Deep Learning Based Identification and Categorization of Various Phases of Diabetic Retinopathy
Keywords:
DenseNet121 Model, Diabetes, Diabetic Retinopathy, Kaggle Dataset, RetinaNetAbstract
Diabetic Retinopathy is a growing disease that affects the human retina of diabetic patients and if it is left untreated it leads to loss of vision. Early diagnosis and accurate classification of DR stages are important for immediate intervention and efficient control. Therefore, this study focuses on the classification of different stages of diabetic retinopathy in retinal images by using a DL (deep learning) model named Densenet121. The dataset used in this research contains various collections of color fundus images obtained from diabetic patients, labelled with corresponding disease stages. The dataset used was taken from Kaggle named APTOS 2019. Standard metrics such as accuracy, recall, F1-score, and precision are used to measure the effectiveness of the proposed model. The proposed DL based classification model shows encouraging results and has achieved a high level of accuracy across various severity levels. This model offers an automated method for detection and classification of the disease facilitating early diagnosis. Overall, this study advances automated diagnosis to lessen the burden of diabetic retinopathy.
References
G. Roglic, “WHO Global report on diabetes: A summary,” Int. J. Noncommunicable Dis., vol. 1, no. 1, p. 3, 2016, doi: 10.4103/2468-8827.184853.
Z. L. Teo et al., “Global Prevalence of Diabetic Retinopathy and Projection of Burden through 2045: Systematic Review and Meta-analysis,” Ophthalmology, vol. 128, no. 11, pp. 1580–1591, Nov. 2021, doi: 10.1016/J.OPHTHA.2021.04.027.
M. Tsighe, H. M. T. Student, and S. Kant, “Transfer Learning based Detection of Diabetic Retinopathy from Small Dataset,” May 2019, Accessed: Jun. 16, 2024. [Online]. Available: https://arxiv.org/abs/1905.07203v2
S. Qummar et al., “A Deep Learning Ensemble Approach for Diabetic Retinopathy Detection,” IEEE Access, vol. 7, pp. 150530–150539, 2019, doi: 10.1109/ACCESS.2019.2947484.
B. Tymchenko, P. Marchenko, and D. Spodarets, “Deep Learning Approach to Diabetic Retinopathy Detection,” Int. Conf. Pattern Recognit. Appl. Methods, vol. 1, pp. 501–509, 2020, doi: 10.5220/0008970805010509.
A. Jabbar et al., “A Lesion-Based Diabetic Retinopathy Detection Through Hybrid Deep Learning Model,” IEEE Access, vol. 12, pp. 40019–40036, 2024, doi: 10.1109/ACCESS.2024.3373467.
Ş. Y. Atcı, A. Güneş, M. Zontul, and Z. Arslan, “Identifying Diabetic Retinopathy in the Human Eye: A Hybrid Approach Based on a Computer-Aided Diagnosis System Combined with Deep Learning,” Tomogr. 2024, Vol. 10, Pages 215-230, vol. 10, no. 2, pp. 215–230, Feb. 2024, doi: 10.3390/TOMOGRAPHY10020017.
B. Lalithadevi and S. Krishnaveni, “Diabetic retinopathy detection and severity classification using optimized deep learning with explainable AI technique,” Multimed. Tools Appl., pp. 1–65, Apr. 2024, doi: 10.1007/S11042-024-18863-Z/METRICS.
C. Mohanty et al., “Using Deep Learning Architectures for Detection and Classification of Diabetic Retinopathy,” Sensors 2023, Vol. 23, Page 5726, vol. 23, no. 12, p. 5726, Jun. 2023, doi: 10.3390/S23125726.
W. K. Wong, F. H. Juwono, and C. Apriono, “Diabetic Retinopathy Detection and Grading: A Transfer Learning Approach Using Simultaneous Parameter Optimization and Feature-Weighted ECOC Ensemble,” IEEE Access, vol. 11, pp. 83004–83016, 2023, doi: 10.1109/ACCESS.2023.3301618.
E. V. Carrera, A. Gonzalez, and R. Carrera, “Automated detection of diabetic retinopathy using SVM,” Proc. 2017 IEEE 24th Int. Congr. Electron. Electr. Eng. Comput. INTERCON 2017, Oct. 2017, doi: 10.1109/INTERCON.2017.8079692.
Q. H. Nguyen et al., “Diabetic retinopathy detection using deep learning,” ACM Int. Conf. Proceeding Ser., pp. 103–107, Jan. 2020, doi: 10.1145/3380688.3380709.
S. Albahli and G. N. A. H. Yar, “Detection of Diabetic Retinopathy Using Custom CNN to Segment the Lesions,” Intell. Autom. Soft Comput., vol. 33, no. 2, pp. 837–853, Feb. 2022, doi: 10.32604/IASC.2022.024427.
“Classification of Diabetic Retinopathy Images by Using Deep Learning Models.” Accessed: Jun. 16, 2024. [Online]. Available: http://article.nadiapub.com/IJGDC/vol11_no1/9.pdf
Z. Khan et al., “Diabetic Retinopathy Detection Using VGG-NIN a Deep Learning Architecture,” IEEE Access, vol. 9, pp. 61408–61416, 2021, doi: 10.1109/ACCESS.2021.3074422.
D. J. Hemanth, O. Deperlioglu, and U. Kose, “An enhanced diabetic retinopathy detection and classification approach using deep convolutional neural network,” Neural Comput. Appl., vol. 32, no. 3, pp. 707–721, Feb. 2020, doi: 10.1007/S00521-018-03974-0/METRICS.
S. Keel et al., “Development and validation of a deep-learning algorithm for the detection of neovascular age-related macular degeneration from colour fundus photographs,” Clin. Experiment. Ophthalmol., vol. 47, no. 8, pp. 1009–1018, Nov. 2019, doi: 10.1111/CEO.13575.
A. K. Gangwar and V. Ravi, “Diabetic Retinopathy Detection Using Transfer Learning and Deep Learning,” Adv. Intell. Syst. Comput., vol. 1176, pp. 679–689, 2021, doi: 10.1007/978-981-15-5788-0_64.
L. Qiao, Y. Zhu, and H. Zhou, “Diabetic Retinopathy Detection Using Prognosis of Microaneurysm and Early Diagnosis System for Non-Proliferative Diabetic Retinopathy Based on Deep Learning Algorithms,” IEEE Access, vol. 8, pp. 104292–104302, 2020, doi: 10.1109/ACCESS.2020.2993937.
Pedregosa, F.; Varoquaux, G.; Gramfort, A.; Michel, V.; Thirion, B.; Grisel, O.; Blondel, M.; Prettenhofer, P.; Weiss, R.; Dubourg, V. & others, "Scikit-learn: Machine learning in Python", Journal of Machine Learning Research Vol. 12, pp. 2825--2830, 2011, https://doi.org/10.48550/arXiv.1201.0490
Abadi, M. et al. “TensorFlow: Large-Scale Machine Learning on Heterogeneous Distributed Systems.” ArXiv abs/1603.04467 (2016).
Diederik Kingma and Jimmy Ba. 2015. Adam: A Method for Stochastic Optimization. https://doi.org/10.48550/arXiv.1412.6980
G. Kurup, J. A. A. Jothi, and A. Kanadath, “Diabetic Retinopathy Detection and Classification using Pretrained Inception-v3,” 2021 Int. Conf. Smart Gener. Comput. Commun. Networking, SMART GENCON 2021, 2021, doi: 10.1109/SMARTGENCON51891.2021.9645914.
R. Yasashvini, V. Raja Sarobin M, R. Panjanathan, S. Graceline Jasmine, and L. Jani Anbarasi, “Diabetic Retinopathy Classification Using CNN and Hybrid Deep Convolutional Neural Networks,” Symmetry 2022, Vol. 14, Page 1932, vol. 14, no. 9, p. 1932, Sep. 2022, doi: 10.3390/SYM14091932.
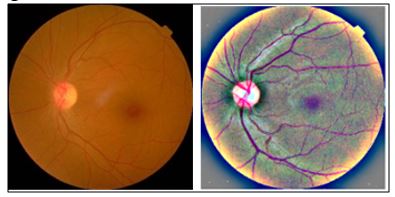
Downloads
Published
How to Cite
Issue
Section
License
Copyright (c) 2024 50SEA

This work is licensed under a Creative Commons Attribution 4.0 International License.