Crypto Currency Compensation Model to Detect Optimal Channel of Internet of Things Through Blockchain
Keywords:
Blockchain, Internet of Things, Cryptocurrency, Optimal Automated Multi ResolutionAbstract
The ever-growing number of belongings of internet (IoT) devices in civilization creates a reliable, accessible, and safe infrastructure for processing the calculated data. One-point failures result from the prevalent IoT version's use of an imperative cloud server approach. Because Blockchain uses a distributed community, IoT is integrated with Blockchain generation to avoid this. Consequently, this study has developed a fully autonomous and self-regulating learning system that can accurately operate channel time/spectral characteristics to communicate multi-user statistics. The future system is distinct in that it uses community metrics as its primary basis to recognize and adjust to increasing community density. Following the extraction of those capabilities, the projected protocol efficiently selects the appropriate channel for incoming nodes based on its interval features, recognizes and allocates the idle spectrum of nearby channels, and provides the optimal and appropriate channel utilization through an article called multilevel Gaussian radial and a multilayer non-linear assist vector machine (SVM) type model. The value consumption rate of the secure network and its functionalities is calculated in order to assess the performance of the proposed system. Future and conventional systems are compared. Associated to the prior model, the accuracy of the current model is 95.6%.
References
Abbas, Z., & Yoon, W. (2015). A survey on energy conserving mechanisms for the internet of things: Wireless networking aspects. Sensors, 15(10), 24818-24847.
Abu-Elkheir, M., Hayajneh, M., & Ali, N. A. (2013). Data management for the internet of things: Design primitives and solution. Sensors, 13(11), 15582-15612.
Ahuja, R. K., Mehlhorn, K., Orlin, J., & Tarjan, R. E. (1990). Faster algorithms for the shortest path problem. Journal of the ACM (JACM), 37(2), 213-223.
Akyildiz, I. F., Melodia, T., & Chowdhury, K. R. (2007). A survey on wireless multimedia sensor networks. Computer networks, 51(4), 921-960.
Alfandi, O., Khanji, S., Ahmad, L., & Khattak, A. (2021). A survey on boosting IoT security and privacy through blockchain. Cluster Computing, 24(1), 37-55.
An, J., Yin, L., Shang, Y., Zhong, Y., Zhang, X., Wu, M., . . . Huang, Y. (2011). The combined effects of BDE47 and BaP on oxidatively generated DNA damage in L02 cells and the possible molecular mechanism. Mutation Research/Genetic Toxicology and Environmental Mutagenesis, 721(2), 192-198.
Aslam, S., Hasan, N. U., Jang, J. W., & Lee, K.-G. (2016). Optimized energy harvesting, cluster-head selection and channel allocation for IoTs in smart cities. Sensors, 16(12), 2046.
Azmat, F., Chen, Y., & Stocks, N. (2015). Analysis of spectrum occupancy using machine learning algorithms. IEEE Transactions on Vehicular Technology, 65(9), 6853-6860.
Banafa, A. (2017). IoT and blockchain convergence: benefits and challenges. IEEE Internet of Things, 9.
Campbell, J., Gibbons, P. B., Nath, S., Pillai, P., Seshan, S., & Sukthankar, R. (2005). Irisnet: an internet-scale architecture for multimedia sensors. Paper presented at the Proceedings of the 13th annual ACM international conference on Multimedia.
Chen, Y., & Oh, H.-S. (2014). A survey of measurement-based spectrum occupancy modeling for cognitive radios. IEEE Communications Surveys & Tutorials, 18(1), 848-859.
Choi, N., & Kim, H. (2019). A Blockchain-based user authentication model using MetaMask. Journal of Internet Computing and Services, 20(6), 119-127.
Del Re, E., Fantacci, R., & Ronga, L. (1996). A dynamic channel allocation technique based on Hopfield neural networks. IEEE Transactions on Vehicular Technology, 45(1), 26-32.
Digham, F. F. (2008). Joint power and channel allocation for cognitive radios. Paper presented at the 2008 IEEE Wireless Communications and Networking Conference.
Ding, G., Wu, Q., & Wang, J. (2013). Sensing confidence level-based joint spectrum and power allocation in cognitive radio networks. Wireless personal communications, 72(1), 283-298.
Hassan, T., Aslam, S., & Jang, J. W. (2018). Fully Automated Multi-Resolution Channels and Multithreaded Spectrum Allocation Protocol for IoT Based Sensor Nets. IEEE Access, 6, 22545-22556. doi: 10.1109/access.2018.2829078
Hoang, A. T., & Liang, Y.-C. (2006). Maximizing spectrum utilization of cognitive radio networks using channel allocation and power control. Paper presented at the IEEE Vehicular Technology Conference.
Huang, C., Fu, Y., Zhong, M., & Yin, C. (2011). Multi-channel Wireless Sensor Network MAC protocol based on dynamic route. Paper presented at the 2011 International Conference on Multimedia Technology.
Lee, J., & Park, H.-K. (2014). Channel prediction-based channel allocation scheme for multichannel cognitive radio networks. Journal of communications and networks, 16(2), 209-216.
Letaief, K. B., & Zhang, W. (2009). Cooperative communications for cognitive radio networks. Proceedings of the IEEE, 97(5), 878-893.
Li, X., Jiang, P., Chen, T., Luo, X., & Wen, Q. (2020). A survey on the security of blockchain systems. Future Generation Computer Systems, 107, 841-853.
McBee, M. P., & Wilcox, C. (2020). Blockchain technology: principles and applications in medical imaging. Journal of digital imaging, 33(3), 726-734.
Miao, G., Li, G. Y., & Swami, A. (2009). Decentralized optimization for multichannel random access. IEEE Transactions on Communications, 57(10), 3012-3023.
Nasipuri, A., & Das, S. R. (1999). On-demand multipath routing for mobile ad hoc networks. Paper presented at the Proceedings Eight International Conference on Computer Communications and Networks (Cat. No. 99EX370).
Patra, S. S. M., Roy, K., Banerjee, S., & Vidyarthi, D. P. (2006). Improved genetic algorithm for channel allocation with channel borrowing in mobile computing. IEEE Transactions on Mobile Computing, 5(7), 884-892.
Pilkington, M. (2016). Blockchain technology: principles and applications Research handbook on digital transformations: Edward Elgar Publishing.
Smart, G., Deligiannis, N., Surace, R., Loscri, V., Fortino, G., & Andreopoulos, Y. (2015). Decentralized time-synchronized channel swapping for ad hoc wireless networks. IEEE Transactions on Vehicular Technology, 65(10), 8538-8553.
Upadhyayula, S., Annamalai, V., & Gupta, S. K. (2003). A low-latency and energy-efficient algorithm for convergecast in wireless sensor networks. Paper presented at the GLOBECOM'03. IEEE Global Telecommunications Conference (IEEE Cat. No. 03CH37489).
Wang, F., Zhu, J., Huang, J., & Zhao, Y. (2010). Admission control and channel allocation for supporting real-time applications in cognitive radio networks. Paper presented at the 2010 IEEE Global Telecommunications Conference GLOBECOM 2010.
Yaga, D., Mell, P., Roby, N., & Scarfone, K. (2019). Blockchain technology overview. arXiv preprint arXiv:1906.11078.
Yen, H.-H. (2009). Optimization-based channel constrained data aggregation routing algorithms in multi-radio wireless sensor networks. Sensors, 9(6), 4766-4788.
Yu, R., Zhong, W., Xie, S., Zhang, Y., & Zhang, Y. (2015). QoS differential scheduling in cognitive-radio-based smart grid networks: An adaptive dynamic programming approach. IEEE transactions on neural networks and learning systems, 27(2), 435-443.
Zhao, D., Huang, Z., Umino, N., Hasegawa, A., & Kanamori, H. (2011). Structural heterogeneity in the megathrust zone and mechanism of the 2011 Tohoku‐oki earthquake (Mw 9.0). Geophysical Research Letters, 38(17).
Zomaya, A. Y., & Wright, M. (2002). Observations on using genetic-algorithms for channel allocation in mobile computing. IEEE Transactions on Parallel and Distributed Systems, 13(9), 948-962.
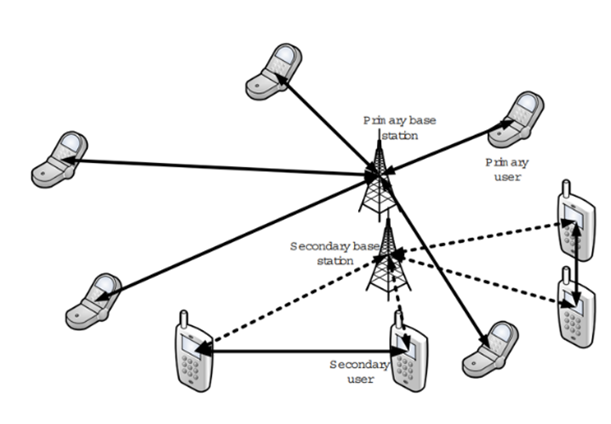
Downloads
Published
How to Cite
Issue
Section
License
Copyright (c) 2025 50SEA

This work is licensed under a Creative Commons Attribution 4.0 International License.