Machine Learning in Livestock Management: A Systematic Exploration of Techniques and Outcomes
Keywords:
Livestock, Animal, Improvement, Productivity, Machine Learning, Deep LearningAbstract
This Systematic Literature Review (SLR) examines the growing field of leveraging Machine Learning (ML) to improve livestock productivity. Through a meticulous analysis of peer-reviewed articles, the study categorizes research into key domains such as disease detection, feed optimization, and reproductive management. Various ML algorithms, including supervised, unsupervised, and reinforcement learning, are evaluated for their efficacy in enhancing herd health and management. The review also addresses the role of diverse data sources, such as sensor technologies and electronic health records and discusses the socio-economic and ethical implications of ML adoption in livestock farming. Insights into scalability, economic viability, and future research directions contribute to a comprehensive understanding of the current background and pave the way for sustainable and technologically advanced livestock management practices. This review serves as a valuable resource for researchers, practitioners, and policymakers in shaping the future of precision agriculture in improving livestock productivity.
References
J. B. and Q. Xie, “Artificial intelligence in animal farming: A systematic literature review,” J. Clean. Prod., vol. 331, p. 129956, 2022.
and M. A. S. Messaoud, A. Bradai, S. H. R. Bukhari, P. T. A. Quang, O. B. Ahmed, “A survey on machine learning in Internet of Things: Algorithms, strategies, and applications,” Internet of Things, vol. 12, p. 100314, 2020.
and K. C. M. Wójcik, P. Jeziorska-Biel, “Between words: A generational discussion about farming knowledge sources,” J. Rural Stud., vol. 67, pp. 130–141, 2019.
M. Balasubramaniyan and C. Navaneethan, “Applications of Internet of Things for smart farming – A survey,” Mater. Today Proc., vol. 47, pp. 18–24, 2021.
B. Ji et al., “A review of measuring, assessing and mitigating heat stress in dairy cattle,” Biosyst. Eng., vol. 190, pp. 4–26, 2020.
and C. D. G. Defalque, R. Santos, M. Pache, “A review on beef cattle supplementation technologies,” Inf. Process. Agric., 2023.
and P. L. N. Kleanthous, A. J. Hussain, W. Khan, J. Sneddon, A. Al-Shamma’a, “A survey of machine learning approaches in animal behaviour,” Neurocomputing, vol. 491, pp. 442–463, 2020.
and A. S. S. D. Alwis, Z. Hou, Y. Zhang, M. H. Na, B. Ofoghi, “A survey on smart farming data, applications and techniques,” Comput. Ind., vol. 138, p. 103624, 2022.
and M. U. K. M. S. Mahmud, A. Zahid, A. K. Das, M. Muzammil, “A systematic literature review on deep learning applications for precision cattle farming,” Comput. Electron. Agric., vol. 187, p. 106313, 2021.
and P. R. R. García, J. Aguilar, M. Toro, A. Pinto, “A systematic literature review on the use of machine learning in precision livestock farming,” Comput. Electron. Agric., vol. 179, p. 105826, 2020.
and J. M. M. E. Hossain, M. A. Kabir, L. Zheng, D. L. Swain, S. McGrath, “A systematic review of machine learning techniques for cattle identification: Datasets, methods and future directions,” Artif. Intell. Agric., vol. 6, pp. 138–155, 2022.
“Abstracts of the 2022 American Dairy Science Association® Annual Meeting,” J. Dairy Sci., vol. 105, pp. 1–474, 2020.
and A. P. R. García, J. Aguilar, “An autonomous system for the self-supervision of animal fattening in the context of precision livestock farming,” Futur. Gener. Comput. Syst., vol. 150, pp. 220–231, 2024.
and P. R. R. García, J. Aguilar, M. Toro, N. Pérez, A. Pinto, “Autonomic computing in a beef-production process for Precision Livestock Farming,” J. Ind. Inf. Integr., vol. 31, p. 100425, 2023.
and L. Z. Y. Zhou, W. Tiemuer, “Bibliometric analysis of smart livestock from 1998-2022,” Procedia Comput. Sci., vol. 214, pp. 1428–1435, 2022.
Dr. M. Alshehri, “Blockchain-assisted internet of things framework in smart livestock farming,” Internet of Things, vol. 22, p. 100739, 2023.
and Y. M. Z. Hou, L. Huang, Q. Zhang, “Body weight estimation of beef cattle with 3D deep learning model: PointNet++,” Comput. Electron. Agric., vol. 213, p. 108184, 2023.
and D. H. Y. Qiao, Y. Guo, K. Yu, “C3D-ConvLSTM based cow behaviour classification using video data for precision livestock farming,” Comput. Electron. Agric., vol. 193, p. 106650, 2022.
and K. K. S. V. Bharti, B. Biswas, “Chapter 5 - Computational intelligence in Internet of things for future healthcare applications,” IoT-Based Data Anal. Healthc. Ind., pp. 57–78, 2021.
B. Beaver and G. Golab, “Chapter 11 - Livestock welfare issues,” Vet. s Guid. to Anim. Welf., pp. 285–338, 2023.
G. Kopper et al., “Chapter 27 - Hygiene in Primary Production,” Food Saf. Manag. (Second Ed. V. Andersen, H. Lelieveld, Y. Motarjemi, Eds., San Diego Acad. Press, pp. 521–585, 2023.
and S. O. M. M. Henchion, C. J. S. De Backer, L. Hudders, “Chapter 30 - Ethical and sustainable aspects of meat production; consumer perceptions and system credibility,” New Asp. Meat Qual. (Second Ed. P. Purslow, Ed., Woodhead Publ. Ser. Food Sci. Technol. Nutr. , Woodhead Publ., pp. 829–851, 2022.
P. Kumar et al., “Chapter 36 - Role of artificial intelligence, sensor technology, big data in agriculture: next-generation farming,” Bioinforma. Agric. P. Sharma, D. Yadav, R. K. Gaur, Eds., Acad. Press, pp. 625–639, 2022.
P. Niloofar et al., “Data-driven decision support in livestock farming for improved animal health, welfare and greenhouse gas emissions: Overview and challenges,” Comput. Electron. Agric., vol. 190, p. 106406, 2021.
S.Neethirajan and B. Kemp, “Digital Livestock Farming,” Sens. Bio-Sensing Res., vol. 32, p. 100408, 2021.
and I. V. R. Lardy, Q. Ruin, “Discriminating pathological, reproductive or stress conditions in cows using machine learning on sensor-based activity data,” Comput. Electron. Agric., vol. 204, p. 107556, 2023.
and V. van Z. E. Jerhamre, C. J. C. Carlberg, “Exploring the susceptibility of smart farming: Identified opportunities and challenges,” Smart Agric. Technol., vol. 2, p. 100026, 2022.
and L. M. M. Cheng, J. Quan, J. Yin, X. Liu, Z. Yuan, “High-resolution maps of intensive and extensive livestock production in China,” Resour. Environ. Sustain., vol. 12, p. 100104, 2023.
and G. M. A. Perrin, M. S. Cristobal, R. Milestad, “Identification of resilience factors of organic dairy cattle farms,” Agric. Syst., vol. 183, p. 102875, 2020.
A. Smith, J., & Williams, “Machine learning in precision livestock farming: Trends and opportunities,” J. Agric. Informatics, vol. 15, no. 3, pp. 145–160, 2024.
Y. Ahmed, K., & Zhou, “IoT-based livestock monitoring and management system using machine learning algorithms,” IEEE Trans. Smart Agric., vol. 12, no. 2, pp. 200–213, 2024.
M. Roberts, L., & Patel, “Applications of machine learning to address complex problems in livestock.,” IEEE Conf. Proceedings., 2024.
S. Hernandez, P., & Taylor, “Machine learning applications for precision agriculture: A comprehensive review.,” IEEE Trans. Precis. Farming, vol. 18, no. 1, pp. 80–95, 2024.
and C. G. M. Durand, C. Largouët, L. Bonneau de Beaufort, J. Y. Dourmad, “A dataset to study group-housed sows’ individual behaviours and production responses to different short-term events,” Anim. - Open Sp., vol. 2, p. 100039, 2023.
N. El-Hage Scialabba, “Chapter 12 - Livestock as a tool to regenerate land,” Manag. Heal. Livest. Prod. Consum., pp. 155–162, 2022.
and J. R. R. D. D. A. Borges Oliveira, L. G. Ribeiro Pereira, T. Bresolin, R. E. Pontes Ferreira, “A review of deep learning algorithms for computer vision systems in livestock,” Livest. Sci., vol. 253, p. 104700, 2021.
K. Mohteshamuddin et al., “A pilot study on the advancement of livestock healthcare bio-capsules and development of customized long-range network in the United Arab Emirates,” Smart Agric. Technol., vol. 3, p. 100082, 2023.
and P. S. N. Goel, Y. Kumar, S. Kaur, M. Sharma, “Chapter 3 - Machine learning-based remote monitoring and predictive analytics system for monitoring and livestock monitoring,” Appl. Mach. Learn. Agric., pp. 47–67, 2022.
U. K. Behera and J. France, “Chapter One - Farming systems research: Concepts, design and methodology,” Adv. Agron. vol. 177, D. L. Sparks, Ed., Acad. Press, pp. 1–49, 2023.
and S. S. Y. Qiao, M. Truman, “Cattle segmentation and contour extraction based on Mask R-CNN for precision livestock farming,” Comput. Electron. Agric., vol. 165, p. 104958, 2019.
and S. K. G. K. R. Singh, S. Dash, “Chapter 15 - Farm automation,” AI, Edge IoT-based Smart Agric., pp. 265–286, 2022.
and L. C. P. A. A. R. de A. Zanella, E. da Silva, “CEIFA: A multi-level anomaly detector for smart farming,” Comput. Electron. Agric., vol. 202, p. 107279, 2022.
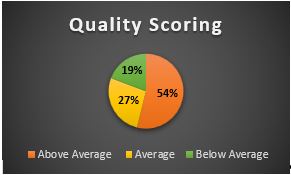
Downloads
Published
How to Cite
Issue
Section
License
Copyright (c) 2024 50sea

This work is licensed under a Creative Commons Attribution 4.0 International License.