Report Generation of Lungs Diseases From Chest X-ray using NLP
Keywords:
Attention, chest X-rays, classification, convolutional neural network, deep learning, natural language processing, pulmonary diseases, recurrent neural network, report generation.Abstract
Pulmonary diseases are very severe health complications in the world that impose a massive worldwide health burden. These diseases comprise of pneumonia, asthma, tuberculosis, Covid-19, cancer, etc. The evidences show that around 65 million people undergo the chronic obstructive pulmonary disease and nearly 3 million people pass away from it each year that make it the third prominent reason of death worldwide. To decrease the burden of lungs diseases timely diagnosis is very essential. Computer-aided diagnostic, are systems that support doctors in the analysis of medical images. This study showcases that Report Generation System has automated the Chest X-Ray interpretation procedure and lessen human effort, consequently helped the people for timely diagnoses of chronic lungs diseases to decrease the death rate. This system provides great relief for people in rural areas where the doctor-to-patient ratio is only 1 doctor per 1300 people. As a result, after utilizing this application, the affected individual can seek further therapy for the ailment they have been diagnosed with. The proposed system is supposed to be used in the distinct architecture of deep learning (Deep Convolution Neural Network), this is fine tuned to CNN-RNN trainable end-to-end architecture. By using the patient-wise official split of the OpenI dataset we have trained a CNN-RNN model with attention. Our model achieved an accuracy of 94%, which is the highest performance.
References
H. Wang, H. Jia, L. Lu, and Y. Xia, “Thorax-Net: An Attention Regularized Deep Neural Network for Classification of Thoracic Diseases on Chest Radiography,” IEEE J. Biomed. Heal. informatics, vol. 24, no. 2, pp. 475–485, Feb. 2020, doi: 10.1109/JBHI.2019.2928369.
Z. Ul Abideen et al., “Uncertainty Assisted Robust Tuberculosis Identification With Bayesian Convolutional Neural Networks,” IEEE access Pract. Innov. open Solut., vol. 8, pp. 22812–22825, 2020, doi: 10.1109/ACCESS.2020.2970023.
Z. Huo et al., “Quality assurance and training procedures for computer-aided detection and diagnosis systems in clinical use,” Med. Phys., vol. 40, no. 7, 2013, doi: 10.1118/1.4807642.
X. Wang, Y. Peng, L. Lu, Z. Lu, and R. M. Summers, “TieNet: Text-Image Embedding Network for Common Thorax Disease Classification and Reporting in Chest X-rays.”
J. Shi, C. Liu, C. T. Ishi, and H. Ishiguro, “3D skeletal movement-enhanced emotion recognition networks,” APSIPA Trans. Signal Inf. Process., vol. 10, pp. 1060–1066, Dec. 2021, doi: 10.1017/ATSIP.2021.11.
M. Almuhayar, H. H. S. Lu, and N. Iriawan, “Classification of Abnormality in Chest X-Ray Images by Transfer Learning of CheXNet,” ICICOS 2019 - 3rd Int. Conf. Informatics Comput. Sci. Accel. Informatics Comput. Res. Smarter Soc. Era Ind. 4.0, Proc., p. 8982455, Oct. 2019, doi: 10.1109/ICICOS48119.2019.8982455.
“Fundamentals of Diagnostic Radiology | William E. Brant, Clyde A. Helms | download.” https://en.1lib.ae/book/2738744/017b45 (accessed Feb. 26, 2022).
E. A. Mendonça, J. Haas, L. Shagina, E. Larson, and C. Friedman, “Extracting information on pneumonia in infants using natural language processing of radiology reports,” J. Biomed. Inform., vol. 38, no. 4, pp. 314–321, Aug. 2005, doi: 10.1016/J.JBI.2005.02.003.
M. Horry et al., “Deep mining generation of lung cancer malignancy models from chest x-ray images,” Sensors, vol. 21, no. 19, Oct. 2021, doi: 10.3390/S21196655.
J. Lovelace and B. Mortazavi, “Learning to Generate Clinically Coherent Chest X-Ray Reports,” Find. Assoc. Comput. Linguist. Find. ACL EMNLP 2020, pp. 1235–1243, 2020, doi: 10.18653/V1/2020.FINDINGS-EMNLP.110.
D. Demner-Fushman et al., “Preparing a collection of radiology examinations for distribution and retrieval.,” J. Am. Med. Inform. Assoc., vol. 23, no. 2, pp. 304–310, Jul. 2015, doi: 10.1093/JAMIA/OCV080.
Y. Jeon and J. Kim, “Active Convolution: Learning the Shape of Convolution for Image Classification,” Accessed: Feb. 26, 2022. [Online]. Available: https://github.com/.
V. Nair and G. E. Hinton, “Rectified Linear Units Improve Restricted Boltzmann Machines.”
S. H. S. Basha, S. R. Dubey, V. Pulabaigari, and S. Mukherjee, “Impact of fully connected layers on performance of convolutional neural networks for image classification,” Neurocomputing, vol. 378, pp. 112–119, Feb. 2020, doi: 10.1016/J.NEUCOM.2019.10.008.
N. M. Nasrabadi, “Book Review: Pattern Recognition and Machine Learning,” https://doi.org/10.1117/1.2819119, vol. 16, no. 4, p. 049901, Oct. 2007, doi: 10.1117/1.2819119.
K. Greff, R. K. Srivastava, J. Koutník, B. R. Steunebrink, and J. Schmidhuber, “TRANSACTIONS ON NEURAL NETWORKS AND LEARNING SYSTEMS 1 LSTM: A Search Space Odyssey,” doi: 10.1109/TNNLS.2016.2582924.
S. Wu, S. Zhao, Q. Zhang, L. Chen, and C. Wu, “Steel surface defect classification based on small sample learning,” Appl. Sci., vol. 11, no. 23, 2021, doi: 10.3390/app112311459.
D. Bahdanau, K. H. Cho, and Y. Bengio, “Neural Machine Translation by Jointly Learning to Align and Translate,” 3rd Int. Conf. Learn. Represent. ICLR 2015 - Conf. Track Proc., Sep. 2014, Accessed: Feb. 26, 2022. [Online]. Available: https://arxiv.org/abs/1409.0473v7.
M. T. Luong, H. Pham, and C. D. Manning, “Effective Approaches to Attention-based Neural Machine Translation,” Conf. Proc. - EMNLP 2015 Conf. Empir. Methods Nat. Lang. Process., pp. 1412–1421, Aug. 2015, doi: 10.18653/v1/d15-1166.
J. Pennington, R. Socher, and C. D. Manning, “GloVe: Global Vectors for Word Representation,” EMNLP 2014 - 2014 Conf. Empir. Methods Nat. Lang. Process. Proc. Conf., pp. 1532–1543, 2014, doi: 10.3115/V1/D14-1162.
A. Gulli, A. Kapoor, S. Pal, O’Reilly for Higher Education (Firm), and an O. M. C. Safari, “Deep Learning with TensorFlow 2 and Keras - Second Edition,” p. 646.
K. Papineni, S. Roukos, T. Ward, and W.-J. Zhu, “Bleu: a Method for Automatic Evaluation of Machine Translation,” Proc. 40th Annu. Meet. Assoc. Comput. Linguist., pp. 311–318, 2002, doi: 10.3115/1073083.1073135.
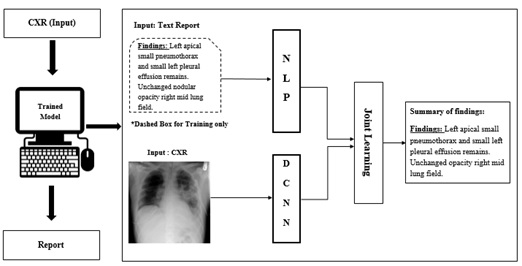
Published
How to Cite
Issue
Section
License
Copyright (c) 2022 50Sea

This work is licensed under a Creative Commons Attribution 4.0 International License.