinforcement learning (RL) has grown to be one of the most talked-about issues. It has a wide range of applications, including end-to-end control, robotic control, recommendation systems, and systems for natural language communication. In this paper, we have critically reviewed model-based and model-free deep reinforcement models for the treatment of cancer patients and evaluated each model based on some parameters. Based on the evaluation, a critical discussion is carried out highlighting the limitations and drawbacks of all the existing models. The analysis also gives suggestions and marks the key indicators of future needs in this domain. In the end, a solution model is proposed that tries to cover all the shortcomings and addresses the issues encountered in the existing models. The findings indicate that we can get a 94% accuracy rate with three agents, and that increasing the number of agents has no further effect on accuracy.
References
Krizhevsky A, Sutskever I, Hinton GE, 2012. ImageNet classification with deep convolutional neural networks. Proc 25th Neural Information Processing Systems, p.1097- 1105
Gu SX, Lillicrap T, Sutskever I, et al., 2016. Continuous deep Q-learning with model-based acceleration. Proc 33rd Int Conf on Machine Learning, p.2829-2838
Sutton RS, Barto AG, 2018. Reinforcement Learning: an Introduction (2nd Ed.). MIT Press, Cambridge, MA, USA
Hessel M, Modayil J, van Hasselt H, et al., 2018. Rainbow: combining improvements in deep reinforcement learning. https://arxiv.org/abs/1710.02298
Haarnoja T, Tang HR, Abbeel P, et al., 2017. Reinforcement learning with deep energy-based policies. Proc 34th Int
Conf on Machine Learning, p.1352-1361
Chua K, Calandra R, McAllister R, et al., 2018. Deep reinforcement learning in a handful of trials using probabilistic dynamics models. Proc 32nd Neural Information Processing Systems, p.4754-4765
Levine S, Koltun V, 2013. Guided policy search. Proc 30th Int Conf on Machine Learning, p.1-9.
Goharimanesh, M., Akbari, A., & Lotfi, B. (2015, October). On using fuzzy reinforcement learning to control the cancer cells. In 1st International Nastaran Cancer Symposium-2015.
Shen, C., Gonzalez, Y., Jung, H., Chen, L., Qin, N., & Jia, X. (2018). Automatic Inverse Treatment Planning for Cervical Cancer High Dose-Rate Brachytherapy via Deep Reinforcement Learning. International Journal of Radiation Oncology, Biology, Physics, 102(3), e540.
Capizzi, G., Sciuto, G. L., Napoli, C., Połap, D., & Woźniak, M. (2019). Small lung nodules detection based on fuzzy-logic and probabilistic neural network with bioinspired reinforcement learning. IEEE Transactions on Fuzzy Systems, 28(6), 1178-1189.
Shen, C., Gonzalez, Y., Chen, L., Jiang, S. B., & Jia, X. (2018). Intelligent parameter tuning in optimization-based iterative CT reconstruction via deep reinforcement learning. IEEE transactions on medical imaging, 37(6), 1430-1439.
Petousis, P., Winter, A., Speier, W., Aberle, D. R., Hsu, W., & Bui, A. A. (2019). Using sequential decision making to improve lung cancer screening performance. Ieee Access, 7, 119403-119419.
Daoud, S., Mdhaffar, A., Jmaiel, M., & Freisleben, B. (2020). Q-Rank: Reinforcement Learning for Recommending Algorithms to Predict Drug Sensitivity to Cancer Therapy. IEEE Journal of Biomedical and Health Informatics, 24(11), 3154-3161.
Liu, Z., Yao, C., Yu, H., & Wu, T. (2019). Deep reinforcement learning with its application for lung cancer detection in medical Internet of Things. Future Generation Computer Systems, 97, 1-9.
Padmanabhan, R., Meskin, N., & Haddad, W. M. (2020). Reinforcement learning-based control of drug dosing with applications to anesthesia and cancer therapy. In Control Applications for Biomedical Engineering Systems (pp. 251-297). Academic Press.
Yazdjerdi, P., Meskin, N., Al-Naemi, M., Al Moustafa, A. E., & Kovács, L. (2019). Reinforcement learning-based control of tumor growth under anti-angiogenic therapy. Computer methods and programs in biomedicine, 173, 15-26.
Padmanabhan, R., Meskin, N., & Haddad, W. M. (2017). Learning-based control of cancer chemotherapy treatment. IFAC-PapersOnLine, 50(1), 15127-15132.
Tseng, H. H., Luo, Y., Cui, S., Chien, J. T., Ten Haken, R. K., & Naqa, I. E. (2017). Deep reinforcement learning for automated radiation adaptation in lung cancer. Medical physics, 44(12), 6690-6705.
Capizzi, G., Sciuto, G. L., Napoli, C., Połap, D., & Woźniak, M. (2019). Small lung nodules detection based on fuzzy-logic and probabilistic neural network with bioinspired reinforcement learning. IEEE Transactions on Fuzzy Systems, 28(6), 1178-1189.
Padmanabhan, R., Meskin, N., & Haddad, W. M. (2017). Reinforcement learning-based control of drug dosing for cancer chemotherapy treatment. Mathematical biosciences, 293, 11-20.
Padmanabhan, R., Meskin, N., & Haddad, W. M. (2019). Optimal adaptive control of drug dosing using integral reinforcement learning. Mathematical biosciences, 309, 131-142.
Mnih, V., Kavukcuoglu, K., Silver, D., Graves, A., Antonoglou, I., Wierstra, D. and Riedmiller, M., 2013. Playing atari with deep reinforcement learning. arXiv preprint arXiv:1312.5602.
Mnih, V., Kavukcuoglu, K., Silver, D., Rusu, A.A., Veness, J., Bellemare, M.G., Graves, A., Riedmiller, M., Fidjeland, A.K., Ostrovski, G. and Petersen, S., 2015. Human-level control through deep reinforcement learning. nature, 518(7540), pp.529-533.
Vidyadhar, V., Nagaraj, R. and Ashoka, D.V., 2021. NetAI-Gym: Customized Environment for Network to Evaluate Agent Algorithm using Reinforcement Learning in Open-AI Gym Platform. International Journal of Advanced Computer Science and Applications, 12(4).
Brockman, G., Cheung, V., Pettersson, L., Schneider, J., Schulman, J., Tang, J. and Zaremba, W., 2016. Openai gym. arXiv preprint arXiv:1606.01540.
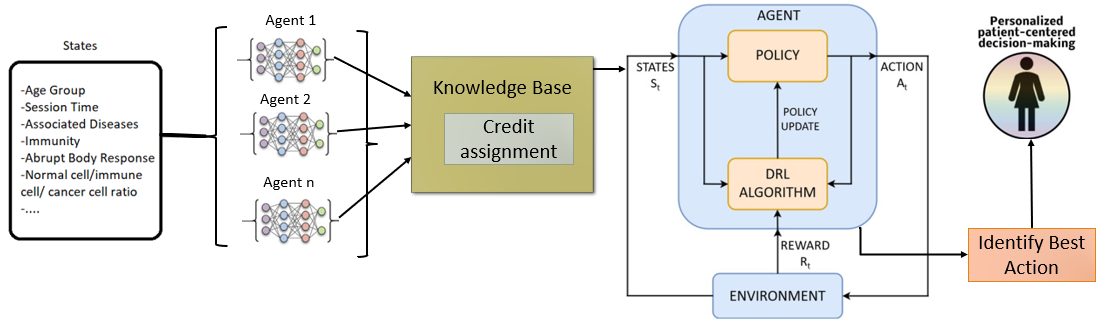
Downloads
Published
How to Cite
Issue
Section
License
Copyright (c) 2024 50SEA

This work is licensed under a Creative Commons Attribution 4.0 International License.