Comparative Analysis of Urban Sprawl through KNN and Random Forest Classification (RFC) ML Techniques
Keywords:
Urban heat island, Land Fragmentation, Land Use, Land land fragmentationRevenue, Cultivated Land, Land Surface Temperature, Normalized Difference vegetation Index (NDVI)Abstract
The majority of optimization strategies fail to take into account the dynamic impact of urban sprawl on the spatial criteria that underlie decision-making processes. Furthermore, the integration of the existing simulation methodology with land use optimization techniques to arrive at a sustainable judgment regarding the appropriate site involves intricate procedures. The urban heat island phenomenon is a prominent consequence of urban expansion and human activities, leading to elevated temperatures within cities compared to their rural surroundings. The extent of sprawl was estimated through ML algorithms and it was revealed that RFC provided promising results that were near to statistics by various administrative authorities. Urban vegetation plays a crucial role in countering the urban heating effect by providing cooling mechanisms through evaporation and shading. In this context, a study was conducted in Allama Iqbal Town, Lahore, focusing on the assessment of land use changes, as well as the analysis of Normalized Difference Vegetation Index and Land Surface Temperature data for the years 2000, 2010, and 2023, obtained from Landsat 5 and Landsat 8 satellite imagery. The findings reveal significant land use changes of 7.52% (36.2 km2) in the study area. The built-up areas expanded by 50.76%, while smart green spaces decreased by 48.30%. The relationships between NDVI and LST demonstrate a robust negative relationship (R² = 0.99). This research underscores the potential of utilizing GIS and remote sensing techniques to inform urban planning, decision-making, and policy formulation, ultimately contributing to the creation of sustainable urban environments in Allama Iqbal Town.
References
T. Susca, S. R. Gaffin, and G. R. Dell’Osso, “Positive effects of vegetation: Urban heat island and green roofs,” Environ. Pollut., vol. 159, no. 8–9, pp. 2119–2126, Aug. 2011, doi: 10.1016/J.ENVPOL.2011.03.007.
T. H. Son, Z. Weedon, T. Yigitcanlar, T. Sanchez, J. M. Corchado, and R. Mehmood, “Algorithmic urban planning for smart and sustainable development: Systematic review of the literature,” Sustain. Cities Soc., vol. 94, Jul. 2023, doi: 10.1016/J.SCS.2023.104562.
T. Yigitcanlar, D. Agdas, and K. Degirmenci, “Artificial intelligence in local governments: perceptions of city managers on prospects, constraints and choices,” AI Soc., vol. 38, no. 3, pp. 1135–1150, Jun. 2023, doi: 10.1007/S00146-022-01450-X.
K. C. Seto, M. Fragkias, B. Güneralp, and M. K. Reilly, “A meta-analysis of global urban land expansion,” PLoS One, vol. 6, no. 8, 2011, doi: 10.1371/JOURNAL.PONE.0023777.
Z. Ren et al., “Estimation of the Relationship between Urban Park Characteristics and Park Cool Island Intensity by Remote Sensing Data and Field Measurement,” For. 2013, Vol. 4, Pages 868-886, vol. 4, no. 4, pp. 868–886, Oct. 2013, doi: 10.3390/F4040868.
A. Aflaki et al., “Urban heat island mitigation strategies: A state-of-the-art review on Kuala Lumpur, Singapore, and Hong Kong,” Cities, vol. 62, pp. 131–145, Feb. 2017, doi: 10.1016/J.CITIES.2016.09.003.
D. Triantakonstantis, G. Mountrakis, D. Triantakonstantis, and G. Mountrakis, “Urban Growth Prediction: A Review of Computational Models and Human Perceptions,” J. Geogr. Inf. Syst., vol. 4, no. 6, pp. 555–587, Dec. 2012, doi: 10.4236/JGIS.2012.46060.
D. Gupta, D. Sethi, and R. Bathija, “A Review on Land-use and Land-change with Machine Learning Algorithm,” IOP Conf. Ser. Mater. Sci. Eng., vol. 1119, no. 1, p. 012006, Mar. 2021, doi: 10.1088/1757-899X/1119/1/012006.
E. J. Gago, J. Roldan, R. Pacheco-Torres, and J. Ordóñez, “The city and urban heat islands: A review of strategies to mitigate adverse effects,” Renew. Sustain. Energy Rev., vol. 25, pp. 749–758, Sep. 2013, doi: 10.1016/J.RSER.2013.05.057.
V. Chettry, “Geospatial measurement of urban sprawl using multi-temporal datasets from 1991 to 2021: case studies of four Indian medium-sized cities,” Environ. Monit. Assess., vol. 194, no. 12, Dec. 2022, doi: 10.1007/S10661-022-10542-6.
D. E. Bowler, L. Buyung-Ali, T. M. Knight, and A. S. Pullin, “Urban greening to cool towns and cities: A systematic review of the empirical evidence,” Landsc. Urban Plan., vol. 97, no. 3, pp. 147–155, Sep. 2010, doi: 10.1016/J.LANDURBPLAN.2010.05.006.
S. Oliveira, H. Andrade, and T. Vaz, “The cooling effect of green spaces as a contribution to the mitigation of urban heat: A case study in Lisbon,” Build. Environ., vol. 46, no. 11, pp. 2186–2194, Nov. 2011, doi: 10.1016/J.BUILDENV.2011.04.034.
A. G. Fontana, V. F. Nascimento, J. P. Ometto, and F. H. F. do Amaral, “Analysis of past and future urban growth on a regional scale using remote sensing and machine learning,” Front. Remote Sens., vol. 4, no. September, p. 1123254, Sep. 2023, doi: 10.3389/FRSEN.2023.1123254.
C. W. Mackey, X. Lee, and R. B. Smith, “Remotely sensing the cooling effects of city scale efforts to reduce urban heat island,” Build. Environ., vol. 49, no. 1, pp. 348–358, Mar. 2012, doi: 10.1016/J.BUILDENV.2011.08.004.
J. M. Kim, J. S. Park, C. Y. Lee, and S. G. Lee, “PREDICTING OF URBAN EXPANSION USING CONVOLUTIONAL LSTM NETWORK MODEL: THE CASE OF SEOUL METROPOLITAN AREA, KOREA”, doi: 10.5194/isprs-annals-X-4-W3-2022-113-2022.
A. Rienow and A. Rienow, “The Future of Central European Cities – Optimization of a Cellular Automaton for the Spatially Explicit Prediction of Urban Sprawl,” Optim. Algorithms - Methods Appl., Sep. 2016, doi: 10.5772/62424.
M. Jabbar, M. M. Yusoff, and A. Shafie, “Assessing the role of urban green spaces for human well-being: a systematic review,” GeoJournal, vol. 87, no. 5, pp. 4405–4423, Oct. 2022, doi: 10.1007/S10708-021-10474-7/FIGURES/2.
S. D. Nagappan and S. M. Daud, “Machine Learning Predictors for Sustainable Urban Planning,” IJACSA) Int. J. Adv. Comput. Sci. Appl., vol. 12, no. 7, p. 2021, Accessed: Sep. 27, 2023. [Online]. Available: www.ijacsa.thesai.org
K. Kulkarni and P. A. Vijaya, “Measuring urban sprawl using machine learning,” Fundam. Methods Mach. Deep Learn. Algorithms, Tools, Appl., pp. 327–340, Jan. 2022, doi: 10.1002/9781119821908.ch14.
Jabbar and M. M. Yusoff, “Assessing The Spatiotemporal Urban Green Cover Changes and Their Impact on Land Surface Temperature and Urban Heat Island in Lahore (Pakistan),” Geogr. Environ. Sustain., vol. 15, no. 1, pp. 130–140, Mar. 2022, doi: 10.24057/2071-9388-2021-005.
P. Ponukumati, M. Azharuddin, S. K. Regonda, P. Ponukumati, M. Azharuddin, and S. K. Regonda, “Estimation of Urban Sprawl Using Machine Learning Methods for the city Hyderabad, India,” AGUFM, vol. 2020, pp. H094-05, 2020, Accessed: Sep. 27, 2023. [Online]. Available: https://ui.adsabs.harvard.edu/abs/2020AGUFMH094...05P/abstract
S. C. Koumetio Tekouabou, E. B. Diop, R. Azmi, R. Jaligot, and J. Chenal, “Reviewing the application of machine learning methods to model urban form indicators in planning decision support systems: Potential, issues and challenges,” J. King Saud Univ. - Comput. Inf. Sci., vol. 34, no. 8, pp. 5943–5967, Sep. 2022, doi: 10.1016/J.JKSUCI.2021.08.007.
A. Khan and M. Sudheer, “Machine learning-based monitoring and modeling for spatio-temporal urban growth of Islamabad,” Egypt. J. Remote Sens. Sp. Sci., vol. 25, no. 2, pp. 541–550, Aug. 2022, doi: 10.1016/J.EJRS.2022.03.012.
M. A. Siddique et al., “Assessment and simulation of land use and land cover change impacts on the land surface temperature of Chaoyang District in Beijing, China,” PeerJ, vol. 2020, no. 3, p. e9115, May 2020, doi: 10.7717/PEERJ.9115/SUPP-3.
B. Stone et al., “Avoided Heat-Related Mortality through Climate Adaptation Strategies in Three US Cities,” PLoS One, vol. 9, no. 6, p. e100852, Jun. 2014, doi: 10.1371/JOURNAL.PONE.0100852.
K. R. Gunawardena, M. J. Wells, and T. Kershaw, “Utilising green and blue space to mitigate urban heat island intensity,” Sci. Total Environ., vol. 584–585, pp. 1040–1055, Apr. 2017, doi: 10.1016/J.SCITOTENV.2017.01.158.
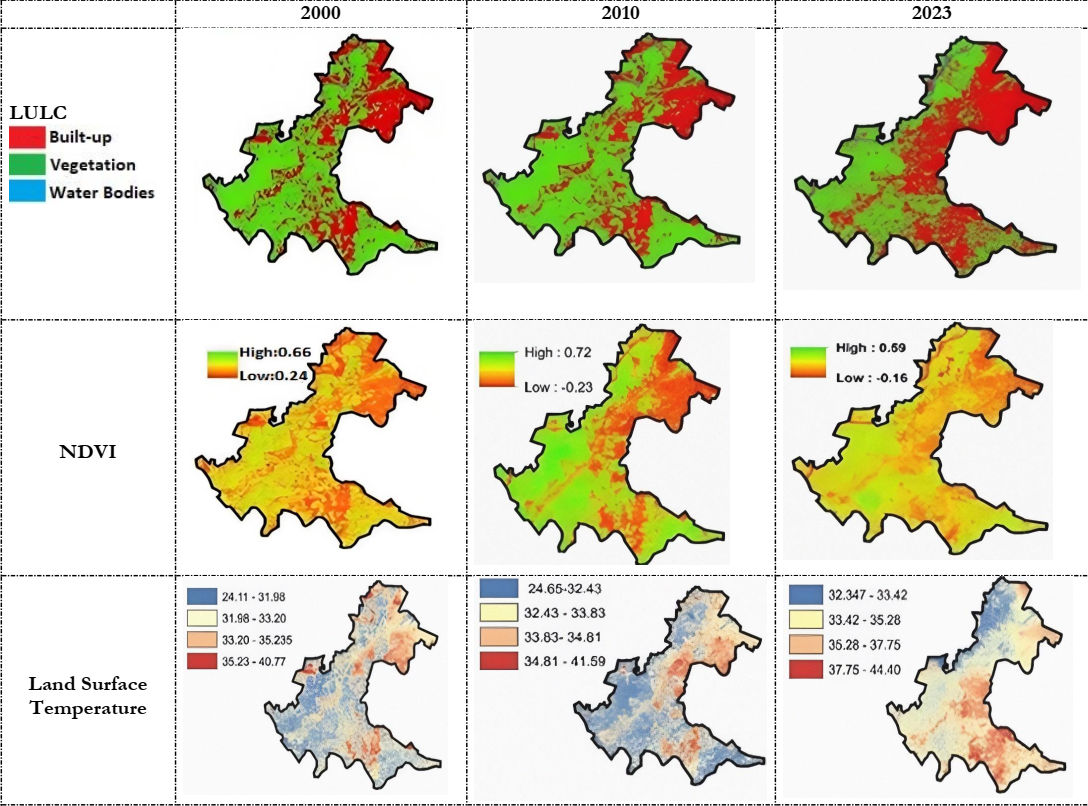
Published
How to Cite
Issue
Section
License
Copyright (c) 2023 50SEA

This work is licensed under a Creative Commons Attribution 4.0 International License.