Evaluating the Effectiveness of Phase Difference in Early Drought Detection
Keywords:
Lag Time, NDVI, Rainfall, Drought, Thar dessertAbstract
Introduction. This research work focuses on how various phase relationships can enhance our understanding of the effects of drought on moisture deficiency in desert ecosystems, an extensive and damaging environmental phenomenon that affects natural ecosystems, economies, health, agriculture, and society.
Novelty Statement. The primary objective of this research is to inspect the lag time variance between fixed and dynamic lag windows correlated with NDVI, aiming to devise an optimal methodology for drought analysis in this region.
Material and Methods. Leveraging remote sensing data, this study delves into the complex drought dynamics of the Thar Desert, employing a comprehensive analysis of 22 years of CHIRPS rainfall time series data and MODIS NDVI (Normalized Difference Vegetation Index) product. This study performed a cross-correlation of rainfall and NDVI, comparing the lag time difference between fixed lag windows (16, 32, 48, 64 days) and dynamic lag windows (ranging from 4 to 64 days with incremental steps) against 22 years of NDVI data of MODIS.
Results and Discussions. The preliminary results showed that dynamic lag windows of 4, 8, 12, 16, …, and 64 days exhibit the highest correlation with NDVI, with a lag time of 40 days showing maximum correlation. These findings suggest that dynamic lag windows capture the temporal variability of drought impact on vegetation more effectively compared to fixed lag windows in the Thar Desert. The same work was done with a sub-dynamic lag window ranging in between the highly correlated lag episodes of dynamic and fix windows respectively i.e.,40 days and 48 days, concluding that a lag phase of 42 days exhibits the highest correlation with vegetation more effectively. Furthermore, the study unveils a significant drought event in 2002, showcasing the sensitivity of the dynamic lag approach in detecting extreme drought occurrences.
Concluding Remarks. This research not only advances drought analysis methodologies in arid regions but also underscores the imperative for future investigations to explore the generalizability of dynamic lag windows across diverse regions and evaluate their predictive capacity in forecasting drought-induced vegetation changes.
References
A. Hussain et al., “Analyzing the impact of drought on agriculture: evidence from Pakistan using standardized precipitation evapotranspiration index,” Natural Hazards, vol. 115, no. 1, pp. 389–408, Jan. 2023, doi: 10.1007/s11069-022-05559-6.
J. E. Nichol and S. Abbas, “Integration of remote sensing datasets for local scale assessment and prediction of drought,” Science of the Total Environment, vol. 505, pp. 503–507, Feb. 2015, doi: 10.1016/j.scitotenv.2014.09.099.
M. Kazemzadeh et al., “Detecting drought events over Iran during 1983–2017 using satellite and ground-based precipitation observations,” Atmos Res, vol. 269, May 2022, doi: 10.1016/j.atmosres.2022.106052.
H. West, N. Quinn, and M. Horswell, “Remote Sensing for Drought Monitoring & Impact Assessment: Progress, Past Challenges and Future Opportunities 2 3.”
A. AghaKouchak et al., “Remote sensing of drought: Progress, challenges and opportunities,” Reviews of Geophysics, vol. 53, no. 2. Blackwell Publishing Ltd, pp. 452–480, Jun. 01, 2015. doi: 10.1002/2014RG000456.
Q. Zhu, Y. Luo, D. Zhou, Y. P. Xu, G. Wang, and H. Gao, “Drought monitoring utility using satellite-based precipitation products over the Xiang River Basin in China,” Remote Sens (Basel), vol. 11, no. 12, Jun. 2019, doi: 10.3390/rs11121483.
Q. Qin et al., “Optical and thermal remote sensing for monitoring agricultural drought,” Remote Sensing, vol. 13, no. 24. MDPI, Dec. 01, 2021. doi: 10.3390/rs13245092.
M. Ding, Y. Zhang, L. Liu, W. Zhang, Z. Wang, and W. Bai, “The relationship between NDVI and precipitation on the Tibetan Plateau,” Journal of Geographical Sciences, vol. 17, no. 3, pp. 259–268, Jul. 2007, doi: 10.1007/s11442-007-0259-7.
S. Abbas, J. E. Nichol, F. M. Qamer, and J. Xu, “Characterization of Drought Development through Remote Sensing: A Case Study in Central Yunnan,” vol. 6, pp. 4998–5018, 2014, [Online]. Available: www.mdpi.com/journal/remotesensing
M. Van Hoek, L. Jia, J. Zhou, C. Zheng, and M. Menenti, “Early drought detection by spectral analysis of satellite time series of precipitation and Normalized Difference Vegetation Index (NDVI),” Remote Sens (Basel), vol. 8, no. 5, 2016, doi: 10.3390/rs8050422.
T. E. of E. Britannica, “Thar Desert,” Britannica.
O. U. . PRISM Climate Group: Corvallis, “PRISM Climate Group. PRISM Gridded Climate Data; .”
N. Gorelick, M. Hancher, M. Dixon, S. Ilyushchenko, D. Thau, and R. Moore, “Google Earth Engine: Planetary-scale geospatial analysis for everyone,” Remote Sens Environ, vol. 202, pp. 18–27, Dec. 2017, doi: 10.1016/j.rse.2017.06.031.
C. Funk et al., “The climate hazards infrared precipitation with stations - A new environmental record for monitoring extremes,” Sci Data, vol. 2, Dec. 2015, doi: 10.1038/sdata.2015.66.
J. Chen, P. Jönsson, M. Tamura, Z. Gu, B. Matsushita, and L. Eklundh, “A simple method for reconstructing a high-quality NDVI time-series data set based on the Savitzky–Golay filter,” Remote Sens Environ, vol. 91, no. 3–4, pp. 332–344, Jun. 2004, doi: 10.1016/J.RSE.2004.03.014.
P. Khatri-Chhetri, S. M. Hendryx, K. A. Hartfield, M. A. Crimmins, W. J. D. van Leeuwen, and V. R. Kane, “Assessing vegetation response to multi-scalar drought across the mojave, sonoran, chihuahuan deserts and apache highlands in the Southwest United States,” Remote Sens (Basel), vol. 13, no. 6, Mar. 2021, doi: 10.3390/rs13061103.
X. jian Lu, Z. bao Li, H. bo Yan, and Y. ji Liang, “Spatiotemporal variations of drought and driving factors based on multiple remote sensing drought indices: A case study in karst areas of southwest China,” J Mt Sci, vol. 20, no. 11, pp. 3215–3232, Nov. 2023, doi: 10.1007/s11629-023-7927-7.
M. Bilal, M. U. Liaqat, M. J. M. Cheema, T. Mahmood, and Q. Khan, “Spatial Drought Monitoring in Thar Desert Using Satellite-Based Drought Indices and Geo-Informatics Techniques,” MDPI AG, Nov. 2017, p. 179. doi: 10.3390/ecws-2-04948.
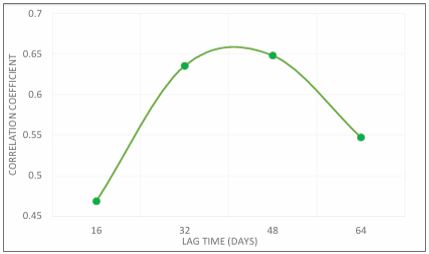
Downloads
Published
How to Cite
Issue
Section
License
Copyright (c) 2024 50SEA

This work is licensed under a Creative Commons Attribution 4.0 International License.