Significance of Education Data Mining in Student’s Academic Performance Prediction and Analysis
Keywords:
Machine Learning, Support Vector Machine, Decision Support, Data MiningAbstract
Data Mining (DM) is relevant to extract the hidden patterns from the voluminous amount of the data. Applying DM, in education is an evolving interdisciplinary research domain, which is also called as educational data mining (EDM). At present, student data about their academics is available to identify important hidden trends to be explored for enhancing student academic performance. In higher education, forecasting student success is essential for helping with course selection and creating individualized study schedules. It helps instructors and managers keep tabs on students, ensure their development, and modify training programs for the best results. Growth and development of any nation depend on educational institutions since they are fundamental social foundations. It is now feasible to use past data for effective learning and prediction of future behavior in a variety of troublesome areas thanks to the development of DM as a potent approach. Educational institutions may make wise judgments and promote improvements in the education sector by utilizing the possibilities of DM supported EDM approaches. It is feasible to pinpoint improvement areas and direct upcoming skill development by examining pupils' performance on various academic evaluations. Furthermore, this procedure lessens the frequency of official warnings and ineffective student expulsions, fostering a more encouraging and fruitful learning atmosphere. In this work, a unique algorithm that combines classification and clustering approaches to predict students' academic success has been suggested. Real-time student datasets from several academic institutes in higher education were used to test the suggested approach. The findings show that the suggested model worked well for predicting students' academic achievement.
References
S. M. Dol and P. M. Jawandhiya, “Classification Technique and its Combination with Clustering and Association Rule Mining in Educational Data Mining — A survey,” Eng. Appl. Artif. Intell., vol. 122, p. 106071, Jun. 2023, doi: 10.1016/J.ENGAPPAI.2023.106071.
A. Sánchez, C. Vidal-Silva, G. Mancilla, M. Tupac-Yupanqui, and J. M. Rubio, “Sustainable e-Learning by Data Mining—Successful Results in a Chilean University,” Sustain., vol. 15, no. 2, pp. 1–16, 2023, doi: 10.3390/su15020895.
L. H. AL-Mashanji, A. K., Hamza, A. H., & Alhasnawy, “Computational Prediction Algorithms and Tools Used in Educational Data Mining: A Review.",” J. Univ. Babylon Pure Appl. Sci., pp. 87-99., 2023.
A. C. Doctor, “‘A Predictive Model using Machine Learning Algorithm in Identifying Students Probability on Passing Semestral Course.’ arXiv preprint arXiv:2304.05565,” 2023.
C. Jalota, “An Effectual Model for Early Prediction of Academic Perfomance using Ensemble Classification,” J. Lang. Linguist. Soc., no. 32, pp. 19–33, 2023, doi: 10.55529/jlls.32.19.33.
Y. Qian, C. X. Li, X. G. Zou, X. Bin Feng, M. H. Xiao, and Y. Q. Ding, “Research on predicting learning achievement in a flipped classroom based on MOOCs by big data analysis,” Comput. Appl. Eng. Educ., vol. 30, no. 1, pp. 222–234, 2022, doi: 10.1002/cae.22452.
K. V. Deshpande, S. Asbe, A. Lugade, Y. More, D. Bhalerao, and A. Partudkar, “Learning Analytics Powered Teacher Facing Dashboard to Visualize, Analyze Students’ Academic Performance and give Key DL(Deep Learning) Supported Key Recommendations for Performance Improvement.,” 2023 Int. Conf. Adv. Technol. ICONAT 2023, 2023, doi: 10.1109/ICONAT57137.2023.10080832.
S. Göktepe Körpeoğlu and S. Göktepe Yıldız, “Comparative analysis of algorithms with data mining methods for examining attitudes towards STEM fields,” Educ. Inf. Technol., vol. 28, no. 3, pp. 2791–2826, Mar. 2023, doi: 10.1007/S10639-022-11216-Z/METRICS.
S. M. Pande, “Machine Learning Models for Student Performance Prediction,” Int. Conf. Innov. Data Commun. Technol. Appl. ICIDCA 2023 - Proc., pp. 27–32, 2023, doi: 10.1109/ICIDCA56705.2023.10099503.
M. Zhang, J. Fan, A. Sharma, and A. Kukkar, “Data mining applications in university information management system development,” J. Intell. Syst., vol. 31, no. 1, pp. 207–220, 2022, doi: 10.1515/jisys-2022-0006.
X. Wang, Y. Zhao, C. Li, and P. Ren, “ProbSAP: A comprehensive and high-performance system for student academic performance prediction,” Pattern Recognit., vol. 137, p. 109309, May 2023, doi: 10.1016/J.PATCOG.2023.109309.
L. Yan, “Application of Data Mining in Psychological Education of College Students in Private Independent Colleges,” Lect. Notes Inst. Comput. Sci. Soc. Telecommun. Eng. LNICST, vol. 465 LNICST, pp. 206–215, 2023, doi: 10.1007/978-3-031-23950-2_23/COVER.
R. A. Kale and M. K. Rawat, “Improvement in student performance using 4QS and machine learning approach,” Recent Adv. Mater. Manuf. Mach. Learn., pp. 440–451, May 2023, doi: 10.1201/9781003358596-48.
M. Maphosa, W. Doorsamy, and B. S. Paul, “Student Performance Patterns in Engineering at the University of Johannesburg: An Exploratory Data Analysis,” IEEE Access, vol. 11, pp. 48977–48987, 2023, doi: 10.1109/ACCESS.2023.3277225.
V. S. Verykios, R. Tsoni, G. Garani, and C. T. Panagiotakopoulos, “Fleshing Out Learning Analytics and Educational Data Mining with Data and ML Pipelines,” Intell. Syst. Ref. Libr., vol. 236, pp. 155–173, 2023, doi: 10.1007/978-3-031-22371-6_8/COVER.
S. Dhara, S. Chatterjee, R. Chaudhuri, A. Goswami, and S. K. Ghosh, “Artificial Intelligence in Assessment of Students’ Performance,” Artif. Intell. High. Educ., pp. 153–167, Aug. 2022, doi: 10.1201/9781003184157-8.
K. Shilpa and T. Adilakshmi, “Analysis of SWCET Student’s Results Using Educational Data Mining Techniques,” Cogn. Sci. Technol., pp. 639–651, 2023, doi: 10.1007/978-981-19-2358-6_58/COVER.
A. Kukkar, R. Mohana, A. Sharma, and A. Nayyar, “Prediction of student academic performance based on their emotional wellbeing and interaction on various e-learning platforms,” Educ. Inf. Technol., vol. 28, no. 8, pp. 9655–9684, Aug. 2023, doi: 10.1007/S10639-022-11573-9/METRICS.
G. Lampropoulos, “‘Educational Data Mining and Learning Analytics in the 21st Century.,’” Encycl. Data Sci. Mach. Learn. IGI Glob., pp. 1642–1651, 2023.
K. Mahboob, R. Asif, and N. G. Haider, “Quality enhancement at higher education institutions by early identifying students at risk using data mining,” Mehran Univ. Res. J. Eng. Technol., vol. 42, no. 1, p. 120, 2023, doi: 10.22581/muet1982.2301.12.
S. Batool, J. Rashid, M. W. Nisar, J. Kim, H. Y. Kwon, and A. Hussain, “Educational data mining to predict students’ academic performance: A survey study,” Educ. Inf. Technol. 2022 281, vol. 28, no. 1, pp. 905–971, Jul. 2022, doi: 10.1007/S10639-022-11152-Y.
A. S. Abdelmagid and A. I. M. Qahmash, “Utilizing the Educational Data Mining Techniques ‘Orange Technology’ for Detecting Patterns and Predicting Academic Performance of University Students,” Inf. Sci. Lett., vol. 12, no. 3, pp. 1415–1431, Mar. 2023, doi: 10.18576/ISL/120330.
V. M. Yonder and G. Elbiz Arslan, “PREDICTING STUDENTS’ ACADEMIC SUCCESS IN HYBRID DESIGN STUDIOS WITH GENERALIZED REGRESSION NEURAL NETWORKS (GRNN),” INTED2023 Proc., vol. 1, pp. 6961–6967, Mar. 2023, doi: 10.21125/INTED.2023.1890.
Y. B. Ampadu, “Handling Big Data in Education: A Review of Educational Data Mining Techniques for Specific Educational Problems,” AI, Comput. Sci. Robot. Technol., vol. 2, no. April, pp. 1–16, 2023, doi: 10.5772/acrt.17.
S. C. Mwape and D. Kunda, “Using data mining techniques to predict university student’s ability to graduate on schedule,” Int. J. Innov. Educ., vol. 8, no. 1, p. 40, 2023, doi: 10.1504/IJIIE.2023.128470.
T. Guarda, O. Barrionuevo, and J. A. Victor, “Higher Education Students Dropout Prediction,” Smart Innov. Syst. Technol., vol. 328, pp. 121–128, 2023, doi: 10.1007/978-981-19-7689-6_11/COVER.
M. D. Adane, J. K. Deku, and E. K. Asare, “Performance Analysis of Machine Learning Algorithms in Prediction of Student Academic Performance,” J. Adv. Math. Comput. Sci., vol. 38, no. 5, pp. 74–86, 2023, doi: 10.9734/jamcs/2023/v38i51762.
R. Trakunphutthirak and V. C. S. Lee, “Application of Educational Data Mining Approach for Student Academic Performance Prediction Using Progressive Temporal Data,” https://doi.org/10.1177/07356331211048777, vol. 60, no. 3, pp. 742–776, Sep. 2021, doi: 10.1177/07356331211048777.
H. Li, “Application of Classification Mining Technology Based on Decision Tree in Student Resource Management,” Lect. Notes Inst. Comput. Sci. Soc. Telecommun. Eng. LNICST, vol. 465 LNICST, pp. 149–160, 2023, doi: 10.1007/978-3-031-23950-2_17/COVER.
A. Xiu, “In Depth Mining Method of Online Higher Education Resources Based on K-Means Clustering,” Lect. Notes Inst. Comput. Sci. Soc. Telecommun. Eng. LNICST, vol. 454 LNICST, pp. 31–43, 2022, doi: 10.1007/978-3-031-21164-5_3/COVER.
G. Ramaswami, T. Susnjak, and A. Mathrani, “On Developing Generic Models for Predicting Student Outcomes in Educational Data Mining,” Big Data Cogn. Comput., vol. 6, no. 1, 2022, doi: 10.3390/bdcc6010006.
M. H. Bin Roslan and C. J. Chen, “Predicting students’ performance in English and Mathematics using data mining techniques,” Educ. Inf. Technol., vol. 28, no. 2, pp. 1427–1453, 2023, doi: 10.1007/s10639-022-11259-2.
A. D. Ali and W. K. Hanna, “Predicting Students’ Achievement in a Hybrid Environment Through Self-Regulated Learning, Log Data, and Course Engagement: A Data Mining Approach,” https://doi.org/10.1177/07356331211056178, vol. 60, no. 4, pp. 960–985, Dec. 2021, doi: 10.1177/07356331211056178.
I. A. Najm et al., “OLAP Mining with Educational Data Mart to Predict Students’ Performance,” Inform., vol. 46, no. 5, pp. 11–19, 2022, doi: 10.31449/inf.v46i5.3853.
S. U. Asad, R., Arooj, S., & Rehman, “‘Study of Educational Data Mining Approaches for Student Performance Analysis.,’” Tech. J., vol. 27, no. 1, pp. 68-81., 2022.
F. Inusah, Y. M. Missah, U. Najim, and F. Twum, “Data Mining and Visualisation of Basic Educational Resources for Quality Education,” Int. J. Eng. Trends Technol., vol. 70, no. 12, pp. 296–307, 2022, doi: 10.14445/22315381/IJETT-V70I12P228.
Z. ul Abideen et al., “Analysis of Enrollment Criteria in Secondary Schools Using Machine Learning and Data Mining Approach,” Electron., vol. 12, no. 3, 2023, doi: 10.3390/electronics12030694.
E. Araka, R. Oboko, E. Maina, and R. Gitonga, “Using Educational Data Mining Techniques to Identify Profiles in Self-Regulated Learning: An Empirical Evaluation,” Int. Rev. Res. Open Distance Learn., vol. 23, no. 1, pp. 131–162, 2022, doi: 10.19173/IRRODL.V22I4.5401.
S. Ahmad, M. A. El-Affendi, M. S. Anwar, and R. Iqbal, “Potential Future Directions in Optimization of Students’ Performance Prediction System,” Comput. Intell. Neurosci., vol. 2022, 2022, doi: 10.1155/2022/6864955.
A. Sánchez, C. Vidal-Silva, G. Mancilla, M. Tupac-Yupanqui, and J. M. Rubio, “Sustainable e-Learning by Data Mining—Successful Results in a Chilean University,” Sustain. 2023, Vol. 15, Page 895, vol. 15, no. 2, p. 895, Jan. 2023, doi: 10.3390/SU15020895.
M. Rahman, M. Hasan, Md Masum Billah, and Rukaiya Jahan Sajuti, “Grading System Prediction of Educational Performance Analysis Using Data Mining Approach,” Malaysian J. Sci. Adv. Technol., vol. 2, no. 4, pp. 204–211, 2022, doi: 10.56532/mjsat.v2i4.96.
S. Arulselvarani, “E-learning and Data Mining using Machine Learning Algorithms,” 8th Int. Conf. Adv. Comput. Commun. Syst. ICACCS 2022, pp. 218–222, 2022, doi: 10.1109/ICACCS54159.2022.9785186.
I. N. M. Al Alawi, S. J. S., Jamil, J. M., & Shaharanee, “‘Predicting Student Performance Using Data Mining Approach: A Case Study in Oman.,’” Math. Stat. Eng. Appl., vol. 71, no. 4, pp. 1389–139, 2022.
Nidhi, M. Kumar, D. Handa, and S. Agarwal, “Student’s Academic Performance Prediction by Using Ensemble Techniques,” AIP Conf. Proc., vol. 2555, no. 1, Oct. 2022, doi: 10.1063/5.0124636/2829661.
D. A. Shafiq, M. Marjani, R. A. A. Habeeb, and D. Asirvatham, “Student Retention Using Educational Data Mining and Predictive Analytics: A Systematic Literature Review,” IEEE Access, vol. 10, no. July, pp. 72480–72503, 2022, doi: 10.1109/ACCESS.2022.3188767.
“S. S. Chetna, ‘Opportunities and Challenges for Educational Data Mining.,’” Math. Stat. Eng. Appl., vol. 71, no. 4, pp. 6176–6188, 2022.
G. Feng, M. Fan, and Y. Chen, “Analysis and Prediction of Students’ Academic Performance Based on Educational Data Mining,” IEEE Access, vol. 10, pp. 19558–19571, 2022, doi: 10.1109/ACCESS.2022.3151652.
C. Liu, H. Wang, Y. Du, and Z. Yuan, “A Predictive Model for Student Achievement Using Spiking Neural Networks Based on Educational Data,” Appl. Sci., vol. 12, no. 8, 2022, doi: 10.3390/app12083841.
G. Novillo Rangone, C. Pizarro, and G. Montejano, “Automation of an Educational Data Mining Model Applying Interpretable Machine Learning and Auto Machine Learning,” Smart Innov. Syst. Technol., vol. 259 SIST, pp. 22–30, 2022, doi: 10.1007/978-981-16-5792-4_3/COVER.
& E. A. B. Y. Y. Dyulicheva, “‘Learning analytics of MOOCs based on natural language processing.,’” CEUR Work. Proc., pp. 187–197, 2022.
S. Garmpis, M. Maragoudakis, and A. Garmpis, “Assisting Educational Analytics with AutoML Functionalities,” Computers, vol. 11, no. 6, 2022, doi: 10.3390/computers11060097.
K. Okoye, A. Arrona-Palacios, C. Camacho-Zuñiga, J. A. G. Achem, J. Escamilla, and S. Hosseini, Towards teaching analytics: a contextual model for analysis of students’ evaluation of teaching through text mining and machine learning classification, vol. 27, no. 3. 2022. doi: 10.1007/s10639-021-10751-5.
L. Zárate, M. W. Rodrigues, S. M. Dias, C. Nobre, and M. Song, “SciBR-M: a method to map the evolution of scientific interest - A case study in educational data mining,” Libr. Hi Tech, vol. ahead-of-p, no. ahead-of-print, Jan. 2023, doi: 10.1108/LHT-04-2022-0222.
S. Caspari-Sadeghi, “Learning assessment in the age of big data: Learning analytics in higher education,” Cogent Educ., vol. 10, no. 1, 2023, doi: 10.1080/2331186X.2022.2162697.
S. Boumi., “‘Development of a Multivariate Poisson Hidden Markov Model for Application in Educational Data Mining.’”.
J. A. Gómez-Pulido, Y. Park, R. Soto, and J. M. Lanza-Gutiérrez, “Data Analytics and Machine Learning in Education,” Appl. Sci., vol. 13, no. 3, pp. 13–15, 2023, doi: 10.3390/app13031418.
N. Nithiyanandam et al., “Artificial Intelligence Assisted Student Learning and Performance Analysis using Instructor Evaluation Model,” 3rd Int. Conf. Electron. Sustain. Commun. Syst. ICESC 2022 - Proc., pp. 1555–1561, 2022, doi: 10.1109/ICESC54411.2022.9885462.
S. N. Safitri, Haryono Setiadi, and E. Suryani, “Educational Data Mining Using Cluster Analysis Methods and Decision Trees based on Log Mining,” J. RESTI (Rekayasa Sist. dan Teknol. Informasi), vol. 6, no. 3, pp. 448–456, 2022, doi: 10.29207/resti.v6i3.3935.
M. Sudais, M. Safwan, and S. Ahmed, “Students ’ Academic Performance Prediction Model Using Machine Learning,” Res. Sq., 2022.
P. Houngue, M. Hountondji, and T. Dagba, “An Effective Decision-Making Support for Student Academic Path Selection using Machine Learning,” Int. J. Adv. Comput. Sci. Appl., vol. 13, no. 11, pp. 727–734, 2022, doi: 10.14569/IJACSA.2022.0131184.
F. Buckland, M., and Gey, “‘The relationship between recall and precision.,’” J. Am. Soc. Inf. Sci., vol. 45, no. 1, pp. 12–19, 1994.
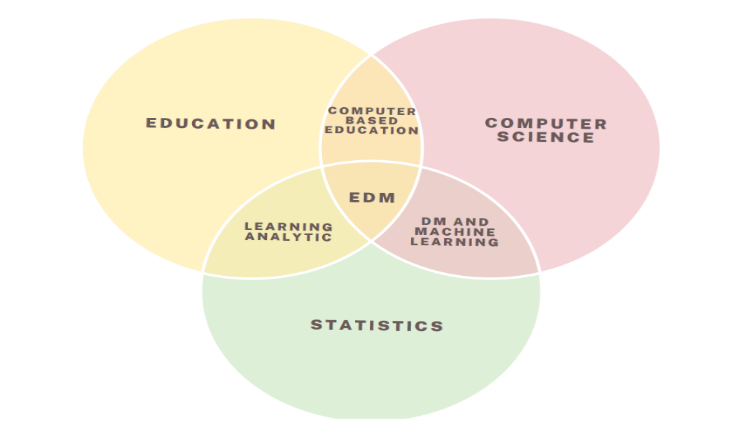
Published
How to Cite
Issue
Section
License
Copyright (c) 2023 50SEA

This work is licensed under a Creative Commons Attribution 4.0 International License.