Clinical Prediction of Female Infertility Through Advanced Machine Learning Techniques
Keywords:
Infertility, Machine Learning Techniques, Random Forest, SVM, Logistic Regression and Naïve BayesAbstract
Infertility in females implies failure by such women to conceive even after having at least one year of intercourse without using any contraceptives. Infertility can be caused by a variety of factors, including ovulation problems, blocked fallopian tubes, hormone imbalances, and abnormalities of the uterus and so on. Infertility can negatively impact people's emotional, psychological, and social well-being. Our proposed study utilizes advanced machine learning techniques to present an innovative and novel method for predicting female infertility. We analyzed a dataset with medical attributes related to reproductive health using logistic regression, Naive Bayes, Support Vector Machines (SVM), and Random Forest algorithms. The Random Forest algorithm achieved an outstanding accuracy rate of 93%, with its exceptional capabilities. The findings show that in the future, this model can be used to diagnose infertility early and provide personalized treatment recommendations. The results of this study have practical implications for reproductive healthcare, as well as providing much-needed support to infertile couples and individuals.
References
J. Datta et al., “Prevalence of infertility and help seeking among 15 000 women and men,” Hum. Reprod., vol. 31, no. 9, pp. 2108–2118, Sep. 2016, doi: 10.1093/HUMREP/DEW123.
“Spontaneous Pregnancy and Unexplained Infertility: A Gift With Many Whys”, [Online]. Available: https://www.ncbi.nlm.nih.gov/pmc/articles/PMC3482789/
S. Gameiro et al., “ESHRE guideline: routine psychosocial care in infertility and medically assisted reproduction—a guide for fertility staff,” Hum. Reprod., vol. 30, no. 11, pp. 2476–2485, Nov. 2015, doi: 10.1093/HUMREP/DEV177.
S. G. Vitale, V. L. La Rosa, A. M. C. Rapisarda, and A. S. Laganà, “Psychology of infertility and assisted reproductive treatment: the Italian situation,” J. Psychosom. Obstet. Gynecol., vol. 38, no. 1, pp. 1–3, Jan. 2017, doi: 10.1080/0167482X.2016.1244184.
T. M. Cousineau and A. D. Domar, “Psychological impact of infertility,” Best Pract. Res. Clin. Obstet. Gynaecol., vol. 21, no. 2, pp. 293–308, Apr. 2007, doi: 10.1016/J.BPOBGYN.2006.12.003.
D. Montagu and C. Goodman, “Prohibit, constrain, encourage, or purchase: how should we engage with the private health-care sector?,” Lancet, vol. 388, no. 10044, pp. 613–621, Aug. 2016, doi: 10.1016/S0140-6736(16)30242-2.
E. A. Sullivan et al., “International Committee for Monitoring Assisted Reproductive Technologies (ICMART) world report: assisted reproductive technology 2004,” Hum. Reprod., vol. 28, no. 5, pp. 1375–1390, May 2013, doi: 10.1093/HUMREP/DET036.
S. Berntsen et al., “The health of children conceived by ART: ‘the chicken or the egg?,’” Hum. Reprod. Update, vol. 25, no. 2, pp. 137–158, Mar. 2019, doi: 10.1093/HUMUPD/DMZ001.
T. H. Kappen, Y. Vergouwe, W. A. Van Klei, L. Van Wolfswinkel, C. J. Kalkman, and K. G. M. Moons, “Adaptation of clinical prediction models for application in local settings,” Med. Decis. Mak., vol. 32, no. 3, May 2012, doi: 10.1177/0272989X12439755/ASSET/IMAGES/LARGE/10.1177_0272989X12439755-FIG2.JPEG.
A. Mehrjerd, H. Rezaei, S. Eslami, M. B. Ratna, and N. Khadem Ghaebi, “Internal validation and comparison of predictive models to determine success rate of infertility treatments: a retrospective study of 2485 cycles,” Sci. Reports 2022 121, vol. 12, no. 1, pp. 1–11, May 2022, doi: 10.1038/s41598-022-10902-9.
J. Wei, D. Xiong, Y. Zhang, J. Zeng, W. Liu, and F. Ye, “Predicting ovarian responses to the controlled ovarian hyperstimulation in elderly infertile women using clinical measurements and random forest regression,” Eur. J. Obstet. Gynecol. Reprod. Biol., vol. 288, pp. 153–159, Sep. 2023, doi: 10.1016/j.ejogrb.2023.07.012.
S. Naseem et al., “DeepFert: An Intelligent Fertility Rate Prediction Approach for Men Based on Deep Learning Neural Networks,” IEEE Access, vol. 11, pp. 75006–75022, 2023, doi: 10.1109/ACCESS.2023.3290554.
X. D. Zhang, J. Zhang, R. S. Xie, and W. H. Zhang, “Sexual and reproductive health correlates of polysubstance use among female adolescents who sell sex in the southwest of China,” Subst. Abus. Treat. Prev. Policy, vol. 15, no. 1, pp. 1–15, Aug. 2020, doi: 10.1186/S13011-020-00302-5/TABLES/6.
G. Ozer, A. Akca, B. Yuksel, I. Duzguner, A. C. Pehlivanli, and S. Kahraman, “Prediction of risk factors for first trimester pregnancy loss in frozen-thawed good-quality embryo transfer cycles using machine learning algorithms,” J. Assist. Reprod. Genet., vol. 40, no. 2, pp. 279–288, Feb. 2023, doi: 10.1007/S10815-022-02645-3/METRICS.
C. W. Wang, C. Y. Kuo, C. H. Chen, Y. H. Hsieh, and E. C. Y. Su, “Predicting clinical pregnancy using clinical features and machine learning algorithms in in vitro fertilization,” PLoS One, vol. 17, no. 6, p. e0267554, Jun. 2022, doi: 10.1371/JOURNAL.PONE.0267554.
Z. Chen, Z. Wang, M. Du, and Z. Liu, “Artificial Intelligence in the Assessment of Female Reproductive Function Using Ultrasound: A Review,” J. Ultrasound Med., vol. 41, no. 6, pp. 1343–1353, Jun. 2022, doi: 10.1002/JUM.15827.
B. Tiras and P. O. Cenksoy, “Practice of embryo transfer: Recommendations during and after,” Semin. Reprod. Med., vol. 32, no. 4, pp. 291–296, 2014, doi: 10.1055/S-0034-1375181/ID/JR00891-20/BIB.
L. G. Nardo and S. Chouliaras, “Definitions and Epidemiology of Unexplained Female Infertility,” Unexplained Infertil. Pathophysiol. Eval. Treat., pp. 21–25, Jan. 2015, doi: 10.1007/978-1-4939-2140-9_4.
M. Ogawa, K. Takamatsu, and F. Horiguchi, “Evaluation of factors associated with the anxiety and depression of female infertility patients,” Biopsychosoc. Med., vol. 5, no. 1, pp. 1–5, Dec. 2011, doi: 10.1186/1751-0759-5-15/TABLES/1.
S. Vats, A. Sengupta, A. Chaurasia, and P. Narad, “Combination of Expression Data and Predictive Modelling for Polycystic Ovary Disease and Assessing Risk of Infertility Using Machine Learning Techniques,” pp. 547–555, 2022, doi: 10.1007/978-981-19-0475-2_48.
M. S. Simi, K. S. Nayaki, M. Parameswaran, and S. Sivadasan, “Exploring female infertility using predictive analytic,” GHTC 2017 - IEEE Glob. Humanit. Technol. Conf. Proc., vol. 2017-January, pp. 1–6, Dec. 2017, doi: 10.1109/GHTC.2017.8239343.
R. Wang et al., “AI-Based Optimal Treatment Strategy Selection for Female Infertility for First and Subsequent IVF-ET Cycles,” J. Med. Syst., vol. 47, no. 1, pp. 1–16, Dec. 2023, doi: 10.1007/S10916-023-01967-8/METRICS.
A. Tsujimura et al., “Impact of age, follicle stimulating hormone and Johnsen’s score on successful sperm retrieval by microdissection testicular sperm extraction,” Reprod. Med. Biol., vol. 4, no. 1, pp. 53–57, 2005, doi: 10.1111/J.1447-0578.2005.00082.X.
K. K. Vaegter, T. G. Lakic, M. Olovsson, L. Berglund, T. Brodin, and J. Holte, “Which factors are most predictive for live birth after in vitro fertilization and intracytoplasmic sperm injection (IVF/ICSI) treatments? Analysis of 100 prospectively recorded variables in 8,400 IVF/ICSI single-embryo transfers,” Fertil. Steril., vol. 107, no. 3, pp. 641-648.e2, Mar. 2017, Accessed: Jul. 11, 2024. [Online]. Available: http://www.fertstert.org/article/S001502821663073X/fulltext
“Predictors of pregnancy and live-birth in couples with unexplained infertility following superovulation-intrauterine insemination”, [Online]. Available: https://www.fertstert.org/article/S0015-0282(15)00803-1/fulltext
A. M. E. Lintsen, D. D. M. Braat, J. D. F. Habbema, J. A. M. Kremer, and M. J. C. Eijkemans, “Can differences in IVF success rates between centres be explained by patient characteristics and sample size?,” Hum. Reprod., vol. 25, no. 1, pp. 110–117, Jan. 2010, doi: 10.1093/HUMREP/DEP358.
L. D. M. Ottosen, U. Kesmodel, J. Hindkjær, and H. J. Ingerslev, “Pregnancy prediction models and eSET criteria for IVF patients - Do we need more information?,” J. Assist. Reprod. Genet., vol. 24, no. 1, pp. 29–36, Jan. 2007, doi: 10.1007/S10815-006-9082-9/METRICS.
M. F. G. Verberg, M. J. C. Eijkemans, N. S. Macklon, E. M. E. W. Heijnen, B. C. J. M. Fauser, and F. J. Broekmans, “Predictors of ongoing pregnancy after single-embryo transfer following mild ovarian stimulation for IVF,” Fertil. Steril., vol. 89, no. 5, pp. 1159–1165, May 2008, Accessed: Jul. 11, 2024. [Online]. Available: http://www.fertstert.org/article/S0015028207011454/fulltext
G. S. Handelman, H. K. Kok, R. V. Chandra, A. H. Razavi, M. J. Lee, and H. Asadi, “eDoctor: machine learning and the future of medicine,” J. Intern. Med., vol. 284, no. 6, pp. 603–619, Dec. 2018, doi: 10.1111/JOIM.12822.
F. Rahimian et al., “Predicting the risk of emergency admission with machine learning: Development and validation using linked electronic health records,” PLOS Med., vol. 15, no. 11, p. e1002695, Nov. 2018, doi: 10.1371/JOURNAL.PMED.1002695.
S. J. Liao et al., “A machine learning-based risk scoring system for infertility considering different age groups,” Int. J. Intell. Syst., vol. 36, no. 3, pp. 1331–1344, Mar. 2021, doi: 10.1002/INT.22344.
“A Predictive Model for the Risk of Infertility in Men Using Machine Learning Algorithms - Journal of Urological Surgery - A Predictive Model for the Risk of Infertility in Men Using Machine Learning Algorithms.” Accessed: Jul. 11, 2024. [Online]. Available: https://jurolsurgery.org/articles/doi/jus.galenos.2022.2021.0134
D. J. McLernon, E. W. Steyerberg, E. R. Te Velde, A. J. Lee, and S. Bhattacharya, “Predicting the chances of a live birth after one or more complete cycles of in vitro fertilisation: population based study of linked cycle data from 113 873 women,” BMJ, vol. 355, 2016, doi: 10.1136/BMJ.I5735.
J. Qiu, P. Li, M. Dong, X. Xin, and J. Tan, “Personalized prediction of live birth prior to the first in vitro fertilization treatment: A machine learning method,” J. Transl. Med., vol. 17, no. 1, pp. 1–8, Sep. 2019, doi: 10.1186/S12967-019-2062-5/FIGURES/4.
M. R. Hassan, S. Al-Insaif, M. I. Hossain, and J. Kamruzzaman, “A machine learning approach for prediction of pregnancy outcome following IVF treatment,” Neural Comput. Appl., vol. 32, no. 7, pp. 2283–2297, Apr. 2020, doi: 10.1007/S00521-018-3693-9/METRICS.
A. Uyar, A. Bener, H. N. Ciray, and M. Bahceci, “ROC Based Evaluation and Comparison of Classifiers for IVF Implantation Prediction,” Lect. Notes Inst. Comput. Sci. Soc. Telecommun. Eng., vol. 27 LNICST, pp. 108–111, 2010, doi: 10.1007/978-3-642-11745-9_17.
L. Nanni, A. Lumini, and C. Manna, “A Data Mining Approach for Predicting the Pregnancy Rate in Human Assisted Reproduction,” Stud. Comput. Intell., vol. 326, pp. 97–111, 2010, doi: 10.1007/978-3-642-16095-0_6.
B. B. de Guimarães, L. Martins, J. L. Metello, F. L. Ferreira, P. Ferreira, and J. M. Fonseca, “Application of Artificial Intelligence Algorithms to Estimate the Success Rate in Medically Assisted Procreation,” Reprod. Med. 2020, Vol. 1, Pages 181-194, vol. 1, no. 3, pp. 181–194, Oct. 2020, doi: 10.3390/REPRODMED1030014.
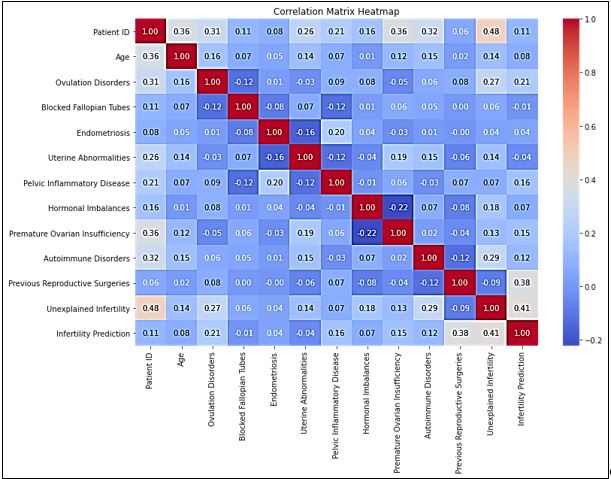
Downloads
Published
How to Cite
Issue
Section
License
Copyright (c) 2024 50SEA

This work is licensed under a Creative Commons Attribution 4.0 International License.