AI-Driven Control and Processing System for Smart Homes with Solar Energy
Keywords:
Consumer-based load profiles, load curves, solar capacity, AI Energy SystemsAbstract
In recent years, the utilization of solar energy has grabbed attention in the industrial and domestic zones. The existing systems to use the services of solar cells are conventional. These systems require parameters (irradiance and temperature) for desirable results that are unknown to the end user. These parameters change with regions and human to human. Therefore, an Artificially Intelligent, Control and Processing System is designed to get more accurate results with the unique feature of empowering the end user, which uses the parameters assembled on different regions. The proposed system has an improved PV model based on (ANN) that resembles experimental results with a few readily available, reprogrammable input parameters from the PV module datasheet. The developed system uses regional irradiation data which exhibits minimal fluctuations. In the model presented here; to avoid overburdening problems, loads were divided into manageable chunks MK. In this case load chunks (needed) were moved from solar to utility more stably and economically. Briefly stated the suggested solution provides a complete package for integrating solar energy systems with the grid in an automated and resilient way.
References
M. Rashid, R. Shakoor, and A. Raheem, "An efficient 3D simulator for optimal wind farm modelling and simulation using Particle Swarm Optimization (PSO) Algorithm," Mehran University Research Journal Of Engineering & Technology, vol. 40, no. 4, pp. 809-823, 2021.
P. Bouquet, I. Jackson, M. Nick, and A. Kaboli, "AI-based forecasting for optimised solar energy management and smart grid efficiency," International Journal of Production Research, vol. 62, no. 13, pp. 4623-4644, 2024.
Y. Li and R. Wang, "Novel Energy Systems for Smart Houses," in China and Europe’s Partnership for a More Sustainable World: Emerald Group Publishing Limited, 2016, pp. 283-295.
R. Charron, "A review of design processes for low energy solar homes," Open house international, vol. 33, no. 3, pp. 7-16, 2008.
P. Neetzow, R. Mendelevitch, and S. Siddiqui, "Modeling coordination between renewables and grid: Policies to mitigate distribution grid constraints using residential PV-battery systems," Energy Policy, vol. 132, pp. 1017-1033, 2019.
S. B. Slama and M. Mahmoud, "A deep learning model for intelligent home energy management system using renewable energy," Engineering Applications of Artificial Intelligence, vol. 123, p. 106388, 2023.
I. D. de Cerio Mendaza, I. G. Szczesny, J. R. Pillai, and B. Bak-Jensen, "Demand response control in low voltage grids for technical and commercial aggregation services," IEEE Transactions on Smart Grid, vol. 7, no. 6, pp. 2771-2780, 2015.
A. Alzoubi, "Machine learning for intelligent energy consumption in smart homes," International Journal of Computations, Information and Manufacturing (IJCIM), vol. 2, no. 1, 2022.
T.-L. Chen, T.-C. Kang, C.-Y. Chang, T.-C. Hsiao, and C.-C. Chen, "Smart Home Power Management Based on Internet of Things and Smart Sensor Networks," Sensors & Materials, vol. 33, 2021.
A. Baliyan, I. J. Mwakitalima, M. Jamil, and M. Rizwan, "Intelligent Energy Management System for a Smart Home Integrated with Renewable Energy Resources," International Journal of Photoenergy, vol. 2022, 2022.
A. Raza, L. Jingzhao, Y. Ghadi, M. Adnan, and M. Ali, "Smart home energy management systems: Research challenges and survey," Alexandria Engineering Journal, vol. 92, pp. 117-170, 2024.
S. Lee and D.-H. Choi, "Reinforcement learning-based energy management of smart home with rooftop solar photovoltaic system, energy storage system, and home appliances," Sensors, vol. 19, no. 18, p. 3937, 2019.
A. Dhage, A. Kakade, G. Nahar, M. Pingale, S. Sonawane, and A. Ghotkar, "Recommendation and Prediction of Solar energy consumption for smart homes using machine learning algorithms," in 2021 International Conference on Artificial Intelligence and Machine Vision (AIMV), 2021: IEEE, pp. 1-5.
K. Stecuła, R. Wolniak, and W. W. Grebski, "AI-Driven urban energy solutions—from individuals to society: a review," Energies, vol. 16, no. 24, p. 7988, 2023.
S. S. Binyamin, S. A. B. Slama, and B. Zafar, "Artificial intelligence-powered energy community management for developing renewable energy systems in smart homes," Energy Strategy Reviews, vol. 51, p. 101288, 2024.
N. Raj, A. Sinha, S. Bharddwaj, and A. L. Yadav, "AI-Powered Energy Consumption Optimization for Smart Homes Using IoT," in 2024 International Conference on Computational Intelligence and Computing Applications (ICCICA), 2024, vol. 1: IEEE, pp. 231-236.
H. Mohammed Ridha, C. Gomes, H. Hizam, and M. Ahmadipour, "Optimal design of standalone photovoltaic system based on multi-objective particle swarm optimization: a case study of Malaysia," Processes, vol. 8, no. 1, p. 41, 2020.
H. Chen, S. Jiao, M. Wang, A. A. Heidari, and X. Zhao, "Parameters identification of photovoltaic cells and modules using diversification-enriched Harris hawks optimization with chaotic drifts," Journal of Cleaner Production, vol. 244, p. 118778, 2020.
R. Ahmed, V. Sreeram, Y. Mishra, and M. Arif, "A review and evaluation of the state-of-the-art in PV solar power forecasting: Techniques and optimization," Renewable and Sustainable Energy Reviews, vol. 124, p. 109792, 2020.
D. A. Brodén, K. Paridari, and L. Nordström, "Matlab applications to generate synthetic electricity load profiles of office buildings and detached houses," in 2017 IEEE Innovative Smart Grid Technologies-Asia (ISGT-Asia), 2017: IEEE, pp. 1-6.
Y. Zhou, N. Zhou, L. Gong, and M. Jiang, "Prediction of photovoltaic power output based on similar day analysis, genetic algorithm and extreme learning machine," Energy, vol. 204, p. 117894, 2020.
V. P. Singh and K. Vaibhav, "Solar Power forecasting: The State-Of-The-Art," Power Research-A Journal of CPRI, pp. 367-372, 2014.
S. Bifaretti, A. Bellini, V. Iacovone, and C. Cornaro, "Simplified model of a photovoltaic module," Applied electronics, 2009.
B. P. Bhattarai et al., "Design and cosimulation of hierarchical architecture for demand response control and coordination," IEEE Transactions on Industrial Informatics, vol. 13, no. 4, pp. 1806-1816, 2016.
M. Groissböck and A. Gusmão, "Impact of renewable resource quality on security of supply with high shares of renewable energies," Applied Energy, vol. 277, p. 115567, 2020.
M. A. A. Mamun, M. Hasanuzzaman, and J. Selvaraj, "Experimental investigation of the effect of partial shading on photovolt performance," IET Renewable Power Generation, vol. 11, no. 7, pp. 912-921, 2017.
I. García, M. Victoria, and I. Antón, "Temperature effects on CPV solar cells, optics and modules," Handbook of Concentrator Photovoltaic Technology, pp. 245-292, 2016.
R. Ayaz, I. Nakir, and M. Tanrioven, "An Improved Matlab‐Simulink Model of PV Module considering Ambient Conditions," International Journal of Photoenergy, no. 1, p. 315893, 2014.
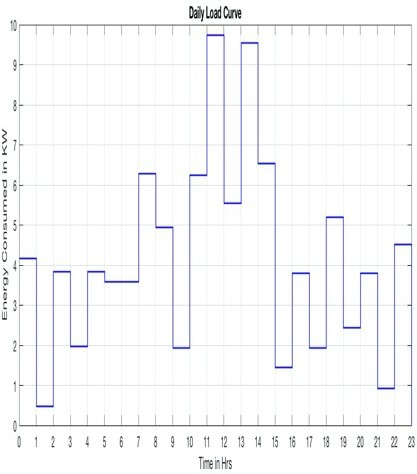
Downloads
Published
How to Cite
Issue
Section
License
Copyright (c) 2024 50sea

This work is licensed under a Creative Commons Attribution 4.0 International License.