Deep Learning-based Skin Lesion Segmentation and Classification
Keywords:
Melanoma, Skin cancer, lesion segmentation, lesion classification, image processing, vision transformerAbstract
By using deep learning to automate skin lesion segmentation, this work aims to improve the classification of melanoma. By properly segmenting lesions and utilizing the U-Net algorithm's preprocessing capabilities, our research aims to improve the accuracy of skin cancer diagnosis. During preprocessing, raw dermoscopic pictures from the HAM10000 dataset are enhanced and normalized early. Next, the U-Net model is used to accurately segment lesions. Advanced deep learning approaches are applied after segmentation segmented images are subjected to classification, such as Convolutional Neural Networks (CNN) and Vision Transformer (VIT) models. The VIT model demonstrated a high training accuracy of 0.94, indicating its effectiveness in learning from the training data. However, its validation and testing accuracies were at 0.73. The CNN model showed a training accuracy of 0.95, implying its ability to learn the training data effectively. However, its validation and testing accuracies were at 0.73. This all-encompassing method not only improves dermatological image analysis's dependability and effectiveness, but it also shows promise for enhancing clinical outcomes in the diagnosis and management of different forms of skin cancer. Our work is a significant step toward the creation of more reliable techniques in this important area, opening the door for improvements in patient care and healthcare diagnostics.
References
M. A. Kadampur and S. Al Riyaee, “Skin cancer detection: Applying a deep learning based model driven architecture in the cloud for classifying dermal cell images,” Informatics Med. Unlocked, vol. 18, p. 100282, Jan. 2020, doi: 10.1016/J.IMU.2019.100282.
M. A. Kassem, K. M. Hosny, R. Damaševičius, and M. M. Eltoukhy, “Machine Learning and Deep Learning Methods for Skin Lesion Classification and Diagnosis: A Systematic Review,” Diagnostics 2021, Vol. 11, Page 1390, vol. 11, no. 8, p. 1390, Jul. 2021, doi: 10.3390/DIAGNOSTICS11081390.
R. A. Mehr and A. Ameri, “Skin Cancer Detection Based on Deep Learning,” J. Biomed. Phys. Eng., vol. 12, no. 6, p. 559, Dec. 2022, doi: 10.31661/JBPE.V0I0.2207-1517.
A. Dzieniszewska, P. Garbat, and R. Piramidowicz, “Skin Lesion Classification Based on Segmented Image,” 2023 12th Int. Conf. Image Process. Theory, Tools Appl. IPTA 2023, 2023, doi: 10.1109/IPTA59101.2023.10320004.
R. D. Seeja and A. Suresh, “Deep Learning Based Skin Lesion Segmentation and Classification of Melanoma Using Support Vector Machine (SVM),” Asian Pac. J. Cancer Prev., vol. 20, no. 5, p. 1555, 2019, doi: 10.31557/APJCP.2019.20.5.1555.
R. L. Araujo, R. D. A. L. Rabelo, J. J. P. C. Rodrigues, and R. R. V. E. Silva, “Automatic segmentation of melanoma skin cancer using deep learning,” 2020 IEEE Int. Conf. E-Health Networking, Appl. Serv. Heal. 2020, Mar. 2021, doi: 10.1109/HEALTHCOM49281.2021.9398926.
M. Taghizadeh and K. Mohammadi, “The Fast and Accurate Approach to Detection and Segmentation of Melanoma Skin Cancer using Fine-tuned Yolov3 and SegNet Based on Deep Transfer Learning,” Oct. 2022, Accessed: May 06, 2024. [Online]. Available: https://arxiv.org/abs/2210.05167v2
L. Bi, J. Kim, E. Ahn, A. Kumar, M. Fulham, and D. Feng, “Dermoscopic Image Segmentation via Multistage Fully Convolutional Networks,” IEEE Trans. Biomed. Eng., vol. 64, no. 9, pp. 2065–2074, Sep. 2017, doi: 10.1109/TBME.2017.2712771.
M. Zafar, J. Amin, M. Sharif, M. A. Anjum, G. A. Mallah, and S. Kadry, “DeepLabv3+-Based Segmentation and Best Features Selection Using Slime Mould Algorithm for Multi-Class Skin Lesion Classification,” Math. 2023, Vol. 11, Page 364, vol. 11, no. 2, p. 364, Jan. 2023, doi: 10.3390/MATH11020364.
N. Dimša and A. Paulauskaitė-Tarasevičienė, “Melanoma multi class segmentation using different U-Net type architectures,” 2021.
M. Nawaz et al., “Skin cancer detection from dermoscopic images using deep learning and fuzzy k-means clustering,” Microsc. Res. Tech., vol. 85, no. 1, pp. 339–351, Jan. 2022, doi: 10.1002/JEMT.23908.
A. Ameri, “A Deep Learning Approach to Skin Cancer Detection in Dermoscopy Images,” J. Biomed. Phys. Eng., vol. 10, no. 6, p. 801, 2020, doi: 10.31661/JBPE.V0I0.2004-1107.
G. M. S. Himel, M. M. Islam, K. A. Al-Aff, S. I. Karim, and M. K. U. Sikder, “Skin Cancer Segmentation and Classification Using Vision Transformer for Automatic Analysis in Dermatoscopy-Based Noninvasive Digital System,” Int. J. Biomed. Imaging, vol. 2024, 2024, doi: 10.1155/2024/3022192.
P. Tschandl, C. Rosendahl, and H. Kittler, “The HAM10000 dataset, a large collection of multi-source dermatoscopic images of common pigmented skin lesions,” Sci. Data 2018 51, vol. 5, no. 1, pp. 1–9, Aug. 2018, doi: 10.1038/sdata.2018.161.
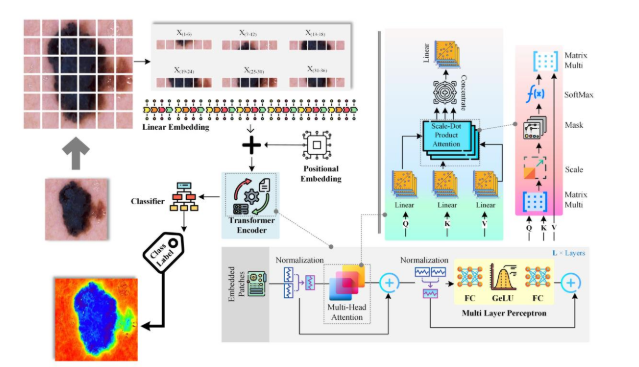
Downloads
Published
How to Cite
Issue
Section
License
Copyright (c) 2024 50SEA

This work is licensed under a Creative Commons Attribution 4.0 International License.