Heart Sense: A novel IoT integrated Deep Learning Based ECG Image Analysis for Enhanced Heart Disease Prediction
Keywords:
Cardiovascular Disease Prediction, IOT, sensors, Deep Learning, Transformer, ECG Images, Heart disease predictionAbstract
The increasing advancements in the healthcare networks leveraging the unmatched capabilities of the Internet of Things for various fatal disease prediction and remote health monitoring that proved to be very beneficial in providing timely and accurate healthcare services to patients. Patients who are suffering from chronic diseases like blood pressure, kidney diseases, and heart diseases need treatment on time to avoid sudden deaths due to these ailments. To avoid this serious scenario, we have presented a novel approach for predicting heart diseases based on the Internet of Things. By leveraging the combined abilities of The IoT and Deep learning we have proposed an advanced approach that will able to predict heart diseases with increased accuracy and precision in comparison to the existing approaches along with providing timely notifications to both patients and the medical professionals to deal with the situation at hand most effectively.
We will be receiving real-time health data from the sensors which will be a wearable IoT device in our case. This collected data contains the continuously monitored information of the patient’s ECG using an ECG sensing system that is sent to the cloud for precise disease prediction. We will also be employing the patients ‘electronic health records which will contain ECG images to increase the accuracy of our results. The Deep Learning model called the transformer will be used in the proposed approach for the precise prediction of cardiovascular disease in real-time. Both the healthcare professionals and the patients are provided with the relevant information if an ailment is predicted for effective healthcare monitoring and treatment. The proposed model has better results than the existing approaches for the prediction of heart disease in terms of accuracy which is 99.8%.
References
J. S. Lucena, “Sudden cardiac death,” Forensic Sci Res, vol. 4, no. 3, pp. 199–201, 2019, doi: 10.1080/20961790.2019.1622062.
R. J. T. Philip A. Ades, ∙ Steven J. Keteyian, Janet S. Wright, Kimberly Newlin, Donald S. Shepard, “Increasing Cardiac Rehabilitation Participation From 20% to 70%: A Road Map From the Million Hearts Cardiac Rehabilitation Collaborative,” Mayo Clin Proc, vol. 92, no. 2, pp. 234–242, 2017, [Online]. Available: https://www.mayoclinicproceedings.org/article/S0025-6196(16)30648-6/fulltext
R. M. Quanhe Yang, Keming Yuan, Edward W. Gregg, Jing Fang, Yuling Hong, “Trends and Clustering of Cardiovascular Health Metrics Among U.S. Adolescents 1988–2010,” J. Adolesc. Heal., vol. 55, no. 4, pp. 513–520, 2014, [Online]. Available: https://www.jahonline.org/article/S1054-139X(14)00159-1/fulltext
M. C. Sophia Greer, Linda J. Schieb, “County Health Factors Associated with Avoidable Deaths from Cardiovascular Disease in the United States, 2006–2010,” Public Health Rep., vol. 131, no. 3, 2016, doi: https://doi.org/10.1177/0033354916131003.
J. P. A.-S. Josue R. Velázquez-González, Hayde Peregrina-Barreto, Jose J. Rangel-Magdaleno, Juan M. Ramirez-Cortes, “ECG-Based Identification of Sudden Cardiac Death through Sparse Representations,” Sensors, vol. 21, no. 22, p. 7666, 2021, doi: https://doi.org/10.3390/s21227666.
P. C. M. Veazie, C. Ayala, L. Schieb, S. Dai, J. A. Henderson, “Trends and Disparities in Heart Disease Mortality Among American Indians/Alaska Natives, 1990–2009,” Am. J. Public Health, 2014, doi: 10.2105/AJPH.2013.301715.
M. B. E. Santhi K. Ganesh, Themistocles L. Assimes, Craig T. Basson, Aravinda Chakravarti, Patrick T. Ellinor, “Genetics and Genomics for the Prevention and Treatment of Cardiovascular Disease: Update: A Scientific Statement From the American Heart Association,” Circulation, vol. 128, no. 25, 2013, doi: https://doi.org/10.1161/01.cir.0000437913.98912.1d.
F. L. Vanessa L. Short, Tameka Ivory-Walls, Larry Smith, “The Mississippi Delta Cardiovascular Health Examination Survey: Study Design and Methods,” Epidemiol. Res. Int., 2014, doi: https://doi.org/10.1155/2014/861461.
Omer Deperlioglu, Utku Kose, Deepak Gupta and A. K. S. Ashish Khanna, “Diagnosis of heart diseases by a secure Internet of Health Things system based on Autoencoder Deep Neural Network,” Comput. Commun., vol. 162, pp. 31–50, 2020, doi: https://doi.org/10.1016/j.comcom.2020.08.011.
M. R. Maurya et al., “A review of smart sensors coupled with Internet of Things and Artificial Intelligence approach for heart failure monitoring,” Med. Biol. Eng. Comput. 2021 5911, vol. 59, no. 11, pp. 2185–2203, Oct. 2021, doi: 10.1007/S11517-021-02447-2.
E. T. Tan and Z. A. Halim, “Health care Monitoring System and Analytics Based on Internet of Things Framework,” IETE J. Res., vol. 65, no. 5, pp. 653–660, Sep. 2019, doi: 10.1080/03772063.2018.1447402.
S. S. Sarmah, “An Efficient IoT-Based Patient Monitoring and Heart Disease Prediction System Using Deep Learning Modified Neural Network,” IEEE Access, vol. 8, pp. 135784–135797, 2020, doi: 10.1109/ACCESS.2020.3007561.
A. Kishor and W. Jeberson, “Diagnosis of Heart Disease Using Internet of Things and Machine Learning Algorithms,” Lect. Notes Networks Syst., vol. 203 LNNS, pp. 691–702, 2021, doi: 10.1007/978-981-16-0733-2_49.
Shivi Chaturvedi, “Clinical Prediction on ML based Internet of Things for E-Health Care System,” Int. J. Data Informatics Intell. Comput., vol. 2, no. 3, 2023, doi: https://doi.org/10.59461/ijdiic.v2i3.76.
E. Kristiani, C. T. Yang, C. Y. Huang, P. C. Ko, and H. Fathoni, “On construction of sensors, edge, and cloud (isec) framework for smart system integration and applications,” IEEE Internet Things J., vol. 8, no. 1, pp. 309–319, Jan. 2021, doi: 10.1109/JIOT.2020.3004244.
Y. Sasaki, “A Survey on IoT Big Data Analytic Systems: Current and Future,” IEEE Internet Things J., vol. 9, no. 2, pp. 1024–1036, 2022, doi: 10.1109/JIOT.2021.3131724.
W. L. Nana Zhang, Di Wang, Jie Wang, Hong Fang, Bin He, Jinrui Guo, Yue Han, Peng Zhang, Chaoqun Shi, Yanan Chen, Qixiang Wang, Miaojuan Ren, “Enhanced Piezoresponse and Dielectric Properties for Ba1-XSrXTiO3 Composition Ultrathin Films by the High-Throughput Method,” Coatings, vol. 11, no. 12, p. 1491, 2021, doi: https://doi.org/10.3390/coatings11121491.
F. Sun, W. Zang, H. Huang, I. Farkhatdinov, and Y. Li, “Accelerometer-Based Key Generation and Distribution Method for Wearable IoT Devices,” IEEE Internet Things J., vol. 8, no. 3, pp. 1636–1650, Feb. 2021, doi: 10.1109/JIOT.2020.3014646.
H. Wang and I. Park, “A Compact Series-Fed Two-Element Dipole Antenna,” 2021 IEEE Int. Symp. Antennas Propag. North Am. Radio Sci. Meet. APS/URSI 2021 - Proc., pp. 939–940, 2021, doi: 10.1109/APS/URSI47566.2021.9704428.
W. Li, G. G. Wang, and A. H. Gandomi, “A Survey of Learning-Based Intelligent Optimization Algorithms,” Arch. Comput. Methods Eng. 2021 285, vol. 28, no. 5, pp. 3781–3799, Feb. 2021, doi: 10.1007/S11831-021-09562-1.
A. S. Albahri et al., “IoT-based telemedicine for disease prevention and health promotion: State-of-the-Art,” J. Netw. Comput. Appl., vol. 173, p. 102873, Jan. 2021, doi: 10.1016/J.JNCA.2020.102873.
“Trends in Passive IoT Biomarker Monitoring and Machine Learning for Cardiovascular Disease Management in the U.S. Elderly Population,” Adv. Geriatr. Med. Res., 2023, doi: 10.20900/AGMR20230002.
M. T. Yuxuan Liao, Zhong Tang, Kun Gao, “Optimization of resources in intelligent electronic health systems based on internet of things to predict heart diseases via artificial neural network,” Heliyon, vol. 10, no. 11, p. e32090, 2024, [Online]. Available: https://www.cell.com/heliyon/fulltext/S2405-8440(24)08121-0?_returnURL=https%3A%2F%2Flinkinghub.elsevier.com%2Fretrieve%2Fpii%2FS2405844024081210%3Fshowall%3Dtrue
H. S. Y. Liu, J. Wang, J. Li, S. Niu, “Machine Learning for the Detection and Identification of Internet of Things Devices: A Survey,” IEEE Internet Things J., vol. 9, no. 1, pp. 298–320, 2022, doi: 10.1109/JIOT.2021.3099028.
S. K. R. F. Mansour, A. E. Amraoui, I. Nouaouri, V. G. Díaz, D. Gupta, “Artificial Intelligence and Internet of Things Enabled Disease Diagnosis Model for Smart Healthcare Systems,” IEEE Access, vol. 9, pp. 45137–45146, 2021, doi: 10.1109/ACCESS.2021.3066365.
Z. L. Zengchen Yu, Ke Wang, Zhibo Wan, Shuxuan Xie, “Popular deep learning algorithms for disease prediction: a review,” Cluster Comput., vol. 26, pp. 1231–1251, 2023, doi: https://doi.org/10.1007/s10586-022-03707-y.
M. A. K. Muhammad Farrukh Khan, Taher M. Ghazal, Raed A. Said, Areej Fatima, Sagheer Abbas, M.A. Khan, Ghassan F. Issa, Munir Ahmad, “An IoMT-Enabled Smart Healthcare Model to Monitor Elderly People Using Machine Learning Technique,” Comput. Intell. Med. Internet Things Appl., 2021, doi: https://doi.org/10.1155/2021/2487759.
T. C. L. tclee@rcsi. i. Richard B. Reilly, “Electrograms (ECG, EEG, EMG, EOG),” Technol. Heal. Care, vol. 18, no. 6, 2010, doi: https://doi.org/10.3233/THC-2010-0604.
F. L. J. Fang, K. Yuan, R. M. Gindi, B. W. Ward, C. Ayala, “Association of Birthplace and Coronary Heart Disease and Stroke Among US Adults: National Health Interview Survey, 2006 to 2014,” J Am Hear. Assoc, vol. 7, no. 7, 2018, doi: https://doi.org/10.1161/JAHA.117.008153.
R. G. Ying Yang, Zengwu Wang, Zuo Chen, Xin Wang, Linfeng Zhang, Suning Li, Congyi Zheng, Yuting Kang, Linlin Jiang, Zhenhui Zhu, “Current status and etiology of valvular heart disease in China: a population-based survey,” BMC Cardiovasc. Disord., vol. 21, no. 339, 2021, doi: https://doi.org/10.1186/s12872-021-02154-8.
Sarah A. Alzakari, Amir Abdel Menaem , Nadir Omer, Amr Abozeid , Loay F. Hussein, and A. R. Islam Abdalla Mohamed Abass, “Enhanced heart disease prediction in remote healthcare monitoring using IoT-enabled cloud-based XGBoost and Bi-LSTM,” Alexandria Eng. J., vol. 105, pp. 280–291, 2024, doi: https://doi.org/10.1016/j.aej.2024.06.036.
V. K. R. Rajaganapathi, R. Mahendran, D. Sivaganesan, Mr. R. Vadivel, M. R. Joel, “An IoT enabled computational model and application development for monitoring cardiovascular risks,” e-Prime - Adv. Electr. Eng. Electron. Energy, vol. 8, p. 100513, 2024, doi: https://doi.org/10.1016/j.prime.2024.100513.
Z. Al-Makhadmeh and A. Tolba, “Utilizing IoT wearable medical device for heart disease prediction using higher order Boltzmann model: A classification approach,” Measurement, vol. 147, p. 106815, Dec. 2019, doi: 10.1016/J.MEASUREMENT.2019.07.043.
A. A. Malibari, “An efficient IoT-Artificial intelligence-based disease prediction using lightweight CNN in healthcare system,” Meas. Sensors, vol. 26, p. 100695, 2023, doi: https://doi.org/10.1016/j.measen.2023.100695.
V. S. S. Satpathy, M. C. Trivedi, S. Malhotra, S. Chaudhary, “FPGA-enhanced IoT methods for disease pre-screening and prediction: An energy optimization approach,” Meas. Sensors, vol. 33, p. 101213, 2024, doi: https://doi.org/10.1016/j.measen.2024.101213.
Sarah A. Alzakari , Amir Abdel Menaem, Nadir Omer, Amr Abozeid, Loay F. Hussein, Islam Abdalla Mohamed Abass, Ayadi Rami and Ahmed Elhadad, “Smart healthcare systems: A new IoT-Fog based disease diagnosis framework for smart healthcare projects,” Alexandria Eng. J., vol. 105, pp. 280–291, 2024, doi: https://doi.org/10.1016/j.aej.2024.06.036.
S. M. S. U. Rehman, I. Sadek, B. Huang, “IoT-Based Emergency Cardiac Death Risk Rescue Alert System,” MethodsX, 2024, doi: 10.1016/j.mex.2024.102834.
I. Ullah, H. Y. Youn, and Y. H. Han, “Integration of type-2 fuzzy logic and Dempster–Shafer Theory for accurate inference of IoT-based health-care system,” Futur. Gener. Comput. Syst., vol. 124, pp. 369–380, Nov. 2021, doi: 10.1016/J.FUTURE.2021.06.012.
S. Tuli et al., “HealthFog: An ensemble deep learning based Smart Healthcare System for Automatic Diagnosis of Heart Diseases in integrated IoT and fog computing environments,” Futur. Gener. Comput. Syst., vol. 104, pp. 187–200, Mar. 2020, doi: 10.1016/J.FUTURE.2019.10.043.
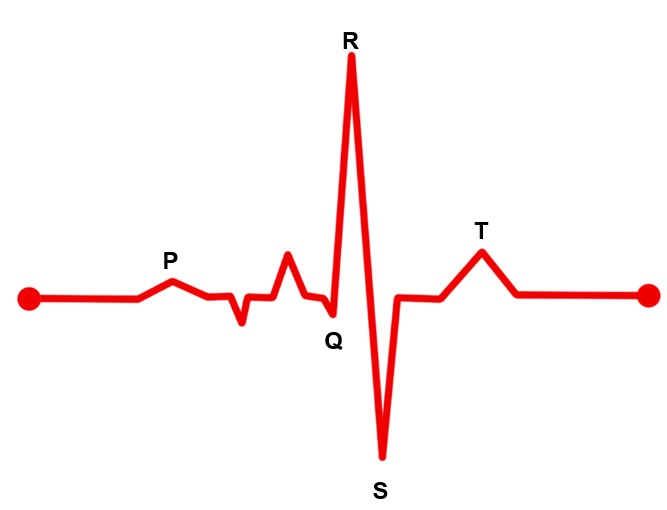
Downloads
Published
How to Cite
Issue
Section
License
Copyright (c) 2025 50SEA

This work is licensed under a Creative Commons Attribution 4.0 International License.