Exploring Contextual Similarity in Quranic Ayahs: A Case Study of Surah Al-Baqarah and Aal-e-Imran in Urdu Translations
Keywords:
Contextual Similarity, Quranic Translation, LSTM Model, Urdu Translation Surah Al-Baqarah and Aal-e-ImranAbstract
The translation of sacred texts, particularly the Quran, requires a deep understanding of both linguistic and contextual nuances to preserve the original message. This research investigates the contextual similarity among Quranic Ayahs by analyzing the Urdu translations of Surah Al-Baqarah and Aal-e-Imran from Maulana Maududi's Urdu Quranic translation. Given the importance of accurately conveying the essence of the original Arabic text, this study aims to quantify the contextual relationships between Ayahs within each Surah and assess the effectiveness of Maulana Maududi’s translation in maintaining these relationships. The novelty of this study lies in its application of deep learning, particularly Long Short-Term Memory (LSTM) networks, to evaluate the contextual similarity between Ayahs. The LSTM model is used to capture the deep linguistic and contextual relationships within the translation, offering a data-driven approach to Quranic translation evaluation. The dataset comprises the complete translations of Surah Al-Baqarah and Aal-e-Imran in Urdu, and each Ayah is compared with every other Ayah within the same Surah to compute similarity scores. The results show varying degrees of similarity among Ayahs, with some Ayahs exhibiting high contextual alignment while others display subtle divergences. These findings highlight the ability of LSTM models to uncover hidden patterns in translation, while also pointing out the challenges in preserving the full contextual integrity of the original Arabic text in translation. In conclusion, this study provides valuable insights into the complexities of Quranic translation and offers a novel approach to evaluating the quality of such translations. By combining advanced machine learning techniques with the study of sacred texts, it presents a new avenue for improving the accuracy and contextual coherence of Quranic translations, ultimately contributing to the field of computational linguistics and religious studies.
References
M. Alshammeri, “Deep Learning and Distributional Semantics for the Qur’an” “University of Leeds, Department of Linguistics,” Univ. Leeds, Dep. Linguist., 2022.
A. Alsaleh, M., Al-Khalifa, H. S., & Al-Ajlan, “Utilizing AraBERT for Semantic Search in Quranic Texts,” Proc. 12th Int. Conf. Islam. Appl. Comput. Sci. Technol., 2021.
E. A. Tall, M., Diop, T. I., Ngom, N. F., & Thiam, “Deep Learning for Quranic Reciter Recognition and Audio Content Identification,” Proc. 6th Int. Conf. Arab. Comput. Linguist., 2021.
A. M. Alashqar, “A Classification of Quran Verses Using Deep Learning,” Int. J. Comput. Digit. Syst., vol. 16, no. 1, pp. 1041–1053, Jul. 2024, doi: 10.12785/IJCDS/160176.
W. Antoun, F. Baly, and H. Hajj, “AraBERT: Transformer-based Model for Arabic Language Understanding,” 2020. Accessed: Jan. 10, 2025. [Online]. Available: https://aclanthology.org/2020.osact-1.2/
A. Zadeh, S., & Shahraki, “Contextual Analysis of Quranic Translations Using Deep Learning Models,” J. Quranic Stud. Comput. Linguist. “Department Comput. Linguist., 2022.
Y. Liu, Y., Li, Q., & Zhang, “Comparing Translations with LSTM: A Case Study on Quranic Texts,” Dep. Mach. Learn., 2021.
T. Wolf et al., “Transformers: State-of-the-Art Natural Language Processing,” EMNLP 2020 - Conf. Empir. Methods Nat. Lang. Process. Proc. Syst. Demonstr., pp. 38–45, 2020, doi: 10.18653/V1/2020.EMNLP-DEMOS.6.
J. Henderson, “The Unstoppable Rise of Computational Linguistics in Deep Learning,” Proc. Annu. Meet. Assoc. Comput. Linguist., pp. 6294–6306, 2020, doi: 10.18653/V1/2020.ACL-MAIN.561.
M. Ghanem, A., Zaki, S., & Ahmed, “Deep Learning Models for Thematic Pattern Extraction in Quranic Texts” Journal of Artificial Intelligence,” Dep. Artif. Intell., 2020.
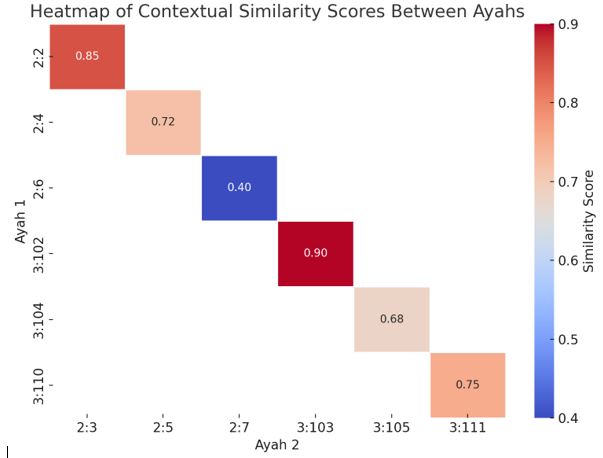
Downloads
Published
How to Cite
Issue
Section
License
Copyright (c) 2024 50SEA

This work is licensed under a Creative Commons Attribution 4.0 International License.