Exploring the Efficacy of CNN Architectures for Esophageal Cancer Classification Using Cell Vizio Images
Keywords:
Barrett's Esophagus, Cell Vizio, Convolutional Neural Network, Dysplasia, Esophageal Cancer, Gastroesophageal Reflux, Res Net 50, Squamous Epithelium.Abstract
Esophageal cancer, as with the global burden of disease, is usually due to Barrret's esophagus and gastroesophageal reflux disease. Fortunately, the disease is amenable to early detection; however, early diagnosis has been complicated by the limitations of the existing diagnostic technologies. To address this problem, a new Convolutional Neural Network and ResNet50 architecture are presented in this study to aid esophageal cancer diagnosis through the classification of Cell Vizio images. This diagnosis is made by the deep learning architecture which does tissue classification into four categories thus improving the diagnostic sensitivity. For model training and testing preoperative perforations in sixty-one patients, 11,161 images were used. Data augmentation and normalization techniques were also performed on the images to help improve the outcome. Our training accuracy reached an impressive 99% 12, while our final f1 score was 93.05%. Our Res Net 50 model obtained an F1 score of 93.26%, precision of 94.05%, recall of 93.52 %, and validation accuracy of 93.32 %. These results indicate how well our deep learning-based technique can be used as a quick, non-embolic, accurate method for early detection of esophageal carcinoma.
References
M. Khieu and S. Mukherjee, “Barrett Esophagus,” StatPearls, Sep. 2024, Accessed: Oct. 12, 2024. [Online]. Available: https://www.ncbi.nlm.nih.gov/books/NBK430979/
C. Q. Liu et al., “Epidemiology of esophageal cancer in 2020 and projections to 2030 and 2040,” Thorac. Cancer, vol. 14, no. 1, pp. 3–11, Jan. 2023, doi: 10.1111/1759-7714.14745.
“Cellvizio® Overview - Mauna Kea Technologies.” Accessed: Oct. 12, 2024. [Online]. Available: https://www.maunakeatech.com/cellvizio-overview/
K. Nakagawa et al., “Classification for invasion depth of esophageal squamous cell carcinoma using a deep neural network compared with experienced endoscopists,” Gastrointest. Endosc., vol. 90, no. 3, pp. 407–414, Sep. 2019, doi: 10.1016/j.gie.2019.04.245.
G. Liu et al., “Automatic classification of esophageal lesions in endoscopic images using a convolutional neural network,” Ann. Transl. Med., vol. 8, no. 7, pp. 486–486, Apr. 2020, doi: 10.21037/ATM.2020.03.24.
Z. Wu et al., “ELNet:Automatic classification and segmentation for esophageal lesions using convolutional neural network,” Med. Image Anal., vol. 67, p. 101838, Jan. 2021, doi: 10.1016/J.MEDIA.2020.101838.
W. Du et al., “Review on the Applications of Deep Learning in the Analysis of Gastrointestinal Endoscopy Images,” IEEE Access, vol. 7, pp. 142053–142069, 2019, doi: 10.1109/ACCESS.2019.2944676.
A. Chempak Kumar and D. M. N. Mubarak, “Ensembled CNN with artificial bee colony optimization method for esophageal cancer stage classification using SVM classifier,” J. Xray. Sci. Technol., vol. 32, no. 1, pp. 31–51, Jan. 2024, doi: 10.3233/XST-230111.
W. Huang et al., “Raman spectroscopy and machine learning for the classification of esophageal squamous carcinoma,” Spectrochim. Acta Part A Mol. Biomol. Spectrosc., vol. 281, p. 121654, Nov. 2022, doi: 10.1016/J.SAA.2022.121654.
“Exploring ResNet50: An In-Depth Look at the Model Architecture and Code Implementation | by Nitish Kundu | Medium.” Accessed: Oct. 12, 2024. [Online]. Available: https://medium.com/@nitishkundu1993/exploring-resnet50-an-in-depth-look-at-the-model-architecture-and-code-implementation-d8d8fa67e46f
“Evaluation Metrics for Classification | by Python Programmer | Medium.” Accessed: Oct. 12, 2024. [Online]. Available: https://medium.com/@mlmind/evaluation-metrics-for-classification-fc770511052d
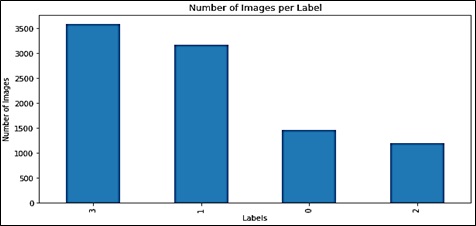
Published
How to Cite
Issue
Section
License
Copyright (c) 2024 50sea

This work is licensed under a Creative Commons Attribution 4.0 International License.