Leveraging CIELAB Segmentation and CNN for Wheat Fungi Disease Classification
Keywords:
CNN, Wheat Fungi Diseases, Deep Learning, CIELAB Segmentation and Smart FarmingAbstract
Wheat is the third most harvested and consumed grain globally, but a significant portion of its production is wasted due to diseases. Fungal infections caused by pathogenic fungi are particularly harmful, greatly reducing crop yields. Manual visual inspection of large fields is slow, exhausting, and requires specialized expertise. This research introduces a novel combination of image augmentation, CIELAB segmentation, and a fine-tuned pre-trained CNN, achieving an unprecedented 98.43% accuracy in wheat fungal disease classification, addressing gaps in current detection methods and promoting sustainable agriculture. To conduct this research, datasets from Kaggle were merged and meticulously validated to create a comprehensive set with five classes: healthy wheat and four fungal diseases. Preprocessing steps included resizing, contrast enhancement and noise removal to ensure uniform and high-quality images followed by rigorous image augmentation techniques to expand and diversify the dataset ultimately enhancing the deep learning model's robustness and accuracy. The CNN model, trained over 80 epochs achieved an impressive 98.43% accuracy in classifying wheat fungal diseases. With a precision of 98.47% and an F1 score of 98.43% the model demonstrated strong positive classification accuracy. Additionally, a recall of 98.43% and specificity of 98.47% indicated its effectiveness in identifying true positive cases and accurately detecting disease presence or absence.
References
Genaev, M.A., et al., Image-based wheat fungi diseases identification by deep learning. 2021. 10(8): p. 1500.
Joseph, D.S., P.M. Pawar, and K.J.I.A. Chakradeo, Real-time Plant Disease Dataset Development and Detection of Plant Disease Using Deep Learning. 2024.
Kolmer, J. and O.J.W.N. Fajolu, Wheat leaf rust in the United States in 2020. 2021. 67: p. 78-87.
Khanfri, S., M. Boulif, and R.J.N.S.B. Lahlali, Yellow rust (Puccinia striiformis): a serious threat to wheat production worldwide. 2018. 10(3): p. 410-423.
Figueroa, M., K.E. Hammond‐Kosack, and P.S.J.M.p.p. Solomon, A review of wheat diseases—a field perspective. 2018. 19(6): p. 1523-1536.
Bhavani, S., et al., Wheat rusts: current status, prospects of genetic control and integrated approaches to enhance resistance durability, in Wheat improvement: Food Security in a changing climate. 2022, Springer International Publishing Cham. p. 125-141.
Sendhil, R., et al., Wheat in Asia: trends, challenges and research priorities, in New horizons in wheat and barley research: global trends, breeding and quality enhancement. 2022, Springer. p. 33-61.
Iqbal, A., et al., Molecular and field-based characterization of yellow rust resistance in exotic wheat germplasm. 2020. 57(6).
Bhavani, S., et al., Globally important wheat diseases: status, challenges, breeding and genomic tools to enhance resistance durability. 2021: p. 59-128.
Savary, S. and L.J.A.r.o.p. Willocquet, Modeling the impact of crop diseases on global food security. 2020. 58(1): p. 313-341.
Kouadio, L., et al., A review on UAV-based applications for plant disease detection and monitoring. 2023. 15(17): p. 4273.
Kumar, D., V.J.M.T. Kukreja, and Applications, Deep learning in wheat diseases classification: A systematic review. 2022. 81(7): p. 10143-10187.
Muhammadnur, J., SEVERITY CLASSIFICATION OF YELLOW RUST DISEASE AND TREATMENT RECOMMENDATION FROM WHEAT IMAGES USING DEEP LEARNING TECHNIQUES. 2024, Haramaya University.
Lei, T., et al., Simulation of automatically annotated visible and multi/hyperspectral images using the Helios 3D plant and radiative transfer modeling framework.
Bhola, A., P.J.M.T. Kumar, and Applications, Deep feature-support vector machine based hybrid model for multi-crop leaf disease identification in Corn, Rice, and Wheat. 2024: p. 1-21.
Long, M., et al., Classification of wheat diseases using deep learning networks with field and glasshouse images. 2023. 72(3): p. 536-547.
Aboneh, T., et al., Computer vision framework for wheat disease identification and classification using Jetson GPU infrastructure. 2021. 9(3): p. 47.
Haider, W., et al., A generic approach for wheat disease classification and verification using expert opinion for knowledge-based decisions. 2021. 9: p. 31104-31129.
Khatri, A., S. Agrawal, and J.M.J.S.p. Chatterjee, Wheat seed classification: utilizing ensemble machine learning approach. 2022. 2022.
Mumtaz, R., et al., Integrated digital image processing techniques and deep learning approaches for wheat stripe rust disease detection and grading. 2023. 8: p. 100305.
Sheenam, S., S. Khattar, and T. Verma. Automated Wheat Plant Disease Detection using Deep Learning: A Multi-Class Classification Approach. in 2023 3rd International Conference on Intelligent Technologies (CONIT). 2023. IEEE.
Cheng, S., et al., A high performance wheat disease detection based on position information. 2023. 12(5): p. 1191.
Liu, Q., et al., Development of Machine Learning Methods for Accurate Prediction of Plant Disease Resistance. 2024.
Borowik, P., et al., Analysis of Wheat Grain Infection by Fusarium Mycotoxin-Producing Fungi Using an Electronic Nose, GC-MS, and qPCR. 2024. 24(2): p. 326.
Wheat Disease Detection. Available from: kaggle.com/datasets/sinadunk23/behzad-safari-jalal.
Wheat Leaf dataset. August 01, 2021 Available from: kaggle.com/datasets/olyadgetch/wheat-leaf-dataset.
Xu, M., et al., A comprehensive survey of image augmentation techniques for deep learning. 2023. 137: p. 109347.
Kaur, A. Comparison between YCbCr Color Space and CIELab Color Space for Skin Color Segmentation. 2012.
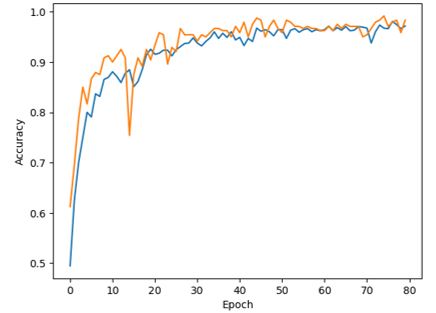
Downloads
Published
How to Cite
Issue
Section
License
Copyright (c) 2024 50SEA

This work is licensed under a Creative Commons Attribution 4.0 International License.