Evaluating Artificial Intelligence and Statistical Methods for Electric Load Forecasting
Keywords:
Electric load forecasting, Power load, Modelling Electricity load, Long term/ Short term forecasting, Performance managementAbstract
Electric Load Forecasting (ELF) is one of the challenges being faced by the Power System industry. With the ever-growing consumer demand, power generating companies struggle to manage and provide an uninterrupted power supply to the users. Over the past few decades, the introduction of smart grids and power deregulation has changed load forecasting dynamics. Most of the current research focuses on short-term load forecasting (STLF), involving an hour to a week’s time forecasting. Various techniques are being used for accurately predicting the electric load. However, gold standards are yet to be defined mainly because of the subject's variety, non-linearity, and un-predictive form. In this study critical review of 25 publications has been carried out to find the most efficient method for ELF. The novelty of this study is that comparative and scientific analyses are carried out to find the most proficient techniques for load forecasting. Also, various parameters are combined for comparison in this study after analyzing published reviews on the subject. Artificial Neural Networks (ANN) and Auto-Regressive Moving Average (ARMA) models outperform other methods basing upon statistical analysis, i.e., Mean Absolute Percentage Error (MAPE) and comparative acceptance, in the research community.
References
Hammad, M. A., Jereb, B., Rosi, B., & Dragan, D. (2020). Methods and Models for Electric Load Forecasting: A Comprehensive Review. Logistics & Sustainable Transport, 11(1), 51-76.
Weron, R. (2014). Electricity price forecasting: A review of the state-of-the-art with a look into the future. International journal of forecasting, 30(4), 1030-1081.
Leung, T. C., Ping, K. P., & Tsui, K. K. (2019). What can deregulators deregulate? The case of electricity. Journal of Regulatory Economics, 56(1), 1-32.
Kuster, C., Rezgui, Y., & Mourshed, M. (2017). Electrical load forecasting models: A critical systematic review. Sustainable cities and society, 35, 257-270.
Zhao, H., & Tang, Z. (2016, June). The review of demand side management and load forecasting in smart grid. In 2016 12th World Congress on Intelligent Control and Automation (WCICA) (pp. 625-629). IEEE.
Zor, K., Timur, O., & Teke, A. (2017, June). A state-of-the-art review of artificial intelligence techniques for short-term electric load forecasting. In 2017 6th International Youth Conference on Energy (IYCE) (pp. 1-7). IEEE.
Almalaq, A., & Edwards, G. (2017, December). A review of deep learning methods applied on load forecasting. In 2017 16th IEEE international conference on machine learning and applications (ICMLA) (pp. 511-516). IEEE.
Upadhaya, D., Thakur, R., & Singh, N. K. (2019, October). A systematic review on the methods of short term load forecasting. In 2019 2nd International Conference on Power Energy, Environment and Intelligent Control (PEEIC) (pp. 6-11). IEEE.
Mohammed, Z. C., Humberto, A. R. J., Kiplel., M. C. & Chepkoech, M. (2017, Dec). A Meta- Analysis on Sustainable Supply Chain Management: An Analytical approach. European Journal of Logistics, Purchasing and Supply Chain Management
Neuman, L. W. (2007). Social research methods, 7/E. Pearson Education India.
Almeshaiei, E., &Soltan, H. (2011). A methodology for electric power load forecasting. Alexandria Engineering Journal, 50(2), 137-144.
Yang, L., & Yang, H. (2019, May). A Combined ARIMA-PPR Model for Short-Term Load Forecasting. In 2019 IEEE Innovative Smart Grid Technologies-Asia (ISGT Asia) (pp. 3363-3367). IEEE.
Huang, S. J., & Shih, K. R. (2003). Short-term load forecasting via ARMA model identification including non-Gaussian process considerations. IEEE Transactions on power systems, 18(2), 673-679.
Amjady, N. (2001). Short-term hourly load forecasti using time-series modeling with peak load estimation capability. IEEE Transactions on power systems, 16(3), 498 505.
Gaur, M., &Majumdar, A. (2017). One-Day-Ahead Load Forecasting using nonlinear Kalman filtering algorithms.
Fan, S., & Hyndman, R. J. (2011). Short-term load forecasting based on a semi-parametric additive model. IEEE Transactions on Power Systems, 27(1), 134-141.
Unsihuay-Vila, C., De Souza, A. Z., Marangon-Lima, J. W., & Balestrassi, P. P. (2010). Electricity demand and spot price forecasting using evolutionary computation combined with chaotic nonlinear dynamic model. International journal of electrical power & energy systems, 32(2), 108-116.
Dong, M., & Grumbach, L. (2019). A Hybrid Distribution Feeder Long-Term Load Forecasting Method Based on Sequence Prediction. IEEE Transactions on Smart Grid, 11(1), 470-482.
Taylor, J. W. (2011). Short-term load forecasting with exponentially weighted methods. IEEE Transactions on Power Systems, 27(1), 458-464.
Taylor, J. W., & McSharry, P. E. (2007). Short-term load forecasting methods: An evaluation based on european data. IEEE Transactions on Power Systems, 22(4), 2213-2219.
Kavanagh, K. (2017). Short Term Demand Forecasting for the Integrated Electricity Market. Student Journal of Energy Research, 2(1), 1.
Zhang, Z., Hong, W. C., & Li, J. (2020). Electric load forecasting by hybrid self-recurrent support vector regression model with variational mode decomposition and improved cuckoo search algorithm. IEEE Access, 8, 14642-14658.
Fan, S., & Chen, L. (2006). Short-term load forecasting based on an adaptive hybrid method. IEEE Transactions on Power Systems, 21(1), 392-401.
Pai, P. F., & Hong, W. C. (2005). Support vector machines with simulated annealing algorithms in electricity load forecasting. Energy Conversion and Management, 46(17), 2669-2688.
Ribeiro, G. T., Mariani, V. C., & dos Santos Coelho, L. (2019). Enhanced ensemble structures using wavelet neural networks applied to short-term load forecasting. Engineering Applications of Artificial Intelligence, 82, 272-281.
Reis, A. R., & Da Silva, A. A. (2005). Feature extraction via multiresolution analysis for short term load forecasting. IEEE Transactions on power systems, 20(1), 189-198.
Lv, Y. C., Xu, X., Xu, R. L., &Ren, H. (2019, January). Research on Short-Term Load Forecasting Approach for Smart Grid. In 2019 International Conference on Intelligent Transportation, Big Data & Smart City (ICITBS) (pp. 602-605). IEEE.
Hamid, M. A., & Rahman, T. A. (2010, March). Short term load forecasting using an artificial neural network trained by artificial immune system learning algorithm. In 2010 12th International Conference on Computer Modelling and Simulation (pp. 408-413). IEEE.
Hippert, H. S., Bunn, D. W., & Souza, R. C. (2005). Large neural networks for electricity load forecasting: Are they overfitted?. International Journal of forecasting, 21(3), 425-434.
Michanos, S. P., Tsakoumis, A. C., Fessas, P., Vladov, S. S., &Mladenov, V. M. (2003, July). Short-term load forecasting using a chaotic time series. In Signals, Circuits and Systems, 2003. SCS 2003. International Symposium on (Vol. 2, pp. 437-440). IEEE.
Wang, Y., Zhang, N., Tan, Y., Hong, T., Kirschen, D. S., & Kang, C. (2018). Combining probabilistic load forecasts. IEEE Transactions on Smart Grid, 10(4), 3664-3674.
Li, S., Wang, P., & Goel, L. (2015). A novel wavelet-based ensemble method for short-term load forecasting with hybrid neural networks and feature selection. IEEE Transactions on power systems, 31(3), 1788-1798.
Charytoniuk, W., & Chen, M. S. (2000). Very short-term load forecasting using artificial neural networks. IEEE transactions on Power Systems, 15(1), 263-268.
Dong, X., Qian, L., & Huang, L. (2017, February). Short-term load forecasting in smart grid: A combined CNN and K-means clustering approach. In 2017 IEEE International Conference on Big Data and Smart Computing (BigComp) (pp. 119-125). IEEE.
Din, G. M. U., &Marnerides, A. K. (2017, January). Short term power load forecasting using deep neural networks. In 2017 International Conference on Computing, Networking and Communications (ICNC) (pp. 594-598). IEEE.
Chen, K., Chen, K., Wang, Q., He, Z., Hu, J., & He, J. (2018). Short-term load forecasting with deep residual networks. IEEE Transactions on Smart Grid, 10(4), 3943-3952.
Chen, Y., Luh, P. B., & Rourke, S. J. (2008, June). Short-term load forecasting: Similar day based wavelet neural networks. In 2008 7th World Congress on Intelligent Control and Automation (pp. 3353-3358). IEEE.
Li, S., Wang, P., & Goel, L. (2015). A novel wavelet-based ensemble method for short-term load forecasting with hybrid neural networks and feature selection. IEEE Transactions on power systems, 31(3), 1788-1798
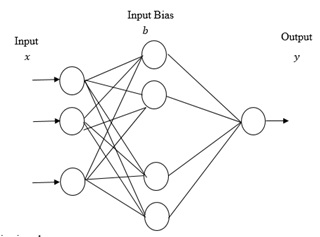
Published
How to Cite
Issue
Section
License
Copyright (c) 2022 50Sea

This work is licensed under a Creative Commons Attribution 4.0 International License.