Using Spatial Covariance of Geometric and Shape Based Features for Recognition of Basic and Compound Emotions
Keywords:
compound emotions, fiducial points, shape features, position based features, SVMAbstract
Introduction. Compound emotion recognition has been an emerging area of research for the last decade due to its vast applications in surveillance systems, suspicious person detection, detection of mental disorders, pain detection, automated patient observation in hospitals, and driver monitoring.
Objectives: This study focuses on emotions, highlighting the fact that the existing knowledge lacks adequate research on compound emotions. This research work emphasizes compound emotions along with basic emotions.
Novelty Statement: The contribution of this paper is three-fold. The study proposes an approach relying on geometric and shape-based features using SVM and then fusing the obtained geometric and shape-based features for both basic as well as compound emotion recognition.
Materials and Method: This study provides a comparison with six state-of-the-art approaches in terms of percentage accuracy and time.
Dataset: The experiments are performed on a publicly available compound emotion recognition dataset that contains images with facial fiducial points and action units.
Result and Discussion: The results show that the proposed approach outperforms the existing approaches. The best accuracy achieved is 98.57% and 77.33% for basic and compound emotion recognition, respectively. The proposed approach is compared with existing state-of-the-art deep Neural Network architecture. The comparison of the proposed approach has been extended further to various existing classifiers both in terms of percentage accuracy and time.
Concluding Remarks: The extensive experiments reveal that the proposed approach using SVM outperforms the state-of-the-art deep Neural network architecture and existing classifiers including Naive Bayes, AdaBoost, Decision Table, NNge, and J48.
References
J. Mesken, Determinants and consequences of drivers’ emotions. Stichting Wetenschappelijk Onderzoek Verkeersveiligheid SWOV, 2006.
S. Du, Y. Tao, and A. M. Martinez, “Compound facial expressions of emotion,” Proceedings of the National Academy of Sciences, vol. 111, no. 15, pp. E1454–E1462, 2014.
K. Ghanem, A. Caplier, and M. Kholladi, “Intensity estimation of unknown expression based on a study of facial permanent features deformations,” Courrier DU Savoir, pp. 103–111, 2012.
L. Aarts and I. Van Schagen, “Driving speed and the risk of road crashes: A review,” Accident Analysis & Prevention, vol. 38, no. 2, pp. 215–224, 2006.
N. H. T. S. Administration et al., “Traffic safety facts, 2011: a compi lation of motor vehicle crash data from the fatality analysis reporting system and the general estimates system. dot hs 811754,” Department of Transportation, Washington, DC, 2013.
M. Chan and A. Singhal, “The emotional side of cognitive distraction: Implications for road safety,” Accident Analysis & Prevention, vol. 50, pp. 147–154, 2013.
G. Rebolledo-Mendez, A. Reyes, S. Paszkowicz, M. C. Domingo, and L. Skrypchuk, “Developing a body sensor network to detect emotions during driving,” IEEE transactions on intelligent transportation systems, vol. 15, no. 4, pp. 1850–1854, 2014.
J. L. Deffenbacher, D. M. Deffenbacher, R. S. Lynch, and T. L. Richards, “Anger, aggression, and risky behavior: a comparison of high and low anger drivers,” Behaviour research and therapy, vol. 41, no. 6, pp. 701–718, 2003.
G. Underwood, P. Chapman, S. Wright, and D. Crundall, “Anger while driving,” Transportation Research Part F: Traffic Psychology and Behaviour, vol. 2, no. 1, pp. 55–68, 1999.
Y. King and D. Parker, “Driving violations, aggression and perceived consensus,” Revue Europeenne de Psychologie Appliqu ´ ee/European ´ Review of Applied Psychology, vol. 58, no. 1, pp. 43–49, 2008.
N. Dibben and V. J. Williamson, “An exploratory survey of in-vehicle music listening,” Psychology of Music, vol. 35, no. 4, pp. 571–589, 2007.
D. Keltner, P. Ekman, G. C. Gonzaga, and J. Beer, “Facial expression of emotion.” 2003.
P. Ekman and W. V. Friesen, “Measuring facial movement,” Environ mental psychology and nonverbal behavior, vol. 1, no. 1, pp. 56–75, 1976.
M. Pantic and I. Patras, “Dynamics of facial expression: recognition of facial actions and their temporal segments from face profile image sequences,” IEEE Transactions on Systems, Man, and Cybernetics, Part B (Cybernetics), vol. 36, no. 2, pp. 433–449, 2006.
J. Wang, L. Yin, X. Wei, and Y. Sun, “3d facial expression recognition based on primitive surface feature distribution,” in Computer Vision and Pattern Recognition, 2006 IEEE Computer Society Conference on, vol. 2. IEEE, 2006, pp. 1399–1406.
L. Yin, X. Wei, Y. Sun, J. Wang, and M. J. Rosato, “A 3d facial expression database for facial behavior research,” in Automatic face and gesture recognition, 2006. FGR 2006. 7th international conference on. IEEE, 2006, pp. 211–216.
P. N. Tumpi, W. R. Rahman, M. H. Ali et al., “An efficient facial expression detection system,” Machine Graphics and Vision, vol. 16, no. 3/4, pp. 377–399, 2007.
A. Besinger, T. Sztynda, S. Lal, C. Duthoit, J. Agbinya, B. Jap, D. Eager, and G. Dissanayake, “Optical flow based analyses to detect emotion from human facial image data,” Expert Systems with Applications, vol. 37, no. 12, pp. 8897–8902, 2010.
J.-C. Chun and O. Kwon, “A vision-based approach for facial expression cloning by facial motion tracking,” KSII Transactions on Internet and Information Systems (TIIS), vol. 2, no. 2, pp. 120–133, 2008.
Y. Tong, Y. Shen, B. Gao, F. Sun, R. Chen, and Y. Xu, “A noisy robust approach for facial expression recognition.” KSII Transactions on Internet & Information Systems, vol. 11, no. 4, 2017.
C. Shan, S. Gong, and P. W. McOwan, “Facial expression recognition based on local binary patterns: A comprehensive study,” Image and Vision Computing, vol. 27, no. 6, pp. 803–816, 2009.
H. Soyel and H. Demirel, “Facial expression recognition using 3d facial feature distances,” Image Analysis and Recognition, pp. 831–838, 2007.
P. Lucey, J. F. Cohn, T. Kanade, J. Saragih, Z. Ambadar, and I. Matthews, “The extended cohn-kanade dataset (ck+): A complete dataset for action unit and emotion-specified expression,” in Computer Vision and Pattern Recognition Workshops (CVPRW), 2010 IEEE Computer Society Conference on. IEEE, 2010, pp. 94–101.
N. Sarode and S. Bhatia, “Facial expression recognition,” International Journal on computer science and Engineering, vol. 2, no. 5, pp. 1552–1557, 2010.
N. Neggaz, M. Besnassi, and A. Benyettou, “Facial expression recog nition,” Journal of applied sciences, vol. 10, no. 15, pp. 1572–1579, 2010.
J. L. Raheja and U. Kumar, “Hum an facial expression detection from detected in captured im age using back propagation neural network,”2010.
N. A. Tawhid, N. U. Laskar, and H. Ali, “A vision-based facial expression recognition and adaptation system from video stream,” International Journal of Machine Learning and Computing, vol. 2,
no. 5, p. 535, 2012.
A. A. Gunawan et al., “Face expression detection on kinect using active appearance model and fuzzy logic,” Procedia Computer Science, vol. 59, pp. 268–274, 2015.
A. Martinez and S. Du, “A model of the perception of facial expressions of emotion by humans: Research overview and perspectives,” Journal of Machine Learning Research, vol. 13, no. May, pp. 1589–1608, 2012.
G. Littlewort, M. S. Bartlett, I. Fasel, J. Susskind, and J. Movellan, “Dynamics of facial expression extracted automatically from video,” Image and Vision Computing, vol. 24, no. 6, pp. 615–625, 2006.
S. Li, W. Zheng, Y. Zong, C. Lu, C. Tang, X. Jiang, J. Liu, and W. Xia, “Bi-modality fusion for emotion recognition in the wild,” in 2019 International Conference on Multimodal Interaction, 2019, pp.
–594.
P. S. Aleksic and A. K. Katsaggelos, “Automatic facial expression recognition using facial animation parameters and multistream hmms,” IEEE Transactions on Information Forensics and Security, vol. 1, no. 1, pp. 3–11, 2006.
N. Sebe, M. S. Lew, Y. Sun, I. Cohen, T. Gevers, and T. S. Huang, “Authentic facial expression analysis,” Image and Vision Computing, vol. 25, no. 12, pp. 1856–1863, 2007.
O. J. Urizar, L. Marcenaro, C. S. Regazzoni, E. I. Barakova, and M. Rauterberg, “Emotion estimation in crowds: the interplay of mo tivations and expectations in individual emotions,” in 26th European Signal Processing Conference (EUSIPCO). IEEE, 2018, pp. 1092–1096.
P. I. Rani and K. Muneeswaran, “Emotion recognition based on facial components,” Sadhan ¯ a¯, vol. 43, no. 3, p. 48, 2018.
C. Fabian Benitez-Quiroz, R. Srinivasan, and A. M. Martinez, “Emotionet: An accurate, real-time algorithm for the automatic annotation of a million facial expressions in the wild,” in Proceedings of the IEEE Conference on Computer Vision and Pattern Recognition, 2016, pp. 5562–5570.
A. Azman, Q. Meng, E. A. Edirisinghe, and H. Azman, “Non-intrusive physiological measurement for driver cognitive distraction detection: Eye and mouth movements,” International Journal of Advanced Computer Science, vol. 1, no. 3, pp. 92–99, 2011.
Y.-G. Jiang, B. Xu, and X. Xue, “Predicting emotions in user-generated videos.” in AAAI, 2014, pp. 73–79.
T. Kanade, J. F. Cohn, and Y. Tian, “Comprehensive database for facial expression analysis,” in Automatic Face and Gesture Recognition, 2000. Proceedings. Fourth IEEE International Conference on. IEEE, 2000, pp. 46–53.
A. Savran, N. Alyuz, H. Dibeklio ¨ glu, O. C¸ eliktutan, B. G ˘ okberk, ¨ B. Sankur, and L. Akarun, “Bosphorus database for 3d face analysis,” Biometrics and identity management, pp. 47–56, 2008.
M. F. Valstar and M. Pantic, “Fully automatic recognition of the temporal phases of facial actions,” IEEE Transactions on Systems, Man, and Cybernetics, Part B (Cybernetics), vol. 42, no. 1, pp. 28–43, 2012.
K. Zhao, W.-S. Chu, F. De la Torre, J. F. Cohn, and H. Zhang, “Joint patch and multi-label learning for facial action unit detection,” in Proceedings of the IEEE Conference on Computer Vision and Pattern Recognition, 2015, pp. 2207–2216.
S. Achiche and S. Ahmed, “Mapping shape geometry and emotions using fuzzy logic,” in Proceedings from ASME 2008 International De sign Engineering Technical Conferences & Computers and Information in Engineering Conference, 2008.
F. Tang and B. Deng, “Facial expression recognition using aam and local facial features,” in Natural Computation, 2007. ICNC 2007. Third International Conference on, vol. 2. IEEE, 2007, pp. 632–635.
E. Cambria, I. Hupont, A. Hussain, E. Cerezo, and S. Baldassarri, “Sentic avatar: Multimodal affective conversational agent with common sense,” in Toward Autonomous, Adaptive, and Context-Aware Multimodal Interfaces. Theoretical and Practical Issues. Springer, 2011, pp. 81–95.
L. M. Bergasa, J. Nuevo, M. A. Sotelo, R. Barea, and M. E. Lopez, “Real-time system for monitoring driver vigilance,” IEEE Transactions on Intelligent Transportation Systems, vol. 7, no. 1, pp. 63–77, 2006.
K. Yao, W. Lin, C.-Y. Fang, J. M. Wang, S.-L. Chang, and S.-W. Chen, “Real-time vision-based driver drowsiness/fatigue detection system,” in Vehicular Technology Conference (VTC 2010-Spring), 2010 IEEE 71st. IEEE, 2010, pp. 1–5.
A. M. Mart´ınez, “Recognizing imprecisely localized, partially occluded, and expression variant faces from a single sample per class,” IEEE Transactions on Pattern analysis and machine intelligence, vol. 24,
no. 6, pp. 748–763, 2002.
E. Osuna, R. Freund, and F. Girosi, “An improved training algorithm for support vector machines,” in Neural Networks for Signal Processing [1997] VII. Proceedings of the 1997 IEEE Workshop. IEEE, 1997, pp. 276–285.
H. Foroughi, B. S. Aski, and H. Pourreza, “Intelligent video surveil lance for monitoring fall detection of elderly in home environments,” in Computer and Information Technology, 2008. ICCIT 2008. 11th International Conference on. IEEE, 2008, pp. 219–224.
M. Bosch-Jorge, A.-J. Sanchez-Salmer ´ on, ´ A. Valera, and C. Ricolfe- ´Viala, “Fall detection based on the gravity vector using a wide-angle camera,” Expert Systems with Applications, vol. 41, no. 17, pp. 7980–7986, 2014.
G. Debard, P. Karsmakers, M. Deschodt, E. Vlaeyen, J. Van den Bergh, E. Dejaeger, K. Milisen, T. Goedeme, T. Tuytelaars, and B. Vanrumste, ´ “Camera based fall detection using multiple features validated with real life video,” in Workshop Proceedings of the 7th International Conference on Intelligent Environments, vol. 10. IOS Press, 2011, pp. 441–450.
M. Yu, Y. Yu, A. Rhuma, S. M. R. Naqvi, L. Wang, and J. A. Chambers, “An online one class support vector machine-based person specific fall detection system for monitoring an elderly individual in a room environment,” IEEE journal of biomedical and health informatics, vol. 17, no. 6, pp. 1002–1014, 2013.
S. Dumais, J. Platt, D. Heckerman, and M. Sahami, “Inductive learning algorithms and representations for text categorization,” in Proceedings of the seventh international conference on Information and knowledge management. ACM, 1998, pp. 148–155.
S. Bouktif, A. Fiaz, A. Ouni, and M. A. Serhani, “Optimal deep learning lstm model for electric load forecasting using feature selection and genetic algorithm: Comparison with machine learning approaches,” Energies, vol. 11, no. 7, p. 1636, 2018.
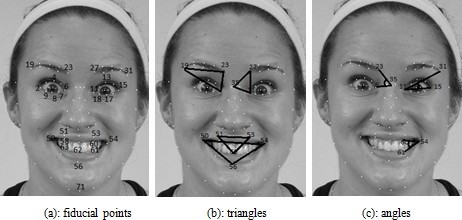
Downloads
Published
How to Cite
Issue
Section
License
Copyright (c) 2024 50SEA

This work is licensed under a Creative Commons Attribution 4.0 International License.