AI-Driven Weed Classification for Improved Cotton Farming in Sindh, Pakistan
Keywords:
Precision agriculture, Weed detection, Machine learning, IoT, CottonWeedsAbstract
This research study proclaims the combination of artificial intelligence and also IoT in precision agriculture, highlighting weed discovery plus cotton plant monitoring in Sindh, Pakistan. The uniqueness lies in creating a deep learning-based computer system vision application to develop a durable real-time weed category system, dealing with a problem not formerly solved. The study entailed gathering datasets utilizing mobile cams under varied ecological problems. A CNN version was educated utilizing the open-source Cotton Weeds dataset, annotated with clinical problems such as Broadleaf and Horse Purslane. Examinations used a Wireless Visual Sensor Network (WVSN) with Raspberry Pi for real-time photo catching as well as category. The CNN version, readjusted to identify in between cotton along with Horse Purslane weed accomplished a precision of 86% and also an ROC AUC rating of 0.93. Efficiency metrics consisting of precision-recall, as well as F1 rating, suggest the model's viability for various other weed category jobs. Nonetheless, obstacles such as photo top-quality variants and also equipment constraints were kept in mind. The research ends that using artificial intelligence as well as IoT in farming can dramatically improve plant return plus assist lasting methods for future generations.
References
“From potentials to reality: transforming Africa’s food production: investment and policy priorities for sufficient, nutritious and sustainable food supplies.” Accessed: Jun. 15, 2024. [Online]. Available: https://www.idos-research.de/en/others-publications/article/from-potentials-to-reality-transforming-africas-food-production-investment-and-policy-priorities-for-sufficient-nutritious-and-sustainable-food-supplies/
G. P. Rao and M. G. Reddy, “Overview of yield losses due to plant viruses,” Appl. Plant Virol. Adv. Detect. Antivir. Strateg., pp. 531–562, Jan. 2020, doi: 10.1016/B978-0-12-818654-1.00038-4.
X. Jin et al., “Machine Learning for Detection and Prediction of Crop Diseases and Pests: A Comprehensive Survey,” Agric. 2022, Vol. 12, Page 1350, vol. 12, no. 9, p. 1350, Sep. 2022, doi: 10.3390/AGRICULTURE12091350.
R. Gerhards, D. Andújar Sanchez, P. Hamouz, G. G. Peteinatos, S. Christensen, and C. Fernandez-Quintanilla, “Advances in site-specific weed management in agriculture—A review,” Weed Res., vol. 62, no. 2, pp. 123–133, Apr. 2022, doi: 10.1111/WRE.12526.
F. López-Granados, “Weed detection for site-specific weed management: mapping and real-time approaches,” Weed Res., vol. 51, no. 1, pp. 1–11, Feb. 2011, doi: 10.1111/J.1365-3180.2010.00829.X.
J. Woodard, “Rapid landscaping of artificial intelligence in agriculture in Pakistan,” USAID Bur. Resilience, Environ. Food Secur., 2023.
M. Tahir Rajput, S. Saleha Tahir, B. Ahmed, and M. Aslam Arain, “CHECK LIST OF THE WEEDS FOUND IN COTTON CROPS, CULTIVATED IN TALUKA UBAURO, DISTRICT SUKKUR, PAKISTAN,” Pak. J. Bot, vol. 40, no. 1, pp. 65–70, 2008.
K. Abas, K. Obraczka, and L. Miller, “Solar-powered, wireless smart camera network: An IoT solution for outdoor video monitoring,” Comput. Commun., vol. 118, pp. 217–233, Mar. 2018, doi: 10.1016/J.COMCOM.2018.01.007.
J. A. Wani, S. Sharma, M. Muzamil, S. Ahmed, S. Sharma, and S. Singh, “Machine Learning and Deep Learning Based Computational Techniques in Automatic Agricultural Diseases Detection: Methodologies, Applications, and Challenges,” Arch. Comput. Methods Eng., vol. 29, no. 1, pp. 641–677, Jan. 2022, doi: 10.1007/S11831-021-09588-5/METRICS.
N. A. Mohidem et al., “How Can Unmanned Aerial Vehicles Be Used for Detecting Weeds in Agricultural Fields?,” Agric. 2021, Vol. 11, Page 1004, vol. 11, no. 10, p. 1004, Oct. 2021, doi: 10.3390/AGRICULTURE11101004.
N. Rai et al., “Applications of deep learning in precision weed management: A review,” Comput. Electron. Agric., vol. 206, p. 107698, Mar. 2023, doi: 10.1016/J.COMPAG.2023.107698.
K. Jiang, U. Afzaal, and J. Lee, “Transformer-Based Weed Segmentation for Grass Management,” Sensors 2023, Vol. 23, Page 65, vol. 23, no. 1, p. 65, Dec. 2022, doi: 10.3390/S23010065.
R. Gao et al., “Intelligent Cotton Pest and Disease Detection: Edge Computing Solutions with Transformer Technology and Knowledge Graphs,” Agric. 2024, Vol. 14, Page 247, vol. 14, no. 2, p. 247, Feb. 2024, doi: 10.3390/AGRICULTURE14020247.
M. I. Younas, M. J. Iqbal, A. Aziz, and A. H. Sodhro, “Toward QoS Monitoring in IoT Edge Devices Driven Healthcare—A Systematic Literature Review,” Sensors 2023, Vol. 23, Page 8885, vol. 23, no. 21, p. 8885, Nov. 2023, doi: 10.3390/S23218885.
M. N. Mowla, N. Mowla, A. F. M. S. Shah, K. M. Rabie, and T. Shongwe, “Internet of Things and Wireless Sensor Networks for Smart Agriculture Applications: A Survey,” IEEE Access, vol. 11, pp. 145813–145852, 2023, doi: 10.1109/ACCESS.2023.3346299.
E. M. B. M. Karunathilake, A. T. Le, S. Heo, Y. S. Chung, and S. Mansoor, “The Path to Smart Farming: Innovations and Opportunities in Precision Agriculture,” Agric. 2023, Vol. 13, Page 1593, vol. 13, no. 8, p. 1593, Aug. 2023, doi: 10.3390/AGRICULTURE13081593.
P. Bhagat, S., Mehta, “Internet of Things (IoT) in Agriculture: Recent Trends, Challenges, and Future Scope,” J. Inf. Technol. Res., vol. 14, no. 3, pp. 23–37, 2021, doi: 10.4018/JITR.2021070102.
M. Alam, M., Kabir, “IoT-Based Smart Farming System Using Raspberry Pi, LoRaWAN, and Solar Power.,” J. Agric. Informatics, vol. 14, no. 1, pp. 30–45, 2023, doi: 10.17700/jai.2023.14.1.626.
S. Mandal, A., Ghosh, “Smart Farming: IoT-based Weed Detection System using Raspberry Pi,” J. Intell. Agric., vol. 14, no. 3, pp. 159–168, 2022, doi: 10.1016/j.jia.2022.01.014.
R. Bhattacharya, A., Ghosh, “Utilization of Machine Learning for Weed Detection in Cotton Fields using CottonWeeds Dataset,” Agric. Informatics J., vol. 18, no. 2, pp. 115–129, 2023, doi: 10.17700/aij.2023.18.2.842.
M. Garcia, C., Torres, “Practical Applications of MobileNet in IoT-Based Smart Agriculture Systems,” IEEE Internet Things J., vol. 8, no. 9, pp. 7432–7440, 2021, doi: 10.1109/JIOT.2020.3034164.
A. Roy, “Performance Analysis and Comparison of Machine and Deep Learning Algorithms for IoT Data Classification,” Int. J. Adv. Comput. Sci. Appl., 2023.
D. Ren, W. Yang, Z. Lu, D. Chen, and H. Shi, “Improved Weed Detection in Cotton Fields Using Enhanced YOLOv8s with Modified Feature Extraction Modules,” Symmetry 2024, Vol. 16, Page 450, vol. 16, no. 4, p. 450, Apr. 2024, doi: 10.3390/SYM16040450.
R. Ali, M., Khan, “Leveraging mobile technology for crop monitoring and data collection in rural Pakistan,” Agric. Syst., 2024, [Online]. Available: https://doi.org/10.1016/j.agsy.2023.103591
X. Zhao, L. Wang, Y. Zhang, X. Han, M. Deveci, and M. Parmar, “A review of convolutional neural networks in computer vision,” Artif. Intell. Rev., vol. 57, no. 4, pp. 1–43, Apr. 2024, doi: 10.1007/S10462-024-10721-6/FIGURES/33.
“GitHub - MIYM5/cotton-purslane-classification: This repository contains the code and resources for a machine learning project aimed at classifying images of Cotton and Purslane (Weed) using a Convolutional Neural Network (CNN).” Accessed: Jun. 15, 2024. [Online]. Available: https://github.com/MIYM5/cotton-purslane-classification
Y. Zhang, Z., Liu, “Evaluating Machine Learning Models in Agricultural Classification Using ROC AUC Metrics,” Comput. Electron. Agric., vol. 204, 2023, [Online]. Available: https://doi.org/10.1016/j.compag.2022.107352
A. Smith, J., Doe, “Precision and Recall in Agricultural Classification Models: A Comprehensive Analysis,” J. Agric. Informatics, vol. 15, no. 3, pp. 205–218, 2023.
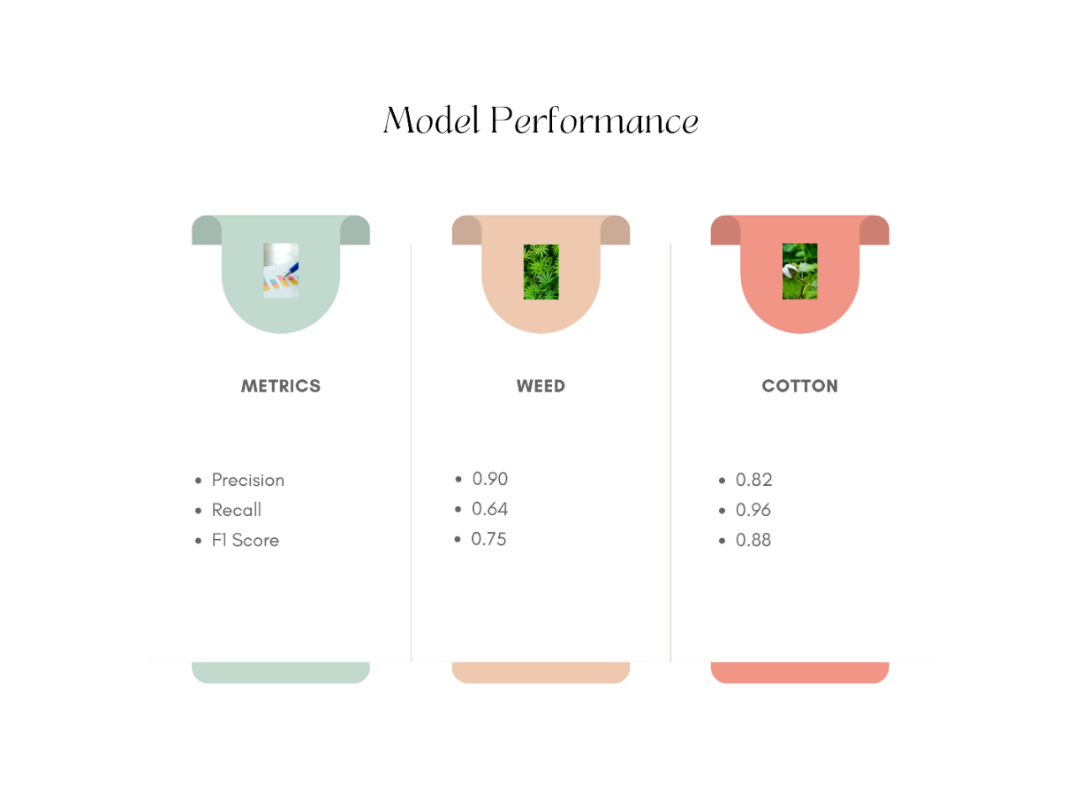
Downloads
Published
How to Cite
Issue
Section
License
Copyright (c) 2024 50SEA

This work is licensed under a Creative Commons Attribution 4.0 International License.