Efficiency Assessment for Crop Classification Using Multi-Sensor Data in Google Earth Engine
Keywords:
Machine learning, Crop classification, Sentinel-1, Sentinel-2, Google Earth EngineAbstract
Accurate mapping of agricultural lands and crop distribution is crucial for food security, sustainable development, and informed policymaking. This research classified agricultural crops in the Rahim Yar Khan district of Pakistan using multi-sensor images from Sentinel-1 and Sentinel-2 satellites. The study employed the cloud computing platform Google Earth Engine (GEE) and compared the performance of the Random Forest (RF) algorithm using Sentinel-1 (VV, HV, and HV+VV), Sentinel-2, and integrated datasets. Ground truth information obtained from field surveys and high-resolution images served as reference samples for training and validation. The fusion of Sentinel-1 and Sentinel-2 data enhanced feature extraction, leading to improved crop type classification. Post-processing procedures ensured that the maps were visually clear and free of noise, allowing for accurate crop mapping and land cover categorization. The classification results indicated high accuracy for crops such as sugarcane, cotton, rice, and water bodies. The RF classifier using fused data achieved the highest accuracy (overall accuracy of 93% and Kappa coefficient of 90%), followed by Sentinel-2 (89%), Sentinel-1 VV+VH (72%), Sentinel-1 VH (66%), and Sentinel-1 VV (62%). The study underscores the value of data integration in improving the classification accuracy of major crops (sugarcane, cotton, and rice) in the region. While some classes showed exceptional accuracy, others, such as Orchard, require further refinement in categorization methods. Overall, the study provides valuable insights into using multi-sensor remote sensing data for agricultural monitoring and decision-making.
References
Asia and the Pacific Regional Overview of Food Security and Nutrition 2022. FAO, 2023. doi: 10.4060/cc3990en.
G. A. Abubakar et al., “Mapping Maize Cropland and Land Cover in Semi-Arid Region in Northern Nigeria Using Machine Learning and Google Earth Engine,” Remote Sens., vol. 15, no. 11, p. 2835, May 2023, doi: 10.3390/rs15112835.
M. Majeed et al., “Prediction of flash flood susceptibility using integrating analytic hierarchy process (AHP) and frequency ratio (FR) algorithms,” Front. Environ. Sci., vol. 10, no. January, pp. 1–14, 2023, doi: 10.3389/fenvs.2022.1037547.
J. Wang et al., “Mapping sugarcane plantation dynamics in Guangxi, China, by time series Sentinel-1, Sentinel-2 and Landsat images,” Remote Sens. Environ., vol. 247, p. 111951, Sep. 2020, doi: 10.1016/j.rse.2020.111951.
V. Tiwari et al., “Wheat Area Mapping in Afghanistan Based on Optical and SAR Time-Series Images in Google Earth Engine Cloud Environment,” Front. Environ. Sci., vol. 8, Jun. 2020, doi: 10.3389/fenvs.2020.00077.
Y. Durgun, A. Gobin, R. Van De Kerchove, and B. Tychon, “Crop Area Mapping Using 100-m Proba-V Time Series,” Remote Sens., vol. 8, no. 7, p. 585, Jul. 2016, doi: 10.3390/rs8070585.
A. Elders et al., “Estimating crop type and yield of small holder fields in Burkina Faso using multi-day Sentinel-2,” Remote Sens. Appl. Soc. Environ., vol. 27, p. 100820, Aug. 2022, doi: 10.1016/j.rsase.2022.100820.
K. Van Tricht, A. Gobin, S. Gilliams, and I. Piccard, “Synergistic Use of Radar Sentinel-1 and Optical Sentinel-2 Imagery for Crop Mapping: A Case Study for Belgium,” Remote Sens., vol. 10, no. 10, p. 1642, Oct. 2018, doi: 10.3390/rs10101642.
Y. Pageot, F. Baup, J. Inglada, N. Baghdadi, and V. Demarez, “Detection of Irrigated and Rainfed Crops in Temperate Areas Using Sentinel-1 and Sentinel-2 Time Series,” Remote Sens., vol. 12, no. 18, p. 3044, Sep. 2020, doi: 10.3390/rs12183044.
G. R. Aduvukha et al., “Cropping Pattern Mapping in an Agro-Natural Heterogeneous Landscape Using Sentinel-2 and Sentinel-1 Satellite Datasets,” Agriculture, vol. 11, no. 6, p. 530, Jun. 2021, doi: 10.3390/agriculture11060530.
S. Felegari et al., “Integration of Sentinel 1 and Sentinel 2 Satellite Images for Crop Mapping,” Appl. Sci., vol. 11, no. 21, p. 10104, Oct. 2021, doi: 10.3390/app112110104.
C. Li et al., “Mapping Winter Wheat with Optical and SAR Images Based on Google Earth Engine in Henan Province, China,” Remote Sens., vol. 14, no. 2, Jan. 2022, doi: 10.3390/rs14020284.
S. Asam, U. Gessner, R. Almengor González, M. Wenzl, J. Kriese, and C. Kuenzer, “Mapping Crop Types of Germany by Combining Temporal Statistical Metrics of Sentinel-1 and Sentinel-2 Time Series with LPIS Data,” Remote Sens., vol. 14, no. 13, p. 2981, Jun. 2022, doi: 10.3390/rs14132981.
N. Gorelick, M. Hancher, M. Dixon, S. Ilyushchenko, D. Thau, and R. Moore, “Google Earth Engine: Planetary-scale geospatial analysis for everyone,” Remote Sens. Environ., vol. 202, pp. 18–27, Dec. 2017, doi: 10.1016/j.rse.2017.06.031.
W. Zhang, M. Brandt, A. V. Prishchepov, Z. Li, C. Lyu, and R. Fensholt, “Mapping the Dynamics of Winter Wheat in the North China Plain from Dense Landsat Time Series (1999 to 2019),” Remote Sens., vol. 13, no. 6, p. 1170, Mar. 2021, doi: 10.3390/rs13061170.
A. Huete, K. Didan, T. Miura, E. . Rodriguez, X. Gao, and L. . Ferreira, “(NDVI EVI)Overview of the radiometric and biophysical performance of the MODIS vegetation indices,” Remote Sens. Environ., vol. 83, no. 1–2, pp. 195–213, Nov. 2002, doi: 10.1016/S0034-4257(02)00096-2.
A. . Huete, “A soil-adjusted vegetation index (SAVI),” Remote Sens. Environ., vol. 25, no. 3, pp. 295–309, Aug. 1988, doi: 10.1016/0034-4257(88)90106-X.
N. Mzid, S. Pignatti, W. Huang, and R. Casa, “An Analysis of Bare Soil Occurrence in Arable Croplands for Remote Sensing Topsoil Applications,” Remote Sens., vol. 13, no. 3, p. 474, Jan. 2021, doi: 10.3390/rs13030474.
Y. He, E. Lee, and T. A. Warner, “A time series of annual land use and land cover maps of China from 1982 to 2013 generated using AVHRR GIMMS NDVI3g data,” Remote Sens. Environ., vol. 199, pp. 201–217, Sep. 2017, doi: 10.1016/j.rse.2017.07.010.
V. F. Rodriguez-Galiano, B. Ghimire, J. Rogan, M. Chica-Olmo, and J. P. Rigol-Sanchez, “An assessment of the effectiveness of a random forest classifier for land-cover classification,” ISPRS J. Photogramm. Remote Sens., vol. 67, pp. 93–104, Jan. 2012, doi: 10.1016/j.isprsjprs.2011.11.002.
L. Breiman, “machine Learning,” Mach. Learn., vol. 45, no. 1, pp. 5–32, 2001, doi: 10.1023/A:1010933404324.
A. F. Koko, Y. Wu, G. A. Abubakar, A. A. N. Alabsi, R. Hamed, and M. Bello, “Thirty Years of Land Use/Land Cover Changes and Their Impact on Urban Climate: A Study of Kano Metropolis, Nigeria,” Land, vol. 10, no. 11, p. 1106, Oct. 2021, doi: 10.3390/land10111106.
A. Tassi and M. Vizzari, “Object-Oriented LULC Classification in Google Earth Engine Combining SNIC, GLCM, and Machine Learning Algorithms,” Remote Sens., vol. 12, no. 22, p. 3776, Nov. 2020, doi: 10.3390/rs12223776.
D. Chicco and G. Jurman, “The advantages of the Matthews correlation coefficient (MCC) over F1 score and accuracy in binary classification evaluation,” BMC Genomics, vol. 21, no. 1, p. 6, Dec. 2020, doi: 10.1186/s12864-019-6413-7.
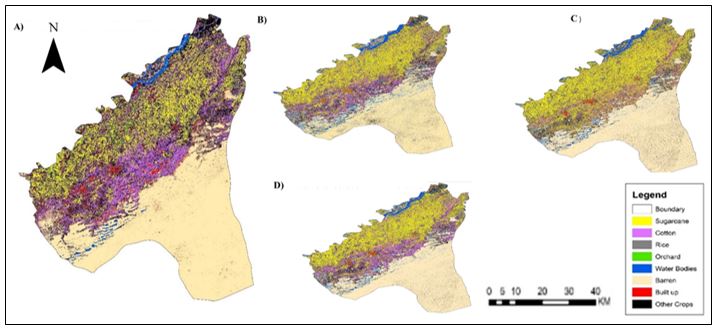
Downloads
Published
How to Cite
Issue
Section
License
Copyright (c) 2024 50SEA

This work is licensed under a Creative Commons Attribution 4.0 International License.