Unlocking Potential: Personality-Aware TVET Course Recommendations Revolutionize Skill Development
Keywords:
TVET Digitization, BFI Personality Traits, Personality-Aware Recommender Systems, Industry 5.0, Digital Skills DevelopmentAbstract
Personality is a complex amalgamation of ideas, behaviors, and social constructs that shape our self-perception and influence our interactions with others. It tends to remain relatively stable over time. The development of personality-aware recommendation systems is driven by the understanding that human behavior and personality play a significant role in skill acquisition, career progression, and overall success. Technical and Vocational Education and Training (TVET) is crucial in building a skilled workforce, particularly in response to the demands of Industry 5.0. Unlike conventional recommendation systems, personality-aware systems effectively address persistent challenges such as the cold start problem and data sparsity. This paper introduces the Personality-aware TVET Course Recommender System (TCRS), which suggests the top three TVET courses by considering trainees' personality traits, demographic information, and the historical success patterns of previous trainees in similar courses. A standout feature of the TCRS is its Academic System Learner, which continuously incorporates insights from individual trainees' progress in TVET courses, thereby enhancing the accuracy of its machine learning model for predictive analysis. The effectiveness of the TCRS is assessed using seven classifiers, yielding notable prediction accuracies: 99% with Random Forest, 98% with Decision Tree, and 89% with k-Nearest Neighbors (kNN). In real-time testing, the TCRS demonstrated an accuracy rate of 84%.
References
S. Dhelim, N. Aung, M. A. Bouras, H. Ning, and E. Cambria, “A survey on personality-aware recommendation systems,” Artif. Intell. Rev. 2021 553, vol. 55, no. 3, pp. 2409–2454, Sep. 2021, doi: 10.1007/S10462-021-10063-7.
K. K. Jena et al., “E-Learning Course Recommender System Using Collaborative Filtering Models,” Electron. 2023, Vol. 12, Page 157, vol. 12, no. 1, p. 157, Dec. 2022, doi: 10.3390/ELECTRONICS12010157.
R. H. and P. Pu, “Enhancing collaborative filtering systems with personality information,” Proc. fifth ACM Conf. Recomm. Syst. RecSys ’11. New York, NY, USA Assoc. Comput. Mach., pp. 197–204, 2011, [Online]. Available: https://dl.acm.org/doi/abs/10.1145/2043932.2043969
A. J. Fernández-García, R. Rodríguez-Echeverría, J. C. Preciado, J. M. Conejero Manzano, and F. Sánchez-Figueroa, “Creating a recommender system to support higher education students in the subject enrollment decision,” IEEE Access, vol. 8, pp. 189069–189088, 2020, doi: 10.1109/ACCESS.2020.3031572.
H. Ning, S. Dhelim, and N. Aung, “PersoNet: Friend Recommendation System Based on Big-Five Personality Traits and Hybrid Filtering,” IEEE Trans. Comput. Soc. Syst., vol. 6, no. 3, pp. 394–402, Jun. 2019, doi: 10.1109/TCSS.2019.2903857.
M. P. O’Mahony and B. Smyth, “A recommender system for on-line course enrolment: An initial study,” RecSys’07 Proc. 2007 ACM Conf. Recomm. Syst., pp. 133–136, 2007, doi: 10.1145/1297231.1297254.
B. Mondal, O. Patra, S. Mishra, and P. Patra, “A course recommendation system based on grades,” 2020 Int. Conf. Comput. Sci. Eng. Appl. ICCSEA 2020, Mar. 2020, doi: 10.1109/ICCSEA49143.2020.9132845.
“Feed | LinkedIn.” Accessed: Aug. 23, 2024. [Online]. Available: https://www.linkedin.com/feed/
Z. Gulzar, A. A. Leema, and G. Deepak, “PCRS: Personalized Course Recommender System Based on Hybrid Approach,” Procedia Comput. Sci., vol. 125, pp. 518–524, Jan. 2018, doi: 10.1016/J.PROCS.2017.12.067.
K. Bhumichitr, S. Channarukul, N. Saejiem, R. Jiamthapthaksin, and K. Nongpong, “Recommender Systems for university elective course recommendation,” Proc. 2017 14th Int. Jt. Conf. Comput. Sci. Softw. Eng. JCSSE 2017, Sep. 2017, doi: 10.1109/JCSSE.2017.8025933.
M. Elahi, M. Braunhofer, F. Ricci, and M. Tkalcic, “Personality-Based Active Learning for Collaborative Filtering Recommender Systems,” Lect. Notes Comput. Sci. (including Subser. Lect. Notes Artif. Intell. Lect. Notes Bioinformatics), vol. 8249 LNAI, pp. 360–371, 2013, doi: 10.1007/978-3-319-03524-6_31.
B. Ferwerda, M. Tkalcic, E. Yang, and M. Schedl, “Personality traits predict music taxonomy preferences,” Conf. Hum. Factors Comput. Syst. - Proc., vol. 18, pp. 2241–2246, Apr. 2015, doi: 10.1145/2702613.2732754.
“Using personality information in collaborative filtering for new users | Request PDF.” Accessed: Aug. 23, 2024. [Online]. Available: https://www.researchgate.net/publication/293007192_Using_personality_information_in_collaborative_filtering_for_new_users
“Online Friend Recommendation through Personality Matching and Collaborative Filtering.” Accessed: Aug. 23, 2024. [Online]. Available: https://www.researchgate.net/publication/268370462_Online_Friend_Recommendation_through_Personality_Matching_and_Collaborative_Filtering
P. J. Corr and G. Matthews, “The Cambridge Handbook of Personality Psychology,” Cambridge Handb. Personal. Psychol. Second Ed., pp. 1–551, Sep. 2020, doi: 10.1017/9781108264822.
S. Dhelim, L. Chen, N. Aung, W. Zhang, and H. Ning, “A hybrid personality-aware recommendation system based on personality traits and types models,” J. Ambient Intell. Humaniz. Comput. 2022 149, vol. 14, no. 9, pp. 12775–12788, Jul. 2022, doi: 10.1007/S12652-022-04200-5.
M. Kleć, A. Wieczorkowska, K. Szklanny, and W. Strus, “Beyond the Big Five personality traits for music recommendation systems,” Eurasip J. Audio, Speech, Music Process., vol. 2023, no. 1, pp. 1–17, Dec. 2023, doi: 10.1186/S13636-022-00269-0/FIGURES/7.
J. C. Patrícia Alves, Helena Martins, Pedro Saraiva, “Group Recommender Systems for Tourism: How does Personality predicts Preferences for Attractions, Travel Motivations, Preferences and Concerns?”, [Online]. Available: https://www.researchsquare.com/article/rs-1762820/v1
D. A. Cobb-Clark and S. Schurer, “The stability of big-five personality traits,” Econ. Lett., vol. 115, no. 1, pp. 11–15, Apr. 2012, doi: 10.1016/J.ECONLET.2011.11.015.
J. Leng et al., “Industry 5.0: Prospect and retrospect,” J. Manuf. Syst., vol. 65, pp. 279–295, Oct. 2022, doi: 10.1016/J.JMSY.2022.09.017.
R. H. Hassan and M. T. Hassan, “An Ensemble Classifier for TVET Course prediction using Big Five Personality Traits,” 2023 25th Int. Multi Top. Conf. INMIC 2023 - Proc., 2023, doi: 10.1109/INMIC60434.2023.10465760.
C. Stephanidis, “Paradigm shifts towards an inclusive society: From the Desktop to Human-Centered Artificial Intelligence,” ACM Int. Conf. Proceeding Ser., Sep. 2023, doi: 10.1145/3609987.3610109/ASSET/9FA6FD32-7F82-4911-8AF1-6FEA1107E99F/ASSETS/3609987.3610109.FP.PNG.
“TVET Skills Gap Analysis in Electrical and Electronic Industry: Perspectives from Academicians and Industry Players”, [Online]. Available: https://www.crossref.org/metadatamanager/publications/10.33411%2Fijist/addarticle
“Sustainable Development Goals - Pakistan TVET REFORM Support Programme.” Accessed: Aug. 23, 2024. [Online]. Available: https://tvetreform.org.pk/sustainable-development-goals/
R. H. Hassan, M. T. Hassan, S. Naseer, Z. Khan, and M. Jeon, “ICT Enabled TVET Education: A Systematic Literature Review,” IEEE Access, vol. 9, pp. 81624–81650, 2021, doi: 10.1109/ACCESS.2021.3085910.
S. M. A. Rana Hammad Hassan, “Identification of Trainees Enrollment Behavior and Course Selection Variables in Technical and Vocational Education Training (TVET) Program Using Education Data Mining”, [Online]. Available: https://www.mecs-press.org/ijmecs/ijmecs-v11-n10/v11n10-2.html
R. H. H. and M. T. Hassan, “A Meta-Analysis of Correlation Between Big Five Personality Traits and TVET Skills Acquisition,” J. Xi’an Shiyou Univ. Nat. Sci. Ed., vol. 20, no. 7, 2024.
J. Parry and M. J. Mojica, “Why ADB Uses Storytelling in Knowledge Management,” Oct. 2023, doi: 10.22617/BRF230384.
“Labour imbalances.” Accessed: Aug. 23, 2024. [Online]. Available: https://www.thenews.com.pk/print/1081706-labour-imbalances
and R. L. Amit Ojha , Mahmoud Habibnezhad , Houtan Jebelli, “Barrier Analysis of Effective Implementation of Robotics in the Construction Industry”, [Online]. Available: https://ascelibrary.org/doi/10.1061/9780784483961.069
“PVTC Official Website.” Accessed: Aug. 23, 2024. [Online]. Available: https://www.pvtc.gop.pk//
P. Lops, M. de Gemmis, and G. Semeraro, “Content-based Recommender Systems: State of the Art and Trends,” Recomm. Syst. Handb., pp. 73–105, 2011, doi: 10.1007/978-0-387-85820-3_3.
J. S. Breese, D. Heckerman, and C. Kadie, “Empirical Analysis of Predictive Algorithms for Collaborative Filtering,” Jan. 2013, Accessed: Aug. 23, 2024. [Online]. Available: https://arxiv.org/abs/1301.7363v1
R. Burke, “Hybrid recommender systems: Survey and experiments,” User Model. User-Adapted Interact., vol. 12, no. 4, pp. 331–370, 2002, doi: 10.1023/A:1021240730564/METRICS.
S. Algarni and F. Sheldon, “Systematic Review of Recommendation Systems for Course Selection,” Mach. Learn. Knowl. Extr. 2023, Vol. 5, Pages 560-596, vol. 5, no. 2, pp. 560–596, Jun. 2023, doi: 10.3390/MAKE5020033.
Y. Ma, R. Ouyang, X. Long, Z. Gao, T. Lai, and C. Fan, “DORIS: Personalized course recommendation system based on deep learning,” PLoS One, vol. 18, no. 6, p. e0284687, Jun. 2023, doi: 10.1371/JOURNAL.PONE.0284687.
Y. Zheng and A. Subramaniyan, “Personality-Aware Collaborative Learning: Models and Explanations,” Adv. Intell. Syst. Comput., vol. 926, pp. 631–642, 2020, doi: 10.1007/978-3-030-15032-7_53.
F. Xia, N. Y. Asabere, H. Liu, Z. Chen, and W. Wang, “Socially Aware Conference Participant Recommendation with Personality Traits,” IEEE Syst. J., vol. 11, no. 4, pp. 2255–2266, Dec. 2017, doi: 10.1109/JSYST.2014.2342375.
N. Y. Asabere, A. Acakpovi, and M. B. Michael, “Improving Socially-Aware Recommendation Accuracy Through Personality,” IEEE Trans. Affect. Comput., vol. 9, no. 3, pp. 351–361, Jul. 2018, doi: 10.1109/TAFFC.2017.2695605.
A. I. Hariadi and D. Nurjanah, “Hybrid attribute and personality based recommender system for book recommendation,” Proc. 2017 Int. Conf. Data Softw. Eng. ICoDSE 2017, vol. 2018-January, pp. 1–5, Jul. 2017, doi: 10.1109/ICODSE.2017.8285874.
M. F. Uddin, S. Banerjee, and J. Lee, “Recommender system framework for academic choices: Personality based recommendation engine (PBRE),” Proc. - 2016 IEEE 17th Int. Conf. Inf. Reuse Integr. IRI 2016, pp. 476–483, 2016, doi: 10.1109/IRI.2016.70.
“Is Big Five better than MBTI? A personality computing challenge using Twitter data”, [Online]. Available: https://books.openedition.org/aaccademia/3147
M. Qamhieh, H. Sammaneh, and M. N. Demaidi, “PCRS: Personalized Career-Path Recommender System for Engineering Students,” IEEE Access, vol. 8, pp. 214039–214049, 2020, doi: 10.1109/ACCESS.2020.3040338.
M. Guesmi et al., “Interactive Explanation with Varying Level of Details in an Explainable Scientific Literature Recommender System,” Int. J. Human–Computer Interact., Oct. 2023, doi: 10.1080/10447318.2023.2262797.
B. Rammstedt and O. P. John, “Measuring personality in one minute or less: A 10-item short version of the Big Five Inventory in English and German,” J. Res. Pers., vol. 41, no. 1, pp. 203–212, Feb. 2007, doi: 10.1016/J.JRP.2006.02.001.
“The short IPIP-BFM-20 questionnaire for measuring the big five.” Accessed: Aug. 23, 2024. [Online]. Available: https://www.researchgate.net/publication/285203927_The_short_IPIP-BFM-20_questionnaire_for_measuring_the_big_five
S. D. Gosling, P. J. Rentfrow, and W. B. Swann, “A very brief measure of the Big-Five personality domains,” J. Res. Pers., vol. 37, no. 6, pp. 504–528, Dec. 2003, doi: 10.1016/S0092-6566(03)00046-1.
A. A. Nana Yaw Asabere, “ROPPSA: TV Program Recommendation Based on Personality and Social Awareness”, [Online]. Available: Nana Yaw Asabere, Amevi Acakpovi
M. Komarraju, S. J. Karau, R. R. Schmeck, and A. Avdic, “The Big Five personality traits, learning styles, and academic achievement,” Pers. Individ. Dif., vol. 51, no. 4, pp. 472–477, Sep. 2011, doi: 10.1016/J.PAID.2011.04.019.
X. Sun, B. Liu, Q. Meng, J. Cao, J. Luo, and H. Yin, “Group-level personality detection based on text generated networks,” World Wide Web, vol. 23, no. 3, pp. 1887–1906, May 2020, doi: 10.1007/S11280-019-00729-2/METRICS.
W. R. dos Santos, R. M. S. Ramos, and I. Paraboni, “Computational personality recognition from Facebook text: psycholinguistic features, words and facets,” New Rev. Hypermedia Multimed., vol. 25, no. 4, pp. 268–287, Oct. 2019, doi: 10.1080/13614568.2020.1722761.
A. Darliansyah, M. A. Naeem, F. Mirza, and R. Pears, “SENTIPEDE: A Smart System for Sentiment-based Personality Detection from Short Texts,” J. Univers. Comput. Sci., vol. 25, no. 10, pp. 1323–1352, 2019.
Y. Kim and J. H. Kim, “Using computer vision techniques on Instagram to link users’ personalities and genders to the features of their photos: An exploratory study,” Inf. Process. Manag., vol. 54, no. 6, pp. 1101–1114, Nov. 2018, doi: 10.1016/J.IPM.2018.07.005.
H. Zhu, L. Li, H. Jiang, and A. Tan, “Inferring Personality Traits from Attentive Regions of User Liked Images Via Weakly Supervised Dual Convolutional Network,” Neural Process. Lett., vol. 51, no. 3, pp. 2105–2121, Jun. 2020, doi: 10.1007/S11063-019-09987-7/METRICS.
L. Zhang, S. Peng, and S. Winkler, “PersEmoN: A Deep Network for Joint Analysis of Apparent Personality, Emotion and Their Relationship,” IEEE Trans. Affect. Comput., vol. 13, no. 1, pp. 298–305, 2022, doi: 10.1109/TAFFC.2019.2951656.
M. M. Tadesse, H. Lin, B. Xu, and L. Yang, “Personality Predictions Based on User Behavior on the Facebook Social Media Platform,” IEEE Access, vol. 6, pp. 61959–61969, 2018, doi: 10.1109/ACCESS.2018.2876502.
G. Nave, J. Minxha, D. M. Greenberg, M. Kosinski, D. Stillwell, and J. Rentfrow, “Musical Preferences Predict Personality: Evidence From Active Listening and Facebook Likes,” https://doi.org/10.1177/0956797618761659, vol. 29, no. 7, pp. 1145–1158, Mar. 2018, doi: 10.1177/0956797618761659.
N. Annalyn, M. W. Bos, L. Sigal, and B. Li, “Predicting Personality from Book Preferences with User-Generated Content Labels,” IEEE Trans. Affect. Comput., vol. 11, no. 3, pp. 482–492, Jul. 2020, doi: 10.1109/TAFFC.2018.2808349.
N. D. Lynn and A. W. R. Emanuel, “A review on Recommender Systems for course selection in higher education,” IOP Conf. Ser. Mater. Sci. Eng., vol. 1098, no. 3, p. 032039, Mar. 2021, doi: 10.1088/1757-899X/1098/3/032039.
“Competency Based Training and Assessment (CBT & A) - Pakistan TVET REFORM Support Programme.” Accessed: Aug. 23, 2024. [Online]. Available: https://tvetreform.org.pk/competency-based-training-and-assessment-cbt-a/
N. R. P. Galit Shmueli, Kenneth C. Lichtendahl Jr., Peter C. Bruce, Inbal Yahav, “Data mining for business analytics: concepts, techniques, and applications in R”, [Online]. Available: https://www.wiley.com/en-be/Data+Mining+for+Business+Analytics%3A+Concepts%2C+Techniques%2C+and+Applications+in+R-p-9781118879337
“Proceedings of the 1st Workshop on Deep Learning for Recommender Systems | ACM Other conferences.” Accessed: Aug. 23, 2024. [Online]. Available: https://dl.acm.org/doi/proceedings/10.1145/2988450
S. Dhelim, N. Aung, and H. Ning, “Mining user interest based on personality-aware hybrid filtering in social networks,” Knowledge-Based Syst., vol. 206, p. 106227, Oct. 2020, doi: 10.1016/J.KNOSYS.2020.106227.
J. Sun, D. Ren, and D. Xu, “Leveraging User Personality and Tag Information for One Class Collaborative Filtering,” Lect. Notes Comput. Sci. (including Subser. Lect. Notes Artif. Intell. Lect. Notes Bioinformatics), vol. 11164 LNCS, pp. 830–840, 2018, doi: 10.1007/978-3-030-00776-8_76.
Z. Yusefi Hafshejani, M. Kaedi, and A. Fatemi, “Improving sparsity and new user problems in collaborative filtering by clustering the personality factors,” Electron. Commer. Res., vol. 18, no. 4, pp. 813–836, Dec. 2018, doi: 10.1007/S10660-018-9287-X/METRICS.
R. H. Hassan and M. T. Hassan, “Identification of Technical and Vocational Education and Training (TVET) trainee’s personality attributes which impact skills learning,” 2024 5th Int. Conf. Adv. Comput. Sci. ICACS 2024, 2024, doi: 10.1109/ICACS60934.2024.10473268.
S. Dhelim, H. Ning, N. Aung, R. Huang, and J. Ma, “Personality-Aware Product Recommendation System Based on User Interests Mining and Metapath Discovery,” IEEE Trans. Comput. Soc. Syst., vol. 8, no. 1, pp. 86–98, Feb. 2021, doi: 10.1109/TCSS.2020.3037040.
J. A. Recio-Garcia, G. Jimenez-Diaz, A. A. Sanchez-Ruiz, and B. Diaz-Agudo, “Personality aware recommendations to groups,” RecSys’09 - Proc. 3rd ACM Conf. Recomm. Syst., pp. 325–328, 2009, doi: 10.1145/1639714.1639779.
R. K. Kamat and R. S. Kamath, “Educational Data Mining with R and Rattle,” Educ. Data Min. with R Ratt., Sep. 2022, doi: 10.1201/9781003338024.
D. Roy and M. Dutta, “A systematic review and research perspective on recommender systems,” J. Big Data, vol. 9, no. 1, pp. 1–36, Dec. 2022, doi: 10.1186/S40537-022-00592-5/FIGURES/6.
F. L. da Silva, B. K. Slodkowski, K. K. A. da Silva, and S. C. Cazella, “A systematic literature review on educational recommender systems for teaching and learning: research trends, limitations and opportunities,” Educ. Inf. Technol., vol. 28, no. 3, pp. 3289–3328, Mar. 2023, doi: 10.1007/S10639-022-11341-9/TABLES/7.
P. C. Sen, M. Hajra, and M. Ghosh, “Supervised Classification Algorithms in Machine Learning: A Survey and Review,” Adv. Intell. Syst. Comput., vol. 937, pp. 99–111, 2020, doi: 10.1007/978-981-13-7403-6_11.
M. Abedini, A. Bijari, T. Banirostam, and A. Professor, “Classification of Pima Indian Diabetes Dataset using Ensemble of Decision Tree, Logistic Regression and Neural Network Staff Data Scientist, General Electric Aviation,” Int. J. Adv. Res. Comput. Commun. Eng., vol. 9, 2020, doi: 10.17148/IJARCCE.2020.9701.
“Utilization of Ensemble Techniques for Prediction of the Academic Performance of Students.” Accessed: Aug. 23, 2024. [Online]. Available: https://www.researchgate.net/publication/361101272_Utilization_of_Ensemble_Techniques_for_Prediction_of_the_Academic_Performance_of_Students
K. Raza, “Improving the prediction accuracy of heart disease with ensemble learning and majority voting rule,” U-Healthcare Monit. Syst. Vol. 1 Des. Appl., pp. 179–196, Jan. 2019, doi: 10.1016/B978-0-12-815370-3.00008-6.
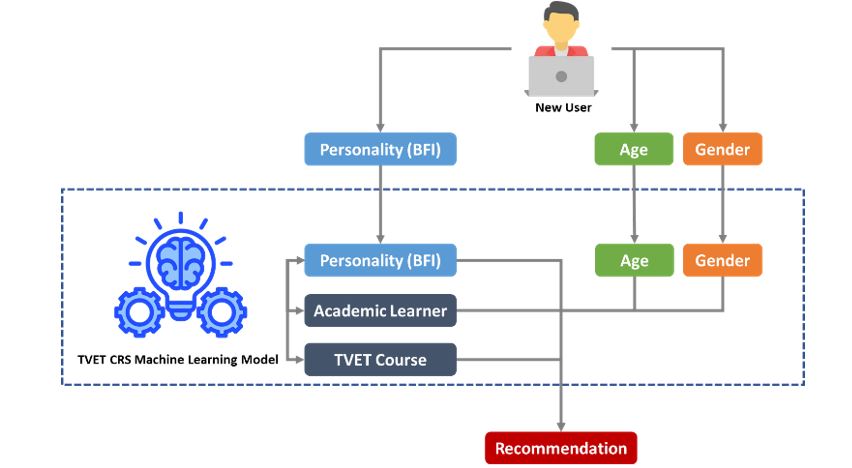
Downloads
Published
How to Cite
Issue
Section
License
Copyright (c) 2024 50SEA

This work is licensed under a Creative Commons Attribution 4.0 International License.