Predicting Depression Among Type 2 Diabetic Patients Using Federated Learning
Keywords:
Depression, Federated Learning, Type 2 diabetes, HealthcareAbstract
Depression being a common and dangerous mental health condition could have significant impact on a person's quality of life. It may result in depressive and gloomy feelings along with a loss of interest in once-enjoyable activities. Depression is considered a leading global cause of impairment that affects people at various stages of age, ethnicities, and socioeconomic statuses. It may cause negative effects on person’s physical and emotional well- being like reduced motivation, energy, and appetite. In this paper, we have presented Federated Learning-based framework to predict depression in patients with type 2 diabetes. Type 2 diabetes frequently coexists with depression, which can have a negative impact on treatment outcomes and raise medical expenses. Objective of this paper is to create a Federated Learning- based framework to predict the impact of depression in causing type-II diabetes by analyzing patient’s data that include laboratory results, medical history, and demographic information. To forecast the likelihood of depression in patients with type 2 diabetes. Analysis has been performed using freely available dataset of Type-II diabetes from Kaggle and accuracy of 97% has been achieved.
References
I. M. Cheng, Y. L., Wu, Y. R., Lin, K. D., Lin, C. H. R., & Lin, “Using Machine Learning for the Risk Factors Classification of Glycemic Control in Type 2 Diabetes Mellitus.,” Healthc., vol. 11, no. 8, p. 1141, 2023.
M. Bank, S., & Das, “Periodic study of electrocardiogram abnormalities can predict major adverse cardiac events in Type 2 diabetics.,” Eur. J. Prev. Cardiol., vol. 30, no. 8, pp. 654–655, 2023.
N. Kee, O. T., Harun, H., Mustafa, N., Abdul Murad, N. A., Chin, S. F., Jaafar, R., & Abdullah, “Cardiovascular complications in a diabetes prediction model using Machine Learning: a systematic review.,” Cardiovasc. Diabetol., vol. 22, no. 1, p. 13, 2023.
Y. F. Liu, C. H., Peng, C. H., Huang, L. Y., Chen, F. Y., Kuo, C. H., Wu, C. Z., & Cheng, “The comparison between multiple linear regression and Machine Learning methods in predicting cognitive function in Chinese type 2 diabetes.,” 2023.
Z. Farooq, M. S., Tehseen, R., Qureshi, J. N., Omer, U., Yaqoob, R., Tanweer, H. A., & Atal, “FFM: Flood forecasting model using FL,” IEEE Access, pp. 24472–24483, 2023.
L. Hennebelle, A., Materwala, H., & Ismail, “HealthEdge: a Machine Learning -based smart healthcare framework for prediction of type 2 diabetes in an integrated IoT, edge, and cloud computing system.,” Procedia Comput. Sci., vol. 220, pp. 331–338, 2023.
W. Al Sadi, K., & Balachandran, “Prediction Model of Type 2 Diabetes Mellitus for Oman Prediabetes Patients Using Artificial Neural Network and Six Machine Learning Classifiers,” Appl. Sci., vol. 13, no. 4, p. 2344, 2023.
“Dataset link https://www.kaggle.com/datasets/mathchi/diabetes-data-set”.
K. T. Arrayyan, A. Z., Setiawan, H., & Putra, “Naive Bayes for Diabetes Prediction: Developing a Classification Model for Risk Identification in Specific Populations,” Semesta Tek., vol. 27, no. 1, pp. 28–36, 2024.
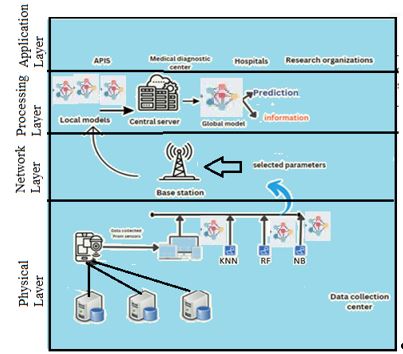
Downloads
Published
How to Cite
Issue
Section
License
Copyright (c) 2024 50sea

This work is licensed under a Creative Commons Attribution 4.0 International License.