Restrictions, Challenges and Opportunities for AI and ML
Keywords:
AI, ML, Restriction, OpportunitiesAbstract
Artificial intelligence (AI) refers to a collection of techniques that are being developed to address a wide variety of practical problems. Machine learning (ML) is the backbone of artificial intelligence (AI), comprising a suite of algorithms and techniques designed to solve the issues of categorization, clustering, and prediction. There are bright prospects for putting AI and ML to use in the real world. As a result, there is a lot of study being done in this field. However, mainstream adoption of AI in industry and its widespread use in society are still in their infancy. For understanding the obstacles involved with mainstream AI implementations, both the AI (internal problems) and societal (external problems) viewpoints are required. With this in mind, we can determine what has to happen first to get AI technology into the hands of industry and the public. This article identifies and discusses some of the obstacles to using artificial intelligence in resource-based economies and societies. Publications in the field form the basis for the systematic application of AI&ML technology. This methodical approach makes it possible to define institutional, human resource, societal, and technological constraints. This paper provides a roadmap for future research in artificial intelligence and machine learning that will help us overcome current obstacles and broaden the range of these technologies' potential uses.
References
L. Mou, P. Ghamisi, and X. X. Zhu, “Deep recurrent neural networks for hyperspectral image classification,” IEEE Trans. Geosci. Remote Sens., vol. 55, no. 7, pp. 3639–3655, 2017, doi: 10.1109/TGRS.2016.2636241.
T. J, de Silva V, and L. J, “A global geometric framework for nonlinear dimensionality reduction,” Science (80-. )., vol. 290, no. December, pp. 2319–2323, 2000.
A. Sanenga, G. A. Mapunda, T. M. L. Jacob, L. Marata, B. Basutli, and J. M. Chuma, “An overview of key technologies in physical layer security,” Entropy, vol. 22, no. 11, pp. 1–34, 2020, doi: 10.3390/e22111261.
W. N. Hussein, L. M. Kamarudin, H. N. Hussain, A. Zakaria, R. Badlishah Ahmed, and N. A. H. Zahri, “The Prospect of Internet of Things and Big Data Analytics in Transportation System,” J. Phys. Conf. Ser., vol. 1018, no. 1, 2018, doi: 10.1088/1742-6596/1018/1/012013.
D. Mhlanga, “Industry 4.0 in finance: the impact of artificial intelligence (ai) on digital financial inclusion,” Int. J. Financ. Stud., vol. 8, no. 3, pp. 1–14, 2020, doi: 10.3390/ijfs8030045.
R. S. Peres, X. Jia, J. Lee, K. Sun, A. W. Colombo, and J. Barata, “Industrial Artificial Intelligence in Industry 4.0 -Systematic Review, Challenges and Outlook,” IEEE Access, pp. 220121–220139, 2020, doi: 10.1109/ACCESS.2020.3042874.
M. H. Jarrahi, “Artificial intelligence and the future of work: Human-AI symbiosis in organizational decision making,” Bus. Horiz., vol. 61, no. 4, pp. 577–586, 2018, doi: 10.1016/j.bushor.2018.03.007.
W. Wang and K. Siau, “Artificial intelligence, machine learning, automation, robotics, future of work and future of humanity: A review and research agenda,” J. Database Manag., vol. 30, no. 1, pp. 61–79, 2019, doi: 10.4018/JDM.2019010104.
D. Kim et al., “Review of machine learning methods in soft robotics,” PLoS One, vol. 16, no. 2 February, pp. 1–24, 2021, doi: 10.1371/journal.pone.0246102.
M. A. Kilian, M. Kattenbeck, M. Ferstl, B. Ludwig, and F. Alt, “Towards task-sensitive assistance in public spaces,” Aslib J. Inf. Manag., vol. 71, no. 3, pp. 344–367, 2019, doi: 10.1108/AJIM-07-2018-0179.
S. Khan, A. Khan, M. Maqsood, F. Aadil, and M. A. Ghazanfar, “Optimized Gabor Feature Extraction for Mass Classification Using Cuckoo Search for Big Data E-Healthcare,” J. Grid Comput., vol. 17, no. 2, pp. 239–254, 2019, doi: 10.1007/s10723-018-9459-x.
A. Mosavi and A. Varkonyi, “Learning in Robotics,” Int. J. Comput. Appl., vol. 157, no. 1, pp. 8–11, 2017, doi: 10.5120/ijca2017911661.
Y. Lecun and K. Kavukcuoglu, “IEEE Xplore - Convolutional networks and applications in vision”, [Online]. Available: http://ieeexplore.ieee.org/xpls/abs_all.jsp?arnumber=5537907
Z. Liu et al., “Swin Transformer V2: Scaling Up Capacity and Resolution,” Proc. IEEE Comput. Soc. Conf. Comput. Vis. Pattern Recognit., vol. 2022-June, pp. 11999–12009, 2022, doi: 10.1109/CVPR52688.2022.01170.
Yoav Goldberg, “A Primer on Neural Network Models for Natural Language Processing,” J. Artif. Intell. Res., vol. 57, pp. 345–420, 2016, [Online]. Available: http://www.jair.org/papers/paper4992.html
J. P. Usuga Cadavid, S. Lamouri, B. Grabot, R. Pellerin, and A. Fortin, “Machine learning applied in production planning and control: a state-of-the-art in the era of industry 4.0,” J. Intell. Manuf., vol. 31, no. 6, pp. 1531–1558, 2020, doi: 10.1007/s10845-019-01531-7.
MacQueen, James and others, “Some methods for classification and analysis of multivariate observations,” Proc. fifth Berkeley Symp. Math. Stat. Probab., vol. 1, no. 14, pp. 281–297, 1967, [Online]. Available: http://books.google.de/books?hl=de&lr=&id=IC4Ku_7dBFUC&oi=fnd&pg=PA281&dq=MacQueen+some+methods+for+classification&ots=nNTcK1IdoQ&sig=fHzdVcbvmYJ-lTNHu1HncmOFOkM#v=onepage&q=MacQueen some methods for classification&f=false
Y. I. Kuchin, R. I. Mukhamediev, and K. O. Yakunin, “One method of generating synthetic data to assess the upper limit of machine learning algorithms performance,” Cogent Eng., vol. 7, no. 1, 2020, doi: 10.1080/23311916.2020.1718821.
Y. Motai, N. A. Siddique, and H. Yoshida, “Heterogeneous data analysis: Online learning for medical-image-based diagnosis,” Pattern Recognit., vol. 63, no. September 2016, pp. 612–624, 2017, doi: 10.1016/j.patcog.2016.09.035.
C. A. Hidalgo and R. Hausmann, “The building blocks of economic complexity,” Proc. Natl. Acad. Sci. U. S. A., vol. 106, no. 26, pp. 10570–10575, 2009, doi: 10.1073/pnas.0900943106.
G. Plastiras, C. Kyrkou, and T. Theocharides, “Efficient convnet-based object detection for unmanned aerial vehicles by selective tile processing,” ACM Int. Conf. Proceeding Ser., 2018, doi: 10.1145/3243394.3243692.
M. Macas and C. Wu, “Review: Deep Learning Methods for Cybersecurity and Intrusion Detection Systems,” Proc. - 2020 IEEE Latin-American Conf. Commun. LATINCOM 2020, pp. 1–6, 2020, doi: 10.1109/LATINCOM50620.2020.9282324.
N. Artrith et al., “Best practices in machine learning for chemistry To cite this version : HAL Id : hal-03243917 Best practices in machine learning for chemistry,” 2021.
G. Ros, L. Sellart, J. Materzynska, D. Vazquez, and A. M. Lopez, “The SYNTHIA Dataset: A Large Collection of Synthetic Images for Semantic Segmentation of Urban Scenes,” Proc. IEEE Comput. Soc. Conf. Comput. Vis. Pattern Recognit., vol. 2016-Decem, no. 600388, pp. 3234–3243, 2016, doi: 10.1109/CVPR.2016.352.
G. Nguyen et al., “Machine Learning and Deep Learning frameworks and libraries for large-scale data mining: a survey,” Artif. Intell. Rev., vol. 52, no. 1, pp. 77–124, 2019, doi: 10.1007/s10462-018-09679-z.
H. F. Yu, F. L. Huang, and C. J. Lin, “Dual coordinate descent methods for logistic regression and maximum entropy models,” Mach. Learn., vol. 85, no. 1–2, pp. 41–75, 2011, doi: 10.1007/s10994-010-5221-8.
K. G. Liakos, P. Busato, D. Moshou, S. Pearson, and D. Bochtis, “Machine learning in agriculture: A review,” Sensors (Switzerland), vol. 18, no. 8, pp. 1–29, 2018, doi: 10.3390/s18082674.
G. Van den Broeck, A. Lykov, M. Schleich, and D. Suciu, “On the Tractability of SHAP Explanations,” J. Artif. Intell. Res., vol. 74, pp. 851–886, 2022, doi: 10.1613/jair.1.13283.
D. Widdows, K. Kitto, and T. Cohen, “Quantum Mathematics in Artificial Intelligence,” J. Artif. Intell. Res., vol. 72, pp. 1307–1341, 2021, doi: 10.1613/JAIR.1.12702.
A. B. Nassif, I. Shahin, I. Attili, M. Azzeh, and K. Shaalan, “Speech Recognition Using Deep Neural Networks: A Systematic Review,” IEEE Access, vol. 7, pp. 19143–19165, 2019, doi: 10.1109/ACCESS.2019.2896880.
M. Duguleană, V. A. Briciu, I. A. Duduman, and O. M. Machidon, “A virtual assistant for natural interactions in museums,” Sustain., vol. 12, no. 17, pp. 1–17, 2020, doi: 10.3390/SU12176958.
R. I. Mukhamediev et al., “Classification of negative information on socially significant topics in mass media,” Symmetry (Basel)., vol. 12, no. 12, pp. 1–23, 2020, doi: 10.3390/sym12121945.
G. Zeng, Y. He, Z. Yu, X. Yang, R. Yang, and L. Zhang, “Preparation of novel high copper ions removal membranes by embedding organosilane-functionalized multi-walled carbon nanotube,” J. Chem. Technol. Biotechnol., vol. 91, no. 8, pp. 2322–2330, 2016, doi: 10.1002/jctb.4820.
S. Panigrahi, A. Nanda, and T. Swarnkar, “A Survey on Transfer Learning,” Smart Innov. Syst. Technol., vol. 194, pp. 781–789, 2021, doi: 10.1007/978-981-15-5971-6_83.
B. A. Erol, A. Majumdar, P. Benavidez, P. Rad, K. K. R. Choo, and M. Jamshidi, “Toward Artificial Emotional Intelligence for Cooperative Social Human-Machine Interaction,” IEEE Trans. Comput. Soc. Syst., vol. 7, no. 1, pp. 234–246, 2020, doi: 10.1109/TCSS.2019.2922593.
T. Tuncer, F. Ertam, S. Dogan, E. Aydemir, and P. Pławiak, “Ensemble residual network-based gender and activity recognition method with signals,” J. Supercomput., vol. 76, no. 3, pp. 2119–2138, 2020, doi: 10.1007/s11227-020-03205-1.
C. R. García-Alonso, L. M. Pérez-Naranjo, and J. C. Fernández-Caballero, “Multiobjective evolutionary algorithms to identify highly autocorrelated areas: The case of spatial distribution in financially compromised farms,” Ann. Oper. Res., vol. 219, no. 1, pp. 187–202, 2014, doi: 10.1007/s10479-011-0841-3.
L. Zhang, S. Wang, and B. Liu, “Deep learning for sentiment analysis: A survey,” Wiley Interdiscip. Rev. Data Min. Knowl. Discov., vol. 8, no. 4, 2018, doi: 10.1002/widm.1253.
T. Miller, “Explanation in artificial intelligence: Insights from the social sciences,” Artif. Intell., vol. 267, pp. 1–38, 2019, doi: 10.1016/j.artint.2018.07.007.
M. S. Mahdavinejad, M. Rezvan, M. Barekatain, P. Adibi, P. Barnaghi, and A. P. Sheth, “Machine learning for internet of things data analysis: a survey,” Digit. Commun. Networks, vol. 4, no. 3, pp. 161–175, 2018, doi: 10.1016/j.dcan.2017.10.002.
H. Panetto, B. Iung, D. Ivanov, G. Weichhart, and X. Wang, “Challenges for the Cyber-Physical Manufacturing Enterprises of the Future Université de Lorraine , CNRS , CRAN , France Integration in Manufacturing ( IiM ) is the first systemic paradigm to organise humans and ma- chines as a whole system , not only at t,” 2019.
M. Zitnik, F. Nguyen, B. Wang, J. Leskovec, A. Goldenberg, and M. M. Hoffman, “Machine learning for integrating data in biology and medicine: Principles, practice, and opportunities,” Inf. Fusion, vol. 50, pp. 71–91, 2019, doi: 10.1016/j.inffus.2018.09.012.
A. L. Barbieri, O. Fadare, L. Fan, H. Singh, and V. Parkash, “Challenges in communication from referring clinicians to pathologists in the electronic health record era,” J. Pathol. Inform., vol. 9, no. 1, p. 38, 2018, doi: 10.4103/jpi.jpi.
M. T. Ribeiro, S. Singh, and C. Guestrin, “‘Why Should I Trust You?’ Explaining the Predictions of Any Classifier,” NAACL-HLT 2016 - 2016 Conf. North Am. Chapter Assoc. Comput. Linguist. Hum. Lang. Technol. Proc. Demonstr. Sess., pp. 97–101, 2016, doi: 10.18653/v1/n16-3020.
Y. Wu et al., “Google’s Neural Machine Translation System: Bridging the Gap between Human and Machine Translation,” pp. 1–23, 2016, [Online]. Available: http://arxiv.org/abs/1609.08144
S. S. Mousavi, M. Schukat, and E. Howley, “Deep Reinforcement Learning: An Overview,” Lect. Notes Networks Syst., vol. 16, pp. 426–440, 2018, doi: 10.1007/978-3-319-56991-8_32.
Y. A. Joo, Y. K. Kim, T. S. Yoon, and K. A. Lee, “Microstructure and High Temperature Oxidation Property of Fe–Cr–B Based Metal/Ceramic Composite Manufactured by Powder Injection Molding Process,” Met. Mater. Int., vol. 24, no. 2, pp. 371–379, 2018, doi: 10.1007/s12540-018-0053-3.
M. Müller, V. Casser, J. Lahoud, N. Smith, and B. Ghanem, “Sim4CV: A Photo-Realistic Simulator for Computer Vision Applications,” Int. J. Comput. Vis., vol. 126, no. 9, pp. 902–919, 2018, doi: 10.1007/s11263-018-1073-7.
A. Creswell, T. White, V. Dumoulin, K. Arulkumaran, B. Sengupta, and A. A. Bharath, “Generative Adversarial Networks: An Overview,” IEEE Signal Process. Mag., vol. 35, no. 1, pp. 53–65, 2018, doi: 10.1109/MSP.2017.2765202.
V. Garcia and J. Bruna, “Few-shot learning with graph neural networks,” 6th Int. Conf. Learn. Represent. ICLR 2018 - Conf. Track Proc., pp. 1–13, 2018.
S. Lu, Y. Zhu, W. Zhang, J. Wang, and Y. Yu, “Neural Text Generation: Past, Present and Beyond,” 2018, [Online]. Available: http://arxiv.org/abs/1803.07133
S. Watanabe et al., “ESPNet: End-to-end speech processing toolkit,” Proc. Annu. Conf. Int. Speech Commun. Assoc. INTERSPEECH, vol. 2018-Septe, pp. 2207–2211, 2018, doi: 10.21437/Interspeech.2018-1456.
A.-D. Doan, A. M. Jawaid, T.-T. Do, and T.-J. Chin, “G2D: from GTA to Data,” pp. 1–9, 2018, [Online]. Available: http://arxiv.org/abs/1806.07381
K. Do, T. Tran, and S. Venkatesh, “Graph transformation policy network for chemical reaction prediction,” Proc. ACM SIGKDD Int. Conf. Knowl. Discov. Data Min., pp. 750–760, 2019, doi: 10.1145/3292500.3330958.
J. Agnese, J. Herrera, H. Tao, and X. Zhu, “A survey and taxonomy of adversarial neural networks for text-to-image synthesis,” Wiley Interdiscip. Rev. Data Min. Knowl. Discov., vol. 10, no. 4, 2020, doi: 10.1002/widm.1345.
A. Barredo Arrieta et al., “Explainable Artificial Intelligence (XAI): Concepts, taxonomies, opportunities and challenges toward responsible AI,” Inf. Fusion, vol. 58, pp. 82–115, 2020, doi: 10.1016/j.inffus.2019.12.012.
V. Kulkarni, M. Kulkarni, and A. Pant, “Quantum computing methods for supervised learning,” Quantum Mach. Intell., vol. 3, no. 2, pp. 1–22, 2021, doi: 10.1007/s42484-021-00050-0.
P. Migkotzidis and A. Liapis, “SuSketch: Surrogate Models of Gameplay as a Design Assistant,” IEEE Trans. Games, vol. 14, no. 2, pp. 273–283, 2022, doi: 10.1109/TG.2021.3068360.
L. Yuan et al., “Florence: A New Foundation Model for Computer Vision,” 2021, [Online]. Available: http://arxiv.org/abs/2111.11432
G. Atherton and L. Cross, “The Use of Analog and Digital Games for Autism Interventions,” Front. Psychol., vol. 12, p. 3049, Aug. 2021, doi: 10.3389/FPSYG.2021.669734/BIBTEX.
M. Isabirye, D. V. . Raju, M. Kitutu, V. Yemeline, J. Deckers, and J. Poesen Additional, “We are IntechOpen , the world ’ s leading publisher of Open Access books Built by scientists , for scientists TOP 1 %,” Intech, p. 13, 2012, [Online]. Available: http://dx.doi.org/10.1039/C7RA00172J%0Ahttps://www.intechopen.com/books/advanced-biometric-technologies/liveness-detection-in-biometrics%0Ahttp://dx.doi.org/10.1016/j.colsurfa.2011.12.014
A. K. Jain, M. N. Murty, and P. J. Flynn, “Data clustering: A review,” ACM Comput. Surv., vol. 31, no. 3, pp. 264–323, 1999, doi: 10.1145/331499.331504.
A. Mardani, M. Nilashi, J. Antucheviciene, M. Tavana, R. Bausys, and O. Ibrahim, “Recent fuzzy generalisations of rough sets theory: A systematic review and methodological critique of the literature,” Complexity, vol. 2017, 2017, doi: 10.1155/2017/1608147.
H. Peng et al., “Large-scale hierarchical text classification with recursively regularized deep graph-CNN,” Web Conf. 2018 - Proc. World Wide Web Conf. WWW 2018, pp. 1063–1072, 2018, doi: 10.1145/3178876.3186005.
N. Sahadat, N. Sebkhi, and M. Ghovanloo, “Simultaneous multimodal access to wheelchair and computer for people with tetraplegia,” ICMI 2018 - Proc. 2018 Int. Conf. Multimodal Interact., pp. 393–399, 2018, doi: 10.1145/3242969.3242980.
J. Lai, J. Qiu, Z. Feng, J. Chen, and H. Fan, “Prediction of Soil Deformation in Tunnelling Using Artificial Neural Networks,” Comput. Intell. Neurosci., vol. 2016, 2016, doi: 10.1155/2016/6708183.
Y. Kuchin, R. Mukhamediev, K. Yakunin, J. Grundspenkis, and A. Symagulov, “Assessing the Impact of Expert Labelling of Training Data on the Quality of Automatic Classification of Lithological Groups Using Artificial Neural Networks,” Appl. Comput. Syst., vol. 25, no. 2, pp. 145–152, 2020, doi: 10.2478/acss-2020-0016.
J. Hemminghaus and S. Kopp, “Towards Adaptive Social Behavior Generation for Assistive Robots Using Reinforcement Learning,” ACM/IEEE Int. Conf. Human-Robot Interact., vol. Part F1271, no. March, pp. 332–340, 2017, doi: 10.1145/2909824.3020217.
F. Tahiru, “AI in education: A systematic literature review,” J. Cases Inf. Technol., vol. 23, no. 1, pp. 1–20, 2021, doi: 10.4018/JCIT.2021010101.
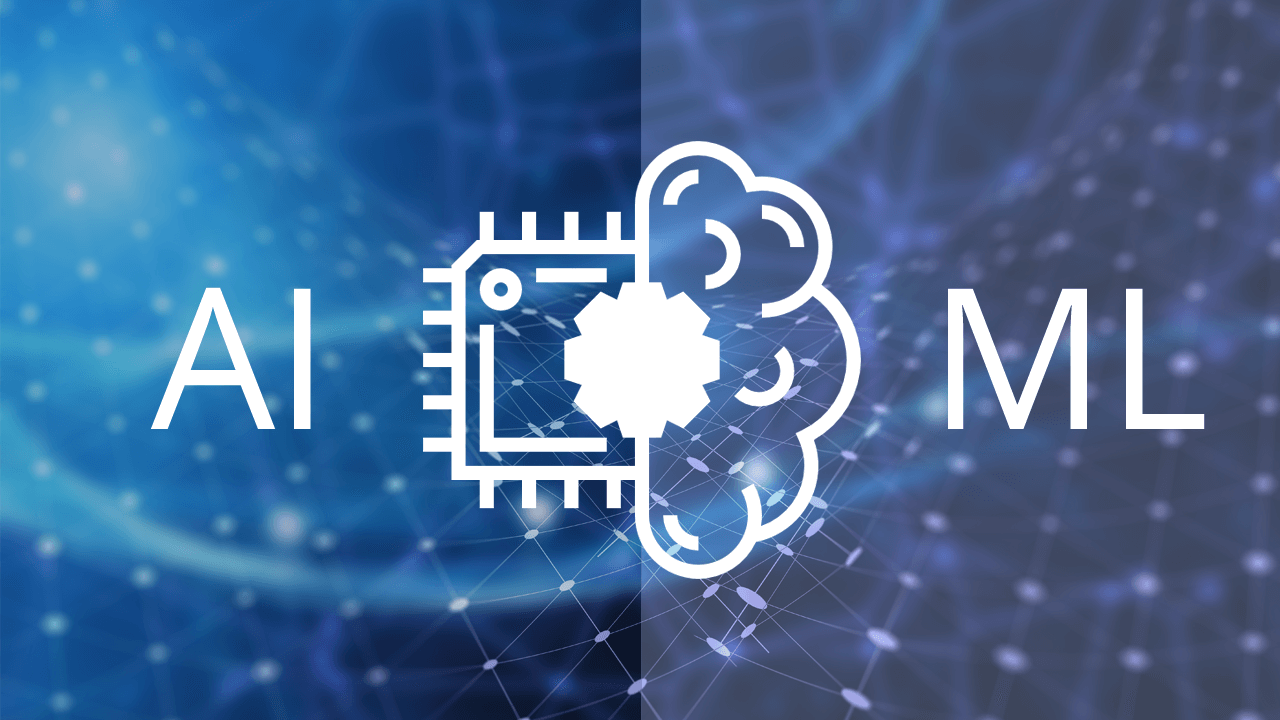
Downloads
Published
How to Cite
Issue
Section
License
Copyright (c) 2023 International Journal of Innovations in Science & Technology

This work is licensed under a Creative Commons Attribution 4.0 International License.