AI Based Predictive Tool-Life Computation in Manufacturing Industry
Keywords:
Predictive maintenance (PdM), cutting factors, tool life, manufacturing industry, computer numerical control (CNC)Abstract
For maximum productivity and optimal utilization of tools, predictive maintenance serves as a standard operation procedure in the manufacturing industry. However, unnecessary or delayed maintenance both causes increased downtime and loss of revenue which should be optimized. Accordingly, this paper presents a method for predicting the maintenance requirement to ensure the optimal utilization of the tools. The experimental data for this research has been collected from a CNC lathe machine in a manufacturing plant for multiple days. The CNC machine equipped with three sensors leads to a detailed log for parameters related to tool wear including current, voltage, acceleration in 3D, motor rpm, and tool temperature respectively. Detailed experimentation has been performed to investigate the importance of different parameters. A direct relationship between current and tool temperature was observed leading to an immediate halt of machine operations. In the subsequent step, maintenance prediction was performed using Logistic regression and Random Forest technique respectively to validate the machine behavior. The retrospective data validated the performance with precise accuracy equal to 98% and 95% for both of methods respectively. The promising results predicting the maintenance schedule of the Lathe machine signify the effectiveness of Machine Learning towards advance scheduling for maintenance. The proactive maintenance strategy helps in potential benefits such as avoiding further costs, avoidance of disruptions, and increased efficiency productivity, thereby enhancing tool life cycles.
References
B. hu Li, B. cun Hou, W. tao Yu, X. bing Lu, and C. wei Yang, “Applications of artificial intelligence in intelligent manufacturing: a review,” Front. Inf. Technol. Electron. Eng., vol. 18, no. 1, pp. 86–96, Jan. 2017, doi: 10.1631/FITEE.1601885/FIGURES/3.
Z. M. Çinar, A. A. Nuhu, Q. Zeeshan, O. Korhan, M. Asmael, and B. Safaei, “Machine Learning in Predictive Maintenance towards Sustainable Smart Manufacturing in Industry 4.0,” Sustain. 2020, Vol. 12, Page 8211, vol. 12, no. 19, p. 8211, Oct. 2020, doi: 10.3390/SU12198211.
K. A. Nguyen, P. Do, and A. Grall, “Multi-level predictive maintenance for multi-component systems,” Reliab. Eng. Syst. Saf., vol. 144, pp. 83–94, Dec. 2015, doi: 10.1016/J.RESS.2015.07.017.
E. Traini, G. Bruno, G. D’Antonio, and F. Lombardi, “Machine Learning Framework for Predictive Maintenance in Milling,” IFAC-PapersOnLine, vol. 52, no. 13, pp. 177–182, Jan. 2019, doi: 10.1016/J.IFACOL.2019.11.172.
J. Krenek, K. Kuca, P. Blazek, O. Krejcar, and D. Jun, “Application of artificial neural networks in condition based predictive maintenance,” Stud. Comput. Intell., vol. 642, pp. 75–86, 2016, doi: 10.1007/978-3-319-31277-4_7/COVER.
S. Kaparthi and D. Bumblauskas, “Designing predictive maintenance systems using decision tree-based machine learning techniques,” Int. J. Qual. Reliab. Manag., vol. 37, no. 4, pp. 659–686, Mar. 2020, doi: 10.1108/IJQRM-04-2019-0131/FULL/XML.
M. Y. You, F. Liu, W. Wang, and G. Meng, “Statistically planned and individually improved predictive maintenance management for continuously monitored degrading systems,” IEEE Trans. Reliab., vol. 59, no. 4, pp. 744–753, Dec. 2010, doi: 10.1109/TR.2010.2085572.
R. K. Mobley, An introduction to predictive maintenance. Butterworth-Heinemann, 2002. Accessed: Feb. 16, 2024. [Online]. Available: http://www.sciencedirect.com:5070/book/9780750675314/an-introduction-to-predictive-maintenance
A. Grall, L. Dieulle, C. Bérenguer, and M. Roussignol, “Continuous-time predictive-maintenance scheduling for a deteriorating system,” IEEE Trans. Reliab., vol. 51, no. 2, pp. 141–150, Jun. 2002, doi: 10.1109/TR.2002.1011518.
W. J. Lee, H. Wu, H. Yun, H. Kim, M. B. G. Jun, and J. W. Sutherland, “Predictive Maintenance of Machine Tool Systems Using Artificial Intelligence Techniques Applied to Machine Condition Data,” Procedia CIRP, vol. 80, pp. 506–511, Jan. 2019, doi: 10.1016/J.PROCIR.2018.12.019.
R. C. M. Yam, P. W. Tse, L. Li, and P. Tu, “Intelligent predictive decision support system for condition-based maintenance,” Int. J. Adv. Manuf. Technol., vol. 17, no. 5, pp. 383–391, 2001, doi: 10.1007/S001700170173/METRICS.
T. P. Carvalho, F. A. A. M. N. Soares, R. Vita, R. da P. Francisco, J. P. Basto, and S. G. S. Alcalá, “A systematic literature review of machine learning methods applied to predictive maintenance,” Comput. Ind. Eng., vol. 137, p. 106024, Nov. 2019, doi: 10.1016/J.CIE.2019.106024.
P. Klein and R. Bergmann, “Generation of complex data for AI-based predictive maintenance research with a physical factory model,” ICINCO 2019 - Proc. 16th Int. Conf. Informatics Control. Autom. Robot., vol. 1, pp. 40–50, 2019, doi: 10.5220/0007830700400050.
T. Zonta, C. A. da Costa, R. da Rosa Righi, M. J. de Lima, E. S. da Trindade, and G. P. Li, “Predictive maintenance in the Industry 4.0: A systematic literature review,” Comput. Ind. Eng., vol. 150, p. 106889, Dec. 2020, doi: 10.1016/J.CIE.2020.106889.
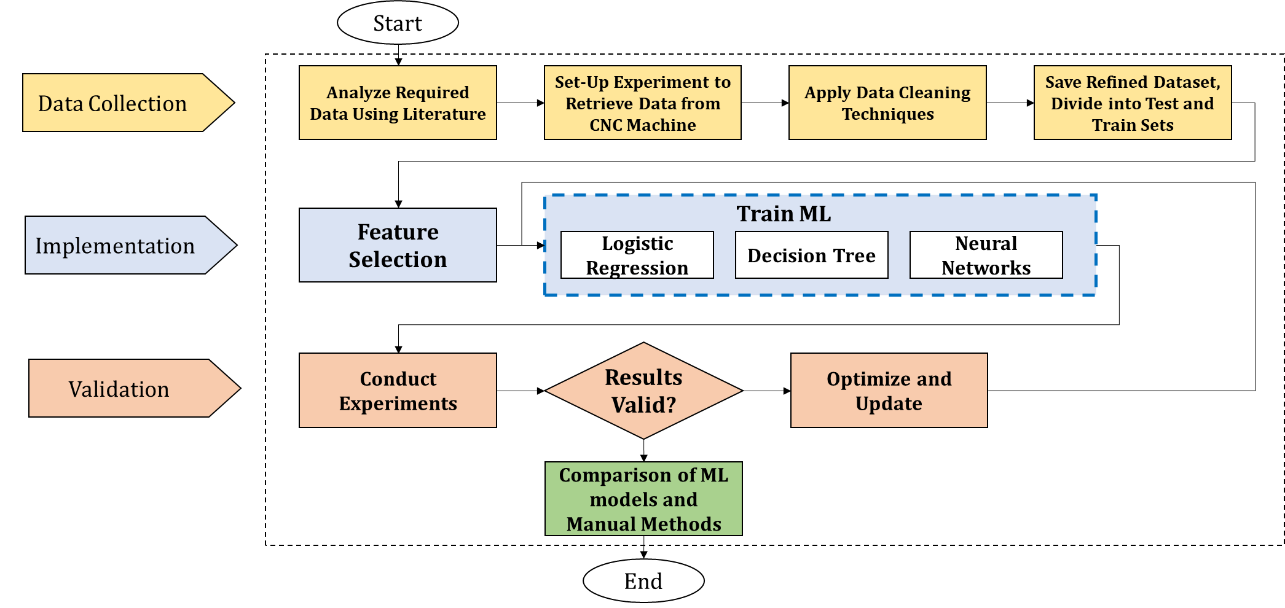
Published
How to Cite
Issue
Section
License
Copyright (c) 2024 50SEA

This work is licensed under a Creative Commons Attribution 4.0 International License.