Comparative Assessment of Classification Algorithms for Land Cover Mapping Using Multispectral and PCA Images of Landsat
Keywords:
MLC, SVM, RF, PCA, Normalized indices, Landsat-8, ESA, LULCAbstract
The advancement of remote sensing technologies and the availability of free satellite data have significantly enhanced the precision of land use and land cover (LULC) mapping, facilitating the analysis of landscape transformations and ecosystem changes. However, selecting the most suitable classifier for LULC mapping remains a complex challenge. Therefore, it is essential to evaluate the accuracy of various LULC modeling algorithms to determine their effectiveness in different applications. This study conducted a comprehensive evaluation of both supervised machine learning algorithms and traditional classification methods applied to Landsat 8 imagery with a 30-meter spatial resolution, covering the Shangla and Battagram districts in Khyber Pakhtunkhwa (KPK), Pakistan. The study focused on three classification algorithms: Maximum Likelihood Classification (MLC), Support Vector Machines (SVM), and Random Forest (RF). The performance of these algorithms was assessed on both multispectral images and composite images derived from Principal Component Analysis (PCA) and Band Ratioing and/or Normalized Indices. Additionally, the accuracy of these algorithms, when applied to different datasets, was compared with the recently released World Cover LULC product by the European Space Agency (ESA). The results indicated that the SVM algorithm outperformed the others, achieving an overall accuracy of 90.43% and a kappa coefficient of 0.8792. The MLC and RF algorithms also produced promising results, with overall accuracies of 85.58% and 88.46%, respectively. Furthermore, the study found that the overall accuracy of ESA’s World Cover LULC product was 70.67% in the study area, based on similar validation samples. These findings underscore the strengths and limitations of each algorithm, providing valuable insights into their suitability for LULC classification and the applicability of existing global LULC maps.
References
A. Jamali, “Evaluation and comparison of eight machine learning models in land use/land cover mapping using Landsat 8 OLI: a case study of the northern region of Iran,” SN Appl Sci, vol. 1, no. 11, Nov. 2019, doi: 10.1007/s42452-019-1527-8.
S. Aldiansyah and R. A. Saputra, “COMPARISON OF MACHINE LEARNING ALGORITHMS FOR LAND USE AND LAND COVER ANALYSIS USING GOOGLE EARTH ENGINE (CASE STUDY: WANGGU WATERSHED).”
S. Bedi, A. Samal, C. Ray, and D. Snow, “Comparative evaluation of machine learning models for groundwater quality assessment,” Environ Monit Assess, vol. 192, no. 12, Dec. 2020, doi: 10.1007/s10661-020-08695-3.
L. Ghayour et al., “Performance evaluation of sentinel-2 and landsat 8 OLI data for land cover/use classification using a comparison between machine learning algorithms,” Remote Sens (Basel), vol. 13, no. 7, Apr. 2021, doi: 10.3390/rs13071349.
Z. Zhao et al., “Comparison of Three Machine Learning Algorithms Using Google Earth Engine for Land Use Land Cover Classification,” Rangel Ecol Manag, vol. 92, pp. 129–137, Jan. 2024, doi: 10.1016/j.rama.2023.10.007.
V. Sheth, U. Tripathi, and A. Sharma, “A Comparative Analysis of Machine Learning Algorithms for Classification Purpose,” in Procedia Computer Science, Elsevier B.V., 2022, pp. 422–431. doi: 10.1016/j.procs.2022.12.044.
M. Z. Hasan, R. S. Leya, and K. S. Islam, “COMPARATIVE ASSESSMENT OF MACHINE LEARNING ALGORITHMS FOR LAND USE AND LAND COVER CLASSIFICATION USING MULTISPECTRAL REMOTE SENSING IMAGE,” Khulna University Studies, pp. 33–46, Oct. 2022, doi: 10.53808/kus.2022.icstem4ir.0124-se.
Z. Zafar, M. Zubair, Y. Zha, S. Fahd, and A. Ahmad Nadeem, “Performance assessment of machine learning algorithms for mapping of land use/land cover using remote sensing data,” Egyptian Journal of Remote Sensing and Space Science, vol. 27, no. 2, pp. 216–226, Jun. 2024, doi: 10.1016/j.ejrs.2024.03.003.
F. F. Camargo, E. E. Sano, C. M. Almeida, J. C. Mura, and T. Almeida, “A comparative assessment of machine-learning techniques for land use and land cover classification of the Brazilian tropical savanna using ALOS-2/PALSAR-2 polarimetric images,” Remote Sens (Basel), vol. 11, no. 13, Jul. 2019, doi: 10.3390/rs11131600.
R. Eastman, “Guide to GIS and Image Processing Volume 2.” [Online]. Available: https://www.researchgate.net/publication/242377547
M. Mohajane et al., “Land use/land cover (LULC) using landsat data series (MSS, TM, ETM+ and OLI) in azrou forest, in the central middle atlas of Morocco,” Environments - MDPI, vol. 5, no. 12, pp. 1–16, Dec. 2018, doi: 10.3390/environments5120131.
S. Talukdar, P. Singha, Shahfahad, S. Mahato, B. Praveen, and A. Rahman, “Dynamics of ecosystem services (ESs) in response to land use land cover (LU/LC) changes in the lower Gangetic plain of India,” Ecol Indic, vol. 112, May 2020, doi: 10.1016/j.ecolind.2020.106121.
B. Rimal, S. Rijal, and R. Kunwar, “Comparing Support Vector Machines and Maximum Likelihood Classifiers for Mapping of Urbanization,” Journal of the Indian Society of Remote Sensing, vol. 48, no. 1, pp. 71–79, Jan. 2020, doi: 10.1007/s12524-019-01056-9.
V. K. A. Somayajula, D. Ghai, and S. Kumar, “Land Use/Land Cover Change Analysis using NDVI, PCA,” in Proceedings - 5th International Conference on Computing Methodologies and Communication, ICCMC 2021, Institute of Electrical and Electronics Engineers Inc., Apr. 2021, pp. 849–855. doi: 10.1109/ICCMC51019.2021.9418025.
T. Li, S. Li, C. Liang, R. T. Bush, L. Xiong, and Y. Jiang, “A comparative assessment of Australia’s Lower Lakes water quality under extreme drought and post-drought conditions using multivariate statistical techniques,” J Clean Prod, vol. 190, pp. 1–11, Jul. 2018, doi: 10.1016/j.jclepro.2018.04.121.
M. Dharani and G. Sreenivasulu, “Land use and land cover change detection by using principal component analysis and morphological operations in remote sensing applications,” International Journal of Computers and Applications, vol. 43, no. 5, pp. 462–471, 2021, doi: 10.1080/1206212X.2019.1578068.
S. Basheer et al., “Comparison of Land Use Land Cover Classifiers Using Different Satellite Imagery and Machine Learning Techniques,” Remote Sens (Basel), vol. 14, no. 19, Oct. 2022, doi: 10.3390/rs14194978.
M. Pal and P. M. Mather, “Support vector machines for classification in remote sensing,” Int J Remote Sens, vol. 26, no. 5, pp. 1007–1011, Mar. 2005, doi: 10.1080/01431160512331314083.
A. Mathur and G. M. Foody, “Multiclass and binary SVM classification: Implications for training and classification users,” IEEE Geoscience and Remote Sensing Letters, vol. 5, no. 2, pp. 241–245, Apr. 2008, doi: 10.1109/LGRS.2008.915597.
S. Talukdar, P. Singha, Shahfahad, S. Mahato, B. Praveen, and A. Rahman, “Dynamics of ecosystem services (ESs) in response to land use land cover (LU/LC) changes in the lower Gangetic plain of India,” Ecol Indic, vol. 112, May 2020, doi: 10.1016/j.ecolind.2020.106121.
L. Breiman, “RANDOM FORESTS-RANDOM FEATURES,” 1999.
B. R. Shivakumar and S. V. Rajashekararadhya, “Investigation on land cover mapping capability of maximum likelihood classifier: A case study on North Canara, India,” in Procedia Computer Science, Elsevier B.V., 2018, pp. 579–586. doi: 10.1016/j.procs.2018.10.434.
P. V Bolstad and T. M. Lillesand, “Rapid Maximum Likelihood Classification,” 1991.
F. Ghasempour, A. Sekertekin, and S. H. Kutoglu, “HOW LANDSAT 9 IS SUPERIOR TO LANDSAT 8: COMPARATIVE ASSESSMENT OF LAND USE LAND COVER CLASSIFICATION AND LAND SURFACE TEMPERATURE,” in ISPRS Annals of the Photogrammetry, Remote Sensing and Spatial Information Sciences, Copernicus Publications, Jan. 2023, pp. 221–227. doi: 10.5194/isprs-annals-X-4-W1-2022-221-2023.
Y. O. Ouma, A. Keitsile, B. Nkwae, P. Odirile, D. Moalafhi, and J. Qi, “Urban land-use classification using machine learning classifiers: comparative evaluation and post-classification multi-feature fusion approach,” Eur J Remote Sens, vol. 56, no. 1, 2023, doi: 10.1080/22797254.2023.2173659.
L. Khaldi, A. Elabed, and A. El Khanchoufi, “Performance evaluation of Machine Learning algorithms for LULC classification: A case study of Fez-Meknes region,” E3S Web of Conferences, vol. 527, p. 02012, May 2024, doi: 10.1051/e3sconf/202452702012.
M. Ganjirad and H. Bagheri, “Google Earth Engine-based mapping of land use and land cover for weather forecast models using Landsat 8 imagery,” Ecol Inform, vol. 80, May 2024, doi: 10.1016/j.ecoinf.2024.102498.
M. Safabakhshpachehkenari and H. Tonooka, “Assessing and Enhancing Predictive Efficacy of Machine Learning Models in Urban Land Dynamics: A Comparative Study Using Multi-Resolution Satellite Data,” Remote Sens (Basel), vol. 15, no. 18, Sep. 2023, doi: 10.3390/rs15184495.
L. Breiman, “Random Forests,” 2001.
C. Pelletier, S. Valero, J. Inglada, N. Champion, C. M. Sicre, and G. Dedieu, “Effect of training class label noise on classification performances for land cover mapping with satellite image time series,” Remote Sens (Basel), vol. 9, no. 2, 2017, doi: 10.3390/rs9020173.
A. M. Abdi, “Land cover and land use classification performance of machine learning algorithms in a boreal landscape using Sentinel-2 data,” GIsci Remote Sens, vol. 57, no. 1, pp. 1–20, Jan. 2020, doi: 10.1080/15481603.2019.1650447.
A. Tariq et al., “Modelling, mapping and monitoring of forest cover changes, using support vector machine, kernel logistic regression and naive bayes tree models with optical remote sensing data,” Heliyon, vol. 9, no. 2, Feb. 2023, doi: 10.1016/j.heliyon.2023.e13212.
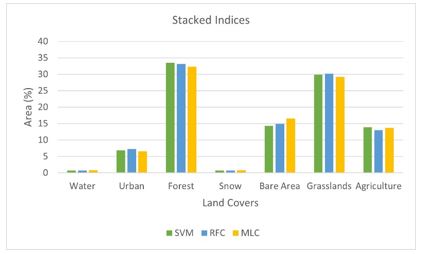
Downloads
Published
How to Cite
Issue
Section
License
Copyright (c) 2024 50SEA

This work is licensed under a Creative Commons Attribution 4.0 International License.