Realistic Face Super-Resolution via Generative Adversarial Networks: Enhancing Facial Recognition in Real-world Scenarios
Keywords:
Super-Resolution, Generative Adversarial Networks, Face Recognition, Surveillance datasets, Image Quality EnhancementAbstract
he accuracy of real-world facial recognition operations faces challenges because of the difficulties connected to Low-Resolution image quality. This indicates that super-resolution methods play a vital role in improving recognition outcomes. Currently, available SR techniques do not achieve generalization due to their dependence on synthetic LR data that uses basic down sampling processes. The proposed GAN-based approach establishes a solution to this challenge through its simulation of actual degradation algorithms which combine Gaussian blur with noise addition and color modification and JPEG compression. Random application of augmentation parameters allows the GAN model to acquire knowledge about diverse and realistic low-resolution data distribution patterns during training. A unique unaligned face image pair dataset was made specifically for research using Zoom-In and Zoom-Out methods to capture high-resolution and low-resolution images from the same individuals. The dataset presents authentic real-life scenarios better than conventional paired collection methods. Based on experimental results our method produces substantial gains in performance compared to other super-resolution methods across both self-created face data as well as established surveillance data. The proposed model achieves higher visual quality standards while improving facial recognition accuracy under different operational situations. In conclusion, this study implements an effective SR solution for facial recognition which tackles problems with standard training datasets while creating authentic face image data. The proposed method shows promise for enhancing SR applications which need high-quality facial recognition capability in surveillance systems and other security-based operations.
References
W. S. Christian Ledig, Lucas Theis, Ferenc Huszar, Jose Caballero, Andrew Cunningham, Alejandro Acosta, Andrew Aitken, Alykhan Tejani, Johannes Totz, Zehan Wang, “Photo-Realistic Single Image Super-Resolution Using a Generative Adversarial Network,” arXiv:1609.04802, 2017, doi: https://doi.org/10.48550/arXiv.1609.04802 Focus to learn more.
M. Farooq, M. N. Dailey, A. Mahmood, J. Moonrinta, and M. Ekpanyapong, “Human face super-resolution on poor quality surveillance video footage,” Neural Comput. Appl., vol. 33, no. 20, pp. 13505–13523, Apr. 2021, doi: 10.1007/S00521-021-05973-0/METRICS.
J. Y. & G. T. Adrian Bulat, “To Learn Image Super-Resolution, Use a GAN to Learn How to Do Image Degradation First,” Comput. Vis. – ECCV 2018, pp. 187–202, 2018, doi: https://doi.org/10.1007/978-3-030-01231-1_12.
H. Z. and Y. S. X. Wang, Y. Li, “Towards Real-World Blind Face Restoration with Generative Facial Prior,” 2021 IEEE/CVF Conf. Comput. Vis. Pattern Recognit. (CVPR), Nashville, TN, USA, pp. 9164–9174, 2021, doi: 10.1109/CVPR46437.2021.00905.
J. M. T. Ioannis Gatopoulos, “Self-Supervised Variational Auto-Encoders,” Entropy, vol. 23, no. 6, p. 747, 2021, doi: https://doi.org/10.3390/e23060747.
A. M. A. Ali Heydari, “SRVAE: super resolution using variational autoencoders,” Pattern Recognit. Track., 2020, doi: https://doi.org/10.1117/12.2559808.
D. Chira, I. Haralampiev, O. Winther, A. Dittadi, and V. Liévin, “Image Super-Resolution with Deep Variational Autoencoders,” Lect. Notes Comput. Sci. (including Subser. Lect. Notes Artif. Intell. Lect. Notes Bioinformatics), vol. 13802 LNCS, pp. 395–411, 2023, doi: 10.1007/978-3-031-25063-7_24.
Y. Q. & C. C. L. Xintao Wang, Ke Yu, Shixiang Wu, Jinjin Gu, Yihao Liu, Chao Dong, “ESRGAN: Enhanced Super-Resolution Generative Adversarial Networks,” Comput. Vis. – ECCV 2018 Work., pp. 63–79, 2019, doi: https://doi.org/10.1007/978-3-030-11021-5_5.
L. Z. Tao Yang, Peiran Ren, Xuansong Xie, “GAN Prior Embedded Network for Blind Face Restoration in the Wild,” arXiv:2105.06070, 2021, doi: https://doi.org/10.48550/arXiv.2105.06070.
X. Wang, L. Xie, C. Dong, and Y. Shan, “Real-ESRGAN: Training Real-World Blind Super-Resolution with Pure Synthetic Data,” Proc. IEEE Int. Conf. Comput. Vis., vol. 2021-October, pp. 1905–1914, 2021, doi: 10.1109/ICCVW54120.2021.00217.
C. C. L. Kelvin C.K. Chan, Xiangyu Xu, Xintao Wang, Jinwei Gu, “GLEAN: Generative Latent Bank for Image Super-Resolution and Beyond,” arXiv:2207.14812, 2022, doi: https://doi.org/10.48550/arXiv.2207.14812.
K.-Y. K. W. Chaofeng Chen, Xiaoming Li, Lingbo Yang, Xianhui Lin, Lei Zhang, “Progressive Semantic-Aware Style Transformation for Blind Face Restoration,” arXiv:2009.08709, 2020, doi: https://doi.org/10.48550/arXiv.2009.08709.
Y. Gu et al., “VQFR: Blind Face Restoration with Vector-Quantized Dictionary and Parallel Decoder,” Lect. Notes Comput. Sci. (including Subser. Lect. Notes Artif. Intell. Lect. Notes Bioinformatics), vol. 13678 LNCS, pp. 126–143, 2022, doi: 10.1007/978-3-031-19797-0_8.
H. L. Zhendong Wang, Xiaodong Cun, Jianmin Bao, Wengang Zhou, Jianzhuang Liu, “Uformer: A General U-Shaped Transformer for Image Restoration,” arXiv:2106.03106, 2021, doi: https://doi.org/10.48550/arXiv.2106.03106.
R. T. Jingyun Liang, Jiezhang Cao, Guolei Sun, Kai Zhang, Luc Van Gool, “SwinIR: Image Restoration Using Swin Transformer,” arXiv:2108.10257, 2021, doi: https://doi.org/10.48550/arXiv.2108.10257.
L. V. G. Jiezhang Cao, Jingyun Liang, Kai Zhang, Yawei Li, Yulun Zhang, Wenguan Wang, “Reference-based Image Super-Resolution with Deformable Attention Transformer,” arXiv:2207.11938, 2022, doi: https://doi.org/10.48550/arXiv.2207.11938.
M. N. Chitwan Saharia, Jonathan Ho, William Chan, Tim Salimans, David J. Fleet, “Image Super-Resolution via Iterative Refinement,” arXiv:2104.07636, 2021, doi: https://doi.org/10.48550/arXiv.2104.07636.
S. Gao et al, “Implicit Diffusion Models for Continuous Super-Resolution,” 2023 IEEE/CVF Conf. Comput. Vis. Pattern Recognit. (CVPR), Vancouver, BC, Canada, pp. 10021–10030, 2023, doi: 10.1109/CVPR52729.2023.00966.
Y. Zhu et al, “Denoising Diffusion Models for Plug-and-Play Image Restoration,” 2023 IEEE/CVF Conf. Comput. Vis. Pattern Recognit. Work. (CVPRW), Vancouver, BC, Canada, pp. 219–1229, 2023, doi: 10.1109/CVPRW59228.2023.00129.
Y. C. Haoying Li, Yifan Yang, Meng Chang, Shiqi Chen, Huajun Feng, Zhihai Xu, Qi Li, “SRDiff: Single image super-resolution with diffusion probabilistic models,” Neurocomputing, vol. 479, pp. 47–59, 2022, doi: https://doi.org/10.1016/j.neucom.2022.01.029.
X. Zhang, Q. Chen, R. Ng, and V. Koltun, “Zoom to learn, learn to zoom,” Proc. IEEE Comput. Soc. Conf. Comput. Vis. Pattern Recognit., vol. 2019-June, pp. 3757–3765, Jun. 2019, doi: 10.1109/CVPR.2019.00388.
L. Zheng, J. Zhu, J. Shi, and S. Weng, “Efficient mixed transformer for single image super-resolution,” Eng. Appl. Artif. Intell., vol. 133, p. 108035, 2024, doi: https://doi.org/10.1016/j.engappai.2024.108035.
C. C. L. Zongsheng Yue, Jianyi Wang, “ResShift: Efficient Diffusion Model for Image Super-resolution by Residual Shifting,” arXiv:2307.12348, 2023, doi: https://doi.org/10.48550/arXiv.2307.12348.
K. Zhang, L. Van Gool, and R. Timofte, “Deep unfolding network for image super-resolution,” Proc. IEEE Comput. Soc. Conf. Comput. Vis. Pattern Recognit., pp. 3214–3223, 2020, doi: 10.1109/CVPR42600.2020.00328.
J. Y. Zhu, T. Park, P. Isola, and A. A. Efros, “Unpaired Image-to-Image Translation Using Cycle-Consistent Adversarial Networks,” Proc. IEEE Int. Conf. Comput. Vis., vol. 2017-October, pp. 2242–2251, Dec. 2017, doi: 10.1109/ICCV.2017.244.
S. H. Martin Heusel, Hubert Ramsauer, Thomas Unterthiner, Bernhard Nessler, “GANs Trained by a Two Time-Scale Update Rule Converge to a Local Nash Equilibrium,” arXiv:1706.08500, 2018, doi: https://doi.org/10.48550/arXiv.1706.08500.
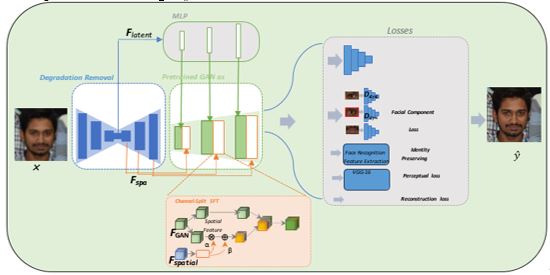
Downloads
Published
How to Cite
Issue
Section
License
Copyright (c) 2025 50sea

This work is licensed under a Creative Commons Attribution 4.0 International License.