Hybrid Technique for Estimating Fetal Head Circumference Using Ultrasound Imaging
Keywords:
Ultrasound, Imposed images, Head circumference, Residua U-netAbstract
Introduction: Analysis of fetal head shape is crucial for assessing head growth and detecting abnormalities in fetuses. In traditional clinical practice, Head Circumference (HC) is determined by manually fitting an ellipse to the fetal skull based on 2D ultrasound images.
Novelty Statement: To address this, an automated method integrating image processing techniques with U-net variant have been developed to achieve maximum accuracy of fetal head circumference detection on HC18 dataset. This method aims to enhance precision in HC delineation, thereby improving clinical reliability.
Material and Method: This study proposed a method that combines image processing techniques (noise removal, edge detection, segmentation) with a Residual U-net model for detecting the boundary of the fetal skull using HC18 dataset.
Results and Discussion: The results of this method outperformed a simple residual u-net model in terms of accuracy. The proposed method is evaluating using the HC18 challenge dataset, achieving a Dice coefficient of 97.99%, a mean difference of 5.86 mm, and a mean Hausdorff distance of 0.56 mm compared to manual annotations. These results demonstrate the effectiveness of the proposed method in accurately delineating the fetal skull boundary.
Concluding remarks: Furthermore, the proposed method shows comparability with state-of-the-art techniques in the field.
References
D. L. Miller, “Safety Assurance in Obstetrical Ultrasound,” Semin. Ultrasound, CT MRI, vol. 29, no. 2, pp. 156–164, Apr. 2008, doi: 10.1053/J.SULT.2007.12.003.
J. Espinoza, S. Good, E. Russell, and W. Lee, “Does the Use of Automated Fetal Biometry Improve Clinical Work Flow Efficiency?,” J. Ultrasound Med., vol. 32, no. 5, pp. 847–850, May 2013, doi: 10.7863/JUM.2013.32.5.847.
J. Lanowski et al., “Impact of Ultrasound Training and Experience on Accuracy regarding Fetal Weight Estimation at Term Creative Education,” Creat. Educ., vol. 8, no. 11, pp. 1761–1773, Sep. 2017, doi: 10.4236/CE.2017.811120.
“Automated measurement of fetal head circumference”, doi: 10.5281/ZENODO.1322001.
“R. Ramya, K. Srinivasan, K. P. Devi, S. Preethi,... - Google Scholar.” Accessed: Aug. 07, 2024. [Online]. Available: https://scholar.google.com/scholar?hl=en&as_sdt=0%2C5&q=R.+Ramya%2C+K.+Srinivasan%2C+K.+P.+Devi%2C+S.+Preethi%2C+and+G.+Poonkuzhali%2C+“Prenatal+fetal+weight+detection+using+image+processing”%2C+International+Journal+of+Scientific+%26+Technology+Research+2018%3B+7%3A+37–39&btnG=
R. Monica, K. Nirmala, V. J. Kumar, and B. Divya, “Assessment of fetal biometry using ultrasound images,” Proc. 2020 IEEE Int. Conf. Adv. Dev. Electr. Electron. Eng. ICADEE 2020, Dec. 2020, doi: 10.1109/ICADEE51157.2020.9368917.
T. James and S. Thomas, “Segmentation of Head from Ultrasound Fetal Image using Chamfer Matching and Hough Transform based Approaches”, Accessed: Aug. 06, 2024. [Online]. Available: www.ijert.org
W. Lu, J. Tan, and R. C. Floyd, “Fetal head detection and measurement in ultrasound images by an iterative randomized Hough transform,” https://doi.org/10.1117/12.533073, vol. 5370, pp. 557–565, May 2004, doi: 10.1117/12.533073.
K. Thirusittampalam and J. Thangavel, “Fetal Head Detection in 2D Ultrasound Images using Deep Learning,” 2020, Accessed: Aug. 06, 2024. [Online]. Available: http://repo.lib.jfn.ac.lk/ujrr/handle/123456789/3804
“C. Sun, “Automatic fetal head measurements from... - Google Scholar.” Accessed: Aug. 07, 2024. [Online]. Available: https://scholar.google.com/scholar?hl=en&as_sdt=0%2C5&q=C.+Sun%2C+“Automatic+fetal+head+measurements+from+ultrasound+images+using+circular+shortest+paths”.+Proceedings+of+challenge+US%3A+biometric+measurements+from+fetal+ultrasound+images%2C+ISBI+2012%2C+13–15&btnG=
“A multilevel thresholding combined with edge detection and shape-based recognition for segmentation of fetal ultrasound images | Request PDF.” Accessed: Aug. 06, 2024. [Online]. Available: https://www.researchgate.net/publication/312455757_A_multilevel_thresholding_combined_with_edge_detection_and_shape-based_recognition_for_segmentation_of_fetal_ultrasound_images
V. Salari, I. Zador, L. Chik, and R. J. Sokol, “Automated measurements of fetal head from ultrasound images,” https://doi.org/10.1117/12.18907, vol. 1233, pp. 213–216, Jul. 1990, doi: 10.1117/12.18907.
Y. Shen, J. Yu, Y. Shen, and Y. Wang, “Fetal skull analysis in ultrasound images based on iterative randomized Hough transform,” https://doi.org/10.1117/12.811431, vol. 7265, pp. 143–151, Mar. 2009, doi: 10.1117/12.811431.
R. Xu, J. Ohya, B. Zhang, Y. Sato, and M. G. Fujie, “Automatic Fetal Head Detection on Ultrasound Images by An Improved Iterative Randomized Hough Transform”, Accessed: Aug. 07, 2024. [Online]. Available: https://www.researchgate.net/publication/303723203
W. Fathima Farsana, “AutomaticFetal Head Circumference Measurement in 2D Ultrasound Fetal Images using Histogram of Oriented Gradient,” Int. J. Adv. Res. Eng. Technol., vol. 11, no. 12, pp. 484–491, 2020, doi: 10.34218/IJARET.11.12.2020.052.
“Automatic image quality assessment and measurement of fetal head in two-dimensional ultrasound image.” Accessed: Jul. 21, 2024. [Online]. Available: https://www.spiedigitallibrary.org/journals/journal-of-medical-imaging/volume-4/issue-02/024001/Automatic-image-quality-assessment-and-measurement-of-fetal-head-in/10.1117/1.JMI.4.2.024001.full#_=_
K. D. Rahayu, R. Sigit, and D. Agata, “Fetal head and femur detection from USG image to estimate gestational age,” Int. Electron. Symp. Knowl. Creat. Intell. Comput. IES-KCIC 2018 - Proc., pp. 242–247, Jul. 2018, doi: 10.1109/KCIC.2018.8628560.
B. Al-Bander, T. Alzahrani, S. Alzahrani, B. M. Williams, and Y. Zheng, “Improving Fetal Head Contour Detection by Object Localisation with Deep Learning,” Commun. Comput. Inf. Sci., vol. 1065 CCIS, pp. 142–150, 2020, doi: 10.1007/978-3-030-39343-4_12.
J. Zhang, C. Petitjean, P. Lopez, and S. Ainouz, “Direct estimation of fetal head circumference from ultrasound images based on regression CNN,” Proceedings of Machine Learning Research, vol. 121. PMLR, pp. 914–922, Sep. 21, 2020. Accessed: Aug. 06, 2024. [Online]. Available: https://proceedings.mlr.press/v121/zhang20a.html
V. Rajinikanth, N. Dey, R. Kumar, J. Panneerselvam, and N. Sri Madhava Raja, “Fetal Head Periphery Extraction from Ultrasound Image using Jaya Algorithm and Chan-Vese Segmentation,” Procedia Comput. Sci., vol. 152, pp. 66–73, Jan. 2019, doi: 10.1016/J.PROCS.2019.05.028.
R. V. Rao, “Jaya: A simple and new optimization algorithm for solving constrained and unconstrained optimization problems,” Int. J. Ind. Eng. Comput., vol. 7, pp. 19–34, 2016, doi: 10.5267/j.ijiec.2015.8.004.
M. G. Oghli et al., “Automatic Measurement of Fetal Head Biometry from Ultrasound Images using Deep Neural Networks,” 2020 IEEE Nucl. Sci. Symp. Med. Imaging Conf. NSS/MIC 2020, 2020, doi: 10.1109/NSS/MIC42677.2020.9507932.
K. Lp and Muthurasu N, “AUTOMATIC ESTIMATION OF FETAL HEAD CIRCUMFERENCE USING UNET AND HOUGH TRANSFORM,” Int. Res. J. Eng. Technol., 2020, Accessed: Aug. 07, 2024. [Online]. Available: www.irjet.net
Z. Sobhaninia et al., “Fetal Ultrasound Image Segmentation for Measuring Biometric Parameters Using Multi-Task Deep Learning,” Proc. Annu. Int. Conf. IEEE Eng. Med. Biol. Soc. EMBS, pp. 6545–6548, Jul. 2019, doi: 10.1109/EMBC.2019.8856981.
S. Bano et al., “AutoFB: Automating Fetal Biometry Estimation from Standard Ultrasound Planes,” Lect. Notes Comput. Sci. (including Subser. Lect. Notes Artif. Intell. Lect. Notes Bioinformatics), vol. 12907 LNCS, pp. 228–238, 2021, doi: 10.1007/978-3-030-87234-2_22.
T. T. Canh, T. D. Toan, L. M. Hung, and T. Van Lang, “AN AUTOMATIC SYSTEM FOR ESTIMATING FETUS HEAD CIRCUMFERENCE USING 2D ULTRASOUND IMAGES,” Dec. 2021, doi: 10.15625/VAP.2021.0055.
J. J. Cerrolaza et al., “Deep learning with ultrasound physics for fetal skull segmentation,” Proc. - Int. Symp. Biomed. Imaging, vol. 2018-April, pp. 564–567, May 2018, doi: 10.1109/ISBI.2018.8363639.
M. C. Fiorentino, F. P. Villani, M. Di Cosmo, E. Frontoni, and S. Moccia, “A review on deep-learning algorithms for fetal ultrasound-image analysis,” Med. Image Anal., vol. 83, p. 102629, Jan. 2023, doi: 10.1016/J.MEDIA.2022.102629.
X. Yang et al., “Hybrid attention for automatic segmentation of whole fetal head in prenatal ultrasound volumes,” Comput. Methods Programs Biomed., vol. 194, p. 105519, Oct. 2020, doi: 10.1016/J.CMPB.2020.105519.
S. Nie, J. Yu, P. Chen, J. Zhang, and Y. Wang, “A novel method with a deep network and directional edges for automatic detection of a fetal head,” 2015 23rd Eur. Signal Process. Conf. EUSIPCO 2015, pp. 654–658, Dec. 2015, doi: 10.1109/EUSIPCO.2015.7362464.
D. Qiao and F. Zulkernine, “Dilated Squeeze-and-Excitation U-Net for Fetal Ultrasound Image Segmentation,” 2020 IEEE Conf. Comput. Intell. Bioinforma. Comput. Biol. CIBCB 2020, Oct. 2020, doi: 10.1109/CIBCB48159.2020.9277667.
K. Rasheed, F. Junejo, A. Malik, and M. Saqib, “Automated Fetal Head Classification and Segmentation Using Ultrasound Video,” IEEE Access, vol. 9, pp. 160249–160267, 2021, doi: 10.1109/ACCESS.2021.3131518.
A. Ciurte et al., “Semi-supervised segmentation of ultrasound images based on patch representation and continuous min cut,” PLoS One, vol. 9, no. 7, Jul. 2014, doi: 10.1371/JOURNAL.PONE.0100972.
T. L. A. van den Heuvel, H. Petros, S. Santini, C. L. de Korte, and B. van Ginneken, “Automated Fetal Head Detection and Circumference Estimation from Free-Hand Ultrasound Sweeps Using Deep Learning in Resource-Limited Countries,” Ultrasound Med. Biol., vol. 45, no. 3, pp. 773–785, Mar. 2019, doi: 10.1016/j.ultrasmedbio.2018.09.015.
“Image Analysis - Connected Components Labeling.” Accessed: Aug. 07, 2024. [Online]. Available: https://homepages.inf.ed.ac.uk/rbf/HIPR2/label.htm
“Image Processing - an overview | ScienceDirect Topics.” Accessed: Aug. 07, 2024. [Online]. Available: https://www.sciencedirect.com/topics/computer-science/image-processing
N. Kumar and M. Nachamai, “Noise Removal and Filtering Techniques Used in Medical Images,” Orient. J. Comput. Sci. Technol., vol. 10, no. 1, pp. 103–113, Mar. 2017, doi: 10.13005/OJCST/10.01.14.
“Spatial Filters - Median Filter.” Accessed: Aug. 07, 2024. [Online]. Available: https://homepages.inf.ed.ac.uk/rbf/HIPR2/median.htm
“An introduction to image segmentation with k-means clustering”, [Online]. Available: https://www.kdnuggets.com/k-means-clustering.html
“Spatial Filters - Gaussian Smoothing.” Accessed: Aug. 07, 2024. [Online]. Available: https://homepages.inf.ed.ac.uk/rbf/HIPR2/gsmooth.htm
“Image pyramids and the laplacian”, [Online]. Available: https://www.owlnet.rice.edu/wiener.html
“Sobel edge detection”, [Online]. Available: https://www.imageeprocessing.com/2011/12/sobel-edge-detection.html
“Image Processing with Python: Thresholding.” Accessed: Jul. 21, 2024. [Online]. Available: https://datacarpentry.org/image-processing/07-thresholding.html
“Types of Morphological Operations - MATLAB & Simulink.” Accessed: Jul. 21, 2024. [Online]. Available: https://www.mathworks.com/help/images/morphological-dilation-and-erosion.html
Z. Li, F. Liu, W. Yang, S. Peng, and J. Zhou, “A Survey of Convolutional Neural Networks: Analysis, Applications, and Prospects,” IEEE Trans. Neural Networks Learn. Syst., vol. 33, no. 12, pp. 6999–7019, Dec. 2022, doi: 10.1109/TNNLS.2021.3084827.
“Attention U-Net, ResUnet, U-Net++, U2-Net | AIGuys.” Accessed: Jul. 21, 2024. [Online]. Available: https://medium.com/aiguys/attention-u-net-resunet-many-more-65709b90ac8b
G. Zhou, K. Zhong, Z. Li, and Y. Shi, “Direct Least Absolute Deviation Fitting of Ellipses,” Math. Probl. Eng., vol. 2020, no. 1, p. 1317349, Jan. 2020, doi: 10.1155/2020/1317349.
V. Ashkani Chenarlogh et al., “Fast and Accurate U-Net Model for Fetal Ultrasound Image Segmentation,” Ultrason. Imaging, vol. 44, no. 1, pp. 25–38, Jan. 2022, doi: 10.1177/01617346211069882/ASSET/IMAGES/LARGE/10.1177_01617346211069882-FIG6.JPEG.
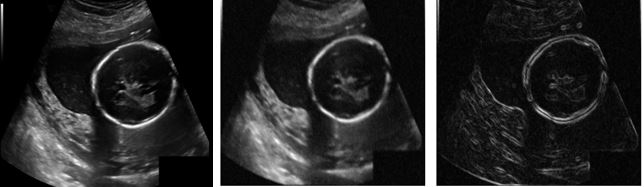
Downloads
Published
How to Cite
Issue
Section
License
Copyright (c) 2024 50SEA

This work is licensed under a Creative Commons Attribution 4.0 International License.