Melanoma Detection Using a Deep Learning Approach
Keywords:
Melanoma Identification, Melanoma detection, Deep Learning, VGG16, ResNet50, Data Augmentation.Abstract
Melanoma is a skin lesion disease; it is a skin cancer that is caused by uncontrolled growth in melanocytic tissues. Damaged cells can cause damage to nearby cells and consequently spreads cancer in other parts of the body. The aim of this research is the early detection of Melanoma disease, many researchers have already struggled and achieved success in detecting melanoma with different values for their evaluation parameters, they used different machine learning as well as deep learning approaches, and we applied deep learning approach for Melanoma detection, we used publicly available dataset for experimentation purpose. We applied deep learning algorithms ResNet50 and VGG16 for Melanoma detection; the accuracy, precision, recall, Jaccard index, and dice co-efficient of our proposed model are 92.3%, 93.3%, 90%, 9.98%, and 97.7%, respectively. Our proposed algorithm can be used to increase chances of survival for patients and can save the money which is used for diagnosis and treatment of Melanoma every year.
References
A. F. Jerant, J. T. Johnson, C. Sheridan, T. J. Caffrey et al., “Early detection and treatment of skin cancer,” American family physician, vol. 62, no. 2, pp. 357–386, 2000.
“ISIC 2018.” Available: https://challenge2018.isic-archive.com/.
Learning, Deep. "Deep learning." High-Dimensional Fuzzy Clustering (2020).
Hsu, Po-Ya, and Chester Holtz. "A comparison of machine learning tools for early prediction of sepsis from icu data." 2019 Computing in Cardiology (CinC). IEEE, 2019.
Setiawan, Agung W. "Image Segmentation Metrics in Skin Lesion: Accuracy, Sensitivity, Specificity, Dice Coefficient, Jaccard Index, and Matthews Correlation Coefficient." In 2020 International Conference on Computer Engineering, Network, and Intelligent Multimedia (CENIM), pp. 97-102. IEEE, 2020.
Kassani, Sara Hosseinzadeh, and Peyman Hosseinzadeh Kassani. "A comparative study of deep learning architectures on melanoma detection." Tissue and Cell 58 (2019): 76-83.
.Mendonça, T., Ferreira, P.M., Marques, J.S., Marcal, A.R., Rozeira, J.:PH2-adermoscopic image database for research and benchmarking. In: 35th International Conference of the IEEE Engineering in Medicine and Biology Society, Osaka, pp. 3–7. IEEE Press (2013).
https://doi.org/10.1109/EMBC.2013.661077.
.Gulati, Savy, and Rosepreet Kaur Bhogal. "Detection of malignant melanoma using deep learning." In International Conference on Advances in Computing and Data Sciences, pp. 312-325. Springer, Singapore, 2019.
Codella, Noel CF, Q-B. Nguyen, Sharath Pankanti, David A. Gutman, Brian Helba, Allan C. Halpern, and John R. Smith. "Deep learning ensembles for melanoma recognition in dermoscopy images." IBM Journal of Research and Development 61, no. 4/5 (2017): 5-1.
D. Gutman, N. Codella, E. Celebi, B. Helba, M. Marchetti, N. Mishra, A. Halpern. “Skin Lesion Analysis toward Melanoma Detection: A Challenge at the International Symposium on Biomedical Imaging (ISBI) 2016, hosted by the International Skin Imaging Collaboration (ISIC)”. eprint arXiv:1605.01397 [cs.CV]. 2016. Available: https://arxiv.org/abs/1605.01397.
Dascalu, Avi, and E. O. David. "Skin cancer detection by deep learning and sound analysis algorithms: A prospective clinical study of an elementary dermoscope." EBioMedicine 43 (2019): 107-113.
Frajka, Tamas, and Kenneth Zeger. "Downsampling dependent upsampling of images." Signal Processing: Image Communication 19, no. 3 (2004): 257-265.
Theckedath, Dhananjay, and R. R. Sedamkar. "Detecting affect states using VGG16, ResNet50 and SE-ResNet50 networks." SN Computer Science 1, no. 2 (2020): 1-7.
Lopez Adria Romero, Xavier Giro-i-Neto, Jack Burdick, and Oge Marques. “Skin lession classification using dermoscopic image using deep learning techniques.” 2017 13th LASTED international conference on biomedical engineering (BioMed), pp. 49-54. IEEE, 2017.
Yu, Chanki, Sejung Yang, Wonoh Kim, Jinwoong Jung, Kee-Yang Chung, Sang Wook Lee, and Byungho Oh. “Acral melanoma detection using a convolutional neural network for dermoscop images.” PloS one 13, no. 3 (2018): e0193321.
Chiem, A. Al-Jumaily, and R. N. Khushaba, A Novel Hybrid System for Skin Lesion Detection. Dec 2007.
Yacin Sikkandar, Mohamed, Bader Awadh Alrasheadi, N. B. Prakash, G. R. Hemalakshmi, A. Mohanarathinam, and K. Shankar. "Deep learning based an automated skin lesion segmentation and intelligent classification model." Journal of ambient intelligence and humanized computing 12, no. 3 (2021): 3245-3255.
Saeed, Jwan, and Subhi Zeebaree. "Skin lesion classification based on deep convolutional neural networks architectures." Journal of Applied Science and Technology Trends 2, no. 01 (2021): 41-51.
Kumar, S. Naresh, and B. Mohammed Ismail. "Systematic investigation on Multi-Class skin cancer categorization using machine learning approach." Materials Today: Proceedings (2020).
Huang, Hsin‐Wei, Benny Wei‐Yun Hsu, Chih‐Hung Lee, and Vincent S. Tseng. "Development of a light‐weight deep learning model for cloud applications and remote diagnosis of skin cancers." The Journal of Dermatology 48, no. 3 (2021): 310-316.
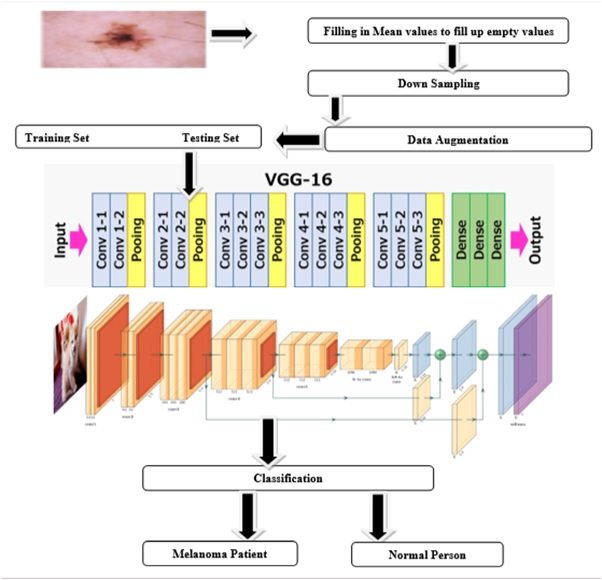
Published
How to Cite
Issue
Section
License
Copyright (c) 2022 50Sea

This work is licensed under a Creative Commons Attribution 4.0 International License.