Disease Detection Using Wrist Pulse Analysis
Keywords:
Wrist Pulses, Wrist Pulse Analysis, Disease Detection, Pulse Signal Processing and analysis, Machine LearningAbstract
Early detection of diseases is crucial for effective treatment and management. Traditionally, disease detection involves invasive and costly medical procedures. However, recent advancements in non-invasive methods have proven highly successful in identifying various illnesses. The wrist pulse has long been an important tool for detecting diseases, with traditional Chinese medicine making extensive use of this method. It shows great promise in diagnosing a wide range of conditions. This study provides a detailed analysis of research on wrist pulse analysis and its applications in disease detection. It examines the physiological basis of wrist pulse analysis, focusing on the relationship between underlying medical conditions and the characteristics of wrist pulses. Additionally, the study explores how wearable pulse detectors and machine learning algorithms can improve the accuracy and effectiveness of wrist pulse analysis. In this research, we use a dataset of 300 samples from various diseases, analyzing it with MATLAB and applying ensemble learning algorithms. We have achieved accuracies above 80% for nearly all algorithms, and accuracy can be further improved by expanding the dataset with more samples and extracting additional features.
References
A. K. Zhichao Zhang, Yuan Zhang, Lina Yao, Houbing Song, “A sensor-based wrist pulse signal processing and lung cancer recognition,” J. Biomed. Inform., vol. 79, pp. 107–116, 2018, doi: https://doi.org/10.1016/j.jbi.2018.01.009.
Y. W. Shihang Wang, Zhinan Zhang, Zhijian Chen, Deqing Mei, “Development of Pressure Sensor Based Wearable Pulse Detection Device for Radial Pulse Monitoring,” Micromachines, vol. 13, no. 10, p. 1699, 2022, doi: https://doi.org/10.3390/mi13101699.
V. S. Suguna GC, “A review of wrist pulse analysis,” Biomed. Res., vol. 30, no. 4, 2019, doi: 10.35841/biomedicalresearch.30-19-174.
H. Pogadadanda, U. Shwetha Shankar, and K. R. Jansi, “Disease Diagnosis Using Ayurvedic Pulse and Treatment Recommendation Engine,” 2021 7th Int. Conf. Adv. Comput. Commun. Syst. ICACCS 2021, pp. 1254–1258, Mar. 2021, doi: 10.1109/ICACCS51430.2021.9441843.
C. A. & A. T. Veronica Zelli, Andrea Manno, Chiara Compagnoni, Rasheed Oyewole Ibraheem, Francesca Zazzeroni, Edoardo Alesse, Fabrizio Rossi, “Classification of tumor types using XGBoost machine learning model: a vector space transformation of genomic alterations,” J. Transl. Med., vol. 21, p. 836, 2023, doi: https://doi.org/10.1186/s12967-023-04720-4.
M. K. Sharoni Narang, Saumya P S K, Omkar Batwal, “Ayurveda based Disease Diagnosis using Machine Learning,” Int. Res. J. Eng. Technol., vol. 5, no. 3, pp. 3704–3707, 2018, [Online]. Available: https://www.irjet.net/archives/V5/i3/IRJET-V5I3868.pdf
F. W. & L. S. Jie Wang, Yirun Zhu, Zhiyong Wu, Yunlin Zhang, Jian Lin, Tao Chen, Huicong Liu, “Wearable multichannel pulse condition monitoring system based on flexible pressure sensor arrays,” Microsystems Nanoeng., vol. 8, no. 16, 2022, doi: https://doi.org/10.1038/s41378-022-00349-3.
W. Zuo, P. Wang, and D. Zhang, “Comparison of three different types of wrist pulse signals by their physical meanings and diagnosis performance,” IEEE J. Biomed. Heal. Informatics, vol. 20, no. 1, pp. 119–127, 2016, doi: 10.1109/JBHI.2014.2369821.
A. L. V. Bhaskar Thakker, “Support Vector Machine for Abnormal Pulse Classification,” Int. J. Comput. Appl., vol. 22, no. 7, 2011, doi: 10.5120/2597-3610.
N. N. Khaire and Y. V. Joshi, “Diagnosis of Disease Using Wrist Pulse Signal for classification of pre-meal and post-meal samples,” 2015 Int. Conf. Ind. Instrum. Control. ICIC 2015, pp. 866–869, Jul. 2015, doi: 10.1109/IIC.2015.7150864.
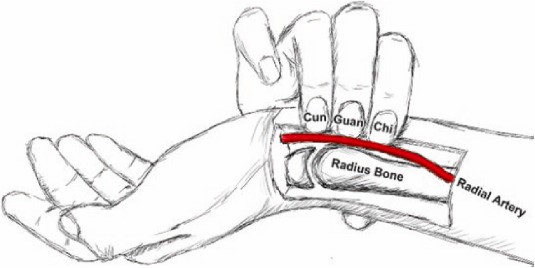
Downloads
Published
How to Cite
Issue
Section
License
Copyright (c) 2025 50sea

This work is licensed under a Creative Commons Attribution 4.0 International License.