Integration of Probability Based Ridge Variation Information with Local Ridge Orientation for Fingerprint Liveness Detection
Keywords:
PWM, Ridge Orientation, Feature integration, Ridge variation, ridge-valley pattern, Higher-order derivative.Abstract
Fingerprints are commonly used in biometric systems. However, the authentication of these systems became an open challenge because fingerprints can easily be fabricated. In this paper, a hybrid feature extraction approach named Integration of Probability Weighted Spatial Gradient with Ridge Orientation (IPWSGRo) has been proposed for fingerprint liveness detection. IPWSGRo integrates intensity variation and local ridge orientation information. Intensity variation is computed by using probability-weighted moments (PWM) and second order directional derivative filter. Moreover, the ridge orientation is estimated using rotation invariant Local Phase Quantization (LPQri) by retaining only the significant frequency components. These two feature vectors are quantized into predefined intervals to plot a 2-D histogram. The support vector machine classifier (SVM) is then used to determine the validity of fingerprints as either live or spoof. Results are obtained by applying the proposed technique on three standard databases of LivDet competition 2011, 2013, and 2015. Experimental results indicate that the proposed method is able to reduce the average classification error rates (ACER) to 5.7, 2.1, and 5.17% on LivDet2011, 2013, and 2015, respectively.
References
Z. Xia, C. Yuan, R. Lv, X. Sun, N. N. Xiong, and Y. Q. Shi, “A novel weber local binary descriptor for fingerprint liveness detection,” IEEE Trans. Syst. Man, Cybern. Syst., vol. 50, no. 4, 2020, doi: 10.1109/TSMC.2018.2874281.
Z. Xia, C. Yuan, X. Sun, D. Sun, and R. Lv, “Combining wavelet transform and LBP related features for fingerprint liveness detection,” IAENG Int. J. Comput. Sci., vol. 43, pp. 290–298, 2016.
Z. Xia, R. Lv, Y. Zhu, P. Ji, H. Sun, and Y. Q. Shi, “Fingerprint liveness detection using gradient-based texture features,” Signal, Image Video Process., vol. 11, no. 2, 2017, doi: 10.1007/s11760-016-0936-z.
A. Abhyankar and S. Schuckers, “Fingerprint liveness detection using local ridge frequencies and multiresolution texture analysis techniques,” 2006, doi: 10.1109/ICIP.2006.313158.
E. Liu and K. Cao, “Minutiae Extraction from Level 1 Features of Fingerprint,” IEEE Trans. Inf. Forensics Secur., vol. 11, no. 9, 2016, doi: 10.1109/TIFS.2016.2541345.
P. Johnson and S. Schuckers, “Fingerprint pore characteristics for liveness detection,” in Lecture Notes in Informatics (LNI), Proceedings - Series of the Gesellschaft fur Informatik (GI), 2014, vol. P-230.
R. Donida Labati, A. Genovese, E. Muñoz, V. Piuri, and F. Scotti, “A novel pore extraction method for heterogeneous fingerprint images using Convolutional Neural Networks,” Pattern Recognit. Lett., vol. 113, 2018, doi: 10.1016/j.patrec.2017.04.001.
T. Ojala, M. Pietikäinen, and T. Mäenpää, “Multiresolution gray-scale and rotation invariant texture classification with local binary patterns,” IEEE Trans. Pattern Anal. Mach. Intell., vol. 24, no. 7, 2002, doi: 10.1109/TPAMI.2002.1017623.
L. Ghiani, G. L. Marcialis, and F. Roli, “Fingerprint liveness detection by local phase quantization,” 2012.
J. Chen et al., “WLD: A robust local image descriptor,” IEEE Trans. Pattern Anal. Mach. Intell., vol. 32, no. 9, 2010, doi: 10.1109/TPAMI.2009.155.
D. Gragnaniello, G. Poggi, C. Sansone, and L. Verdoliva, “An Investigation of Local Descriptors for Biometric Spoofing Detection,” IEEE Trans. Inf. Forensics Secur., vol. 10, no. 4, 2015, doi: 10.1109/TIFS.2015.2404294.
L. Ghiani, A. Hadid, G. L. Marcialis, and F. Roli, “Fingerprint Liveness Detection using Binarized Statistical Image Features,” 2013, doi: 10.1109/BTAS.2013.6712708.
W. Kim, “Fingerprint Liveness Detection Using Local Coherence Patterns,” IEEE Signal Process. Lett., vol. 24, no. 1, 2017, doi: 10.1109/LSP.2016.2636158.
M. Verma and B. Raman, “Local tri-directional patterns: A new texture feature descriptor for image retrieval,” Digit. Signal Process. A Rev. J., vol. 51, 2016, doi: 10.1016/j.dsp.2016.02.002.
B. Zhang, Y. Gao, S. Zhao, and J. Liu, “Local derivative pattern versus local binary pattern: Face recognition with high-order local pattern descriptor,” IEEE Trans. Image Process., vol. 19, no. 2, 2010, doi: 10.1109/TIP.2009.2035882.
D. Gragnaniello, G. Poggi, C. Sansone, and L. Verdoliva, “Local contrast phase descriptor for fingerprint liveness detection,” Pattern Recognit., vol. 48, no. 4, 2015, doi: 10.1016/j.patcog.2014.05.021.
R. Mehboob, H. Dawood, H. Dawood, M. U. Ilyas, P. Guo, and A. Banjar, “Live fingerprint detection using magnitude of perceived spatial stimuli and local phase information,” J. Electron. Imaging, vol. 27, no. 05, 2018, doi: 10.1117/1.jei.27.5.053038.
A. Alshdadi, R. Mehboob, H. Dawood, M. O. Alassafi, R. Alghamdi, and H. Dawood, “Exploiting Level 1 and Level 3 features of fingerprints for liveness detection,” Biomed. Signal Process. Control, vol. 61, 2020, doi: 10.1016/j.bspc.2020.102039.
M. Sajjad et al., “CNN-based anti-spoofing two-tier multi-factor authentication system,” Pattern Recognit. Lett., vol. 126, 2019, doi: 10.1016/j.patrec.2018.02.015.
D. M. Uliyan, S. Sadeghi, and H. A. Jalab, “Anti-spoofing method for fingerprint recognition using patch based deep learning machine,” Eng. Sci. Technol. an Int. J., vol. 23, no. 2, 2020, doi: 10.1016/j.jestch.2019.06.005.
W. Jian, Y. Zhou, and H. Liu, “Densely Connected Convolutional Network Optimized by Genetic Algorithm for Fingerprint Liveness Detection,” IEEE Access, vol. 9, 2021, doi: 10.1109/ACCESS.2020.3047723.
H. Dawood et al., “Probability weighted moments regularization based blind image De-blurring,” Multimed. Tools Appl., vol. 79, no. 7–8, 2020, doi: 10.1007/s11042-019-7520-9.
R. M. Yousaf, H. A. Habib, Z. Mehmood, A. Banjar, R. Alharbey, and O. Aboulola, “Single image dehazing and edge preservation based on the dark channel probability-weighted moments,” Math. Probl. Eng., vol. 2019, 2019, doi: 10.1155/2019/9721503.
J. J. Mathew and A. P. James, “Spatial stimuli gradient sketch model,” IEEE Signal Process. Lett., vol. 22, no. 9, 2015, doi: 10.1109/LSP.2015.2404827.
D. Yambay, L. Ghiani, P. Denti, G. L. Marcialis, F. Roli, and S. Schuckers, “LivDet 2011 - Fingerprint liveness detection competition 2011,” 2012, doi: 10.1109/ICB.2012.6199810.
L. Ghiani et al., “LivDet 2013 fingerprint liveness detection competition 2013,” 2013, doi: 10.1109/ICB.2013.6613027.
L. Ghiani, D. A. Yambay, V. Mura, G. L. Marcialis, F. Roli, and S. A. Schuckers, “Review of the Fingerprint Liveness Detection (LivDet) competition series: 2009 to 2015,” Image Vis. Comput., vol. 58, 2017, doi: 10.1016/j.imavis.2016.07.002.
C. Yuan, Z. Xia, L. Jiang, Y. Cao, Q. M. J. Wu, and X. Sun, “Fingerprint Liveness Detection Using an Improved CNN With Image Scale Equalization,” IEEE Access, vol. 7, 2019, doi: 10.1109/ACCESS.2019.2901235.
S. Kim, B. Park, B. S. Song, and S. Yang, “Deep belief network based statistical feature learning for fingerprint liveness detection,” Pattern Recognit. Lett., vol. 77, 2016, doi: 10.1016/j.patrec.2016.03.015.
D. Gragnaniello, G. Poggi, C. Sansone, and L. Verdoliva, “Fingerprint liveness detection based on Weber Local image Descriptor,” 2013, doi: 10.1109/BIOMS.2013.6656148.
C. Yuan, Z. Xia, X. Sun, and Q. M. J. Wu, “Deep Residual Network with Adaptive Learning Framework for Fingerprint Liveness Detection,” IEEE Trans. Cogn. Dev. Syst., vol. 12, no. 3, 2020, doi: 10.1109/TCDS.2019.2920364.
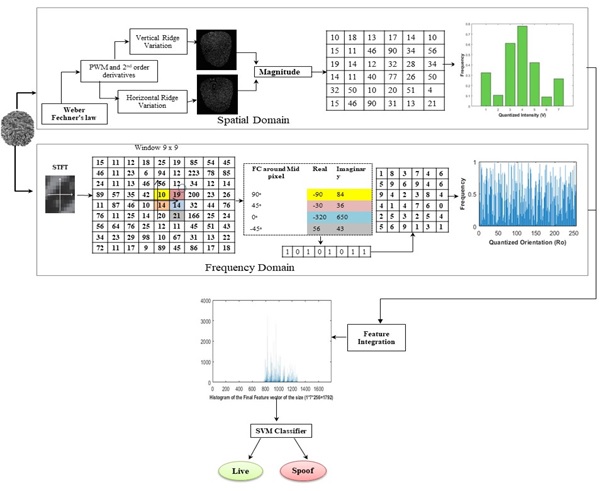
Published
How to Cite
Issue
Section
License
Copyright (c) 2022 50Sea

This work is licensed under a Creative Commons Attribution 4.0 International License.