Detection of Coronary Artery Using Novel Optimized Grid Search-based MLP
Keywords:
heart disease, coronary artery narrowness, block vessels, heart attack, deep learning, intelligent systems.Abstract
In recent years, we have witnessed a rapid rise in the mortality rate of people of every age due to cardiac diseases. The diagnosis of heart disease has become a challenging task in present medical research, and it depends upon the history of patients. Rapid advancements in the field of deep learning. Therefore, it is a need to develop an automated system that assists medical experts in their decision-making process. In this work, we proposed a novel optimized grid search-based multi-layer perceptron method to effectively detect heart disease patients earlier and accurately. We evaluated the performance of our method on a dataset named Public Health dataset for heart diseases. More specifically, our method obtained an accuracy of 95.12%, precision of 95.32%, recall of 95.32%, and F1-score of 95.32%. We made a comparison of our method with existing methods to check superiority and robustness of our system to detect heart disease patients. Experimental results along with comprehensive comparison with other methods illustrate that our technique has superior performance and is robust to detect heart disease patients. From the results, we can conclude that our method is reliable to be used in hospitals for the early detection of heart disease patients.
References
. R. Poplin, A. V. Varadarajan, K. Blumer, Y. Liu, M. V. McConnell, G. S. Corrado, L. Peng, and D. R. Webster, ‘‘Prediction of cardiovascular risk factors from retinal fundus photographs via deep learning,’’ Nature Biomed. Eng., vol. 2, no. 3, pp. 158–164, Mar. 2018.
. J. Kim, U. Kang, and Y. Lee, ‘‘Statistics and deep belief network-based cardiovascular risk prediction,’’ Healthcare Inform. Res., vol. 23, no. 3, pp. 169–175, 2017.
. K. M. Z. Hasan, S. Datta, M. Z. Hasan, and N. Zahan, ‘‘Automated prediction of heart disease patients using sparse discriminant analysis,’’ in Proc. Int. Conf. Electr., Comput. Commun. Eng. (ECCE), Feb. 2019, pp. 1–6.
. G. Altan, ‘‘Diagnosis of coronary artery disease using deep belief net- works,’’ Makalenizi Yükleyebilmek için Lütfen İngilizce Dilini Seçiniz!!! EJENS, vol. 2, no. 1, pp. 29–36, 2017.
. R. Alizadehsani, M. Abdar, M. Roshanzamir, A. Khosravi, P. M. Kebria, F. Khozeimeh, S. Nahavandi, N. Sarrafzadegan, and U. R. Acharya, ‘‘Machine learning-based coronary artery disease diagnosis: A comprehensive review,’’ Comput. Biol. Med., vol. 111, Aug. 2019, Art. no. 103346.
. G. Luo, G. Sun, K. Wang, S. Dong, and H. Zhang, ‘‘A novel left ventricular volumes prediction method based on deep learning network in cardiac MRI,’’ in Proc. Comput. Cardiol. Conf. (CinC), Sep. 2016, pp. 89–92.
. A. Caliskan and M. E. Yuksel, ‘‘Classification of coronary artery disease data sets by using a deep neural network,’’ EuroBiotech J., vol. 1, no. 4, pp. 271–277, Oct. 2017.
. N. I. Hasan and A. Bhattacharjee, ‘‘Deep learning approach to cardiovascular disease classification employing modified ECG signal from empirical mode decomposition,’’ Biomed. Signal Process. Control, vol. 52, pp. 128–140, Jul. 2019.
. J. Kwon, K. Kim, K. Jeon, and J. Park, ‘‘Deep learning for predicting in-hospital mortality among heart disease patients based on echocardiography,’’ Echocardiography, vol. 36, no. 2, pp. 213–218, Feb. 2019.
. K. H. Miao and J. H., ‘‘Coronary heart disease diagnosis using deep neural networks,’’ Int. J. Adv. Comput. Sci. Appl., vol. 9, no. 10, pp. 1–8, 2018.
. G.-P. Diller, A. Kempny, S. V. Babu-Narayan, M. Henrichs, M. Brida, A. Uebing, A. E. Lammers, H. Baumgartner, W. Li, S. J. Wort, K. Dimopoulos, and M. A. Gatzoulis, ‘‘Machine learning algorithms estimating prognosis and guiding therapy in adult congenital heart disease: Data from a single tertiary center including 10 019 patients,’’ Eur. Heart J., vol. 40, no. 13, pp. 1069–1077, Apr. 2019.
. A. Junejo, Y. Shen, A. A. Laghari, X. Zhang, and H. Luo, ‘‘Molecular diagnostic and using deep learning techniques for predict functional recovery of patients treated of cardiovascular disease,’’ IEEE Access, vol. 7, pp. 120315–120325, 2019.
. P. Lu, S. Guo, H. Zhang, Q. Li, Y. Wang, Y. Wang, and L. Qi, ‘‘Research on improved depth belief network-based prediction of cardiovascular dis-eases,’’ J. Healthcare Eng., vol. 2018, pp. 1–9, May 2018.
. A. Phalke and S. Sonder, ‘‘Deep learning-based heart disease prediction,’’ Asian J. Converg. Technol. (AJCT), vol. 5, no. 1, pp. 1–4, Apr. 2019.
. R. Jin, ‘‘Predict the risk of cardiovascular diseases in the future using deep learning,’’ Ph.D. dissertation, Dept. Elect. Comput. Eng., Univ. Texas San Antonio, San Antonio, TX, USA, 2018
. S. G. van Velzen, M. Zreik, N. Lessmann, M. A. Viergever, P. A. de Jong, H. M. Verkooijen, and I. Išgum, ‘‘Direct prediction of cardiovascular mortality from low-dose chest CT using deep learning,’’ Proc. SPIE, vol. 10949, Mar. 2019, Art. no. 109490X.
. B. Jin, C. Che, Z. Liu, S. Zhang, X. Yin, and X. Wei, ‘‘Predicting the risk of heart failure with EHR sequential data modeling,’’ IEEE Access, vol. 6, pp. 9256–9261, 2018.
. S. Habib, M. B. Moin, and S. Aziz, ‘‘Heart failure risk prediction and medicine recommendation system using exploratory analysis and big data analytics,’’ Ph.D. dissertation, Dept. Comput. Sci. Eng., BRAC Univ., Dhaka, Bangladesh, 2018.
. N. Meng, P. Zhang, J. Li, J. He, and J. Zhu, ‘‘Prediction of coronary heart disease using routine blood tests,’’ 2018, arXiv:1809.09553. [Online]. Available: http://arxiv.org/abs/1809.09553
. C. Krittanawong, K. W. Johnson, R. S. Rosenson, Z. Wang, M. Aydar, U. Baber, J. K. Min, W. H. W. Tang, J. L. Halperin, and S. M. Narayan, ‘‘Deep learning for cardiovascular medicine: A practical primer,’’ Eur. Heart J., vol. 40, no. 25, pp. 2058–2073, Jul. 2019.
. S. M. Awan, M. U. Riaz, and A. G. Khan, ‘‘Prediction of heart disease using artificial neural networks,’’ VFAST Trans. Softw. Eng., vol. 13, no. 3, pp. 102–112, 2018.
. Deep Learning in Science a Survey of Opportunities and Trends. Accessed: Aug. 2019. [Online]. Available: https://towardsdatascience. com/deep-learning-in-science-fd614bb3f3ce
. N.-S. Tomov and S. Tomov, ‘‘On deep neural networks for detecting heart disease,’’ 2018, arXiv:1808.07168. [Online]. Available: http://arxiv. org/abs/1808.07168
. Baccouche, A., Garcia-Zapirain, B., Castillo Olea, C., & Elmaghraby, A. (2020). Ensemble deep learning models for heart disease classification: A case study from Mexico. Information, 11(4), 207.
. S. Tuli, N. Basumatary, S. S. Gill, M. Kahani, R. C. Arya, G. S. Wander, and R. Buyya, ‘‘HealthFog: An ensemble deep learning-based smart healthcare system for automatic diagnosis of heart diseases in integrated IoT and fog computing environments,’’ Future Gener. Comput. Syst., vol. 104, pp. 187–200, Mar. 2020.
. E. Choi, A. Schuetz, W. F. Stewart, and J. Sun, ‘‘Using recurrent neural network models for early detection of heart failure onset,’’ J. Amer. Med. Information. Assoc., vol. 24, no. 2, pp. 361–370, Mar. 2017.
. R. Das, I. Turkoglu, and A. Sengur, ‘‘Effective diagnosis of heart disease through neural networks ensembles,’’ Expert Syst. Appl., vol. 36, no. 4, pp. 7675–7680, May 2009.
. Access on 10-19-2021, available online at: https://archive.ics.uci.edu/ml/datasets/heart+disease.
. T. Helmy and Z. Rasheed, “Multi-category bioinformatics dataset classification using extreme learning machine,” in Proceedings of the IEEE Congress on Evolutionary Computation (CEC '09), pp. 3234–3240, Trondheim, Norway, May 2009.
. S.-J. Wang, A. Mathew, Y. Chen, L.-F. Xi, L. Ma, and J. Lee, “Empirical analysis of support vector machine ensemble classifiers,” Expert Systems with Applications, vol. 36, no. 3, pp. 6466–6476, 2009.
. S. Özşen and S. Güneş, “Effect of feature-type in selecting distance measure for an artificial immune system as a pattern recognizer,” Digital Signal Processing, vol. 18, no. 4, pp. 635–645, 2008.
. H. Kahramanli and N. Allahverdi, “Design of a hybrid system for the diabetes and heart diseases,” Expert Systems with Applications, vol. 35, no. 1-2, pp. 82–89, 2008.
. G. Yan, G. Ma, J. Lv, and B. Song, “Combining independent component analysis with support vector machines,” in Proceedings of the in 1st International Symposium on Systems and Control in Aerospace and Astronautics (ISSCAA '06), pp. 493–496, Harbin, China, January 2006.
. S. Şahan, K. Polat, H. Kodaz, and S. Günes, “The medical applications of attribute weighted artificial immune system (AWAIS): diagnosis of heart and diabetes diseases,” Artificial Immune Systems, vol. 3627, pp. 456–468, 2005.
. W. Duch, R. Adamczak, and K. Grabczewski, “A new methodology of extraction, optimization and application of crisp and fuzzy logical rules,” IEEE Transactions on Neural Networks, vol. 12, no. 2, pp. 277–306, 2001.
. Abdar, M., Książek, W., Acharya, U. R., Tan, R. S., Makarenkov, V., & Pławiak, P. (2019). A new machine learning technique for an accurate diagnosis of coronary artery disease. Computer methods and programs in biomedicine, 179, 104992.
. Firdaus, F. F., Nugroho, H. A., & Soesanti, I. (2021, April). Deep Neural Network with Hyperparameter Tuning for Detection of Heart Disease. In 2021 IEEE Asia Pacific Conference on Wireless and Mobile (APWiMob) (pp. 59-65). IEEE.
. Qrenawi, M.I.; Al Sarraj, W.: Identification of cardiovascular diseases risk factors among diabetes patients using ontological data mining techniques. In: 2018 International Conference on Promising Electronic Technologies (ICPET), pp. 129–134 (2018).
. Awan, S.M.; Riaz, M.U.; Khan, A.G.: Prediction of heart disease using artificial neural network. VFAST Trans. Softw. Eng. 13, 102–112, 2018.
. More, K.; Raihan, M.; More, A.; Padule, S.; Mondal, S.: A12176 Smart phone based “heart attack” risk prediction; innovation of clinical and social approachforpreventivecardiachealth.J. Hypertens. 36, e321, 2018
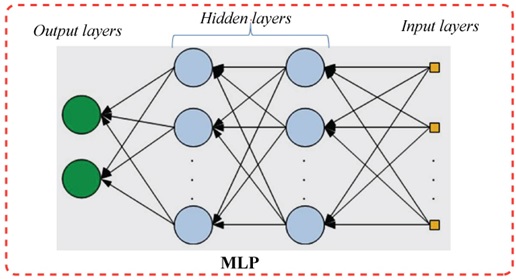
Published
How to Cite
Issue
Section
License
Copyright (c) 2022 50sea

This work is licensed under a Creative Commons Attribution 4.0 International License.